Open Access
CORRECTION
Correction: Breast Calcifications and Histopathological Analysis on Tumour Detection by CNN
1
Department of Computer Science and Engineering, Paavai Engineering College, Pachal, Namakkal, 637018, Tamil Nadu, India
2
Al-Nahrain University, Al-Nahrain Nanorenewable Energy Research Center, Baghdad, Iraq
3
COMBA R&D Laboratory, Faculty of Engineering, Universidad Santiago de Cali, 76001, Cali, Colombia
4
Department of Computer Science and Engineering, Kongu Engineering College, Perundurai, 638060, Tamil Nadu, India
5
Department of Mathematics, Jaypee University of Engineering and Technology, Guna, 473226, Madhya Pradesh, India
*
Corresponding Author: D. Banumathy. Email: baanumathyd@gmail.com
Computer Systems Science and Engineering 2024, 48(3), 863-866. https://doi.org/10.32604/csse.2024.053657
Issue published 20 May 2024
This article is a correction of:
Breast Calcifications and Histopathological Analysis on Tumour Detection by CNN
Read original article
Abstract
This article has no abstract.In the article “Breast Calcifications and Histopathological Analysis on Tumour Detection by CNN” by D. Banumathy, Osamah Ibrahim Khalaf, Carlos Andrés Tavera Romero, P. Vishnu Raja and Dilip Kumar Sharma (Computer Systems Science and Engineering, 2023, Vol. 44, No. 1, pp. 595–612. DOI: 10.32604/csse.2023.025611), some references were wrongly cited.
The authors wish to apologize for any inconvenience caused by this error. Please check the following updates:
Original Content/Reference:
1. Delete References [7], [22], [23], [25–28], [31–39], [42–47], [49], [51], [55–68], [70]
7. A. V. Wedelstedt, S. Arnrich, T. Haehnel and G. Kalies, “A cut-off criterion for calculating more reliable adsorption energy distributions from gas adsorption isotherms,” Adsorption Science & Technology, vol. 36, no. 9, pp. 1629–1647, 2018.
22. R. Vasanthi, O. I. Khalaf, C. A. T. Romero, S. Sudhakar and D. K. Sharma, “Interactive middleware services for heterogeneous systems,” Computer Systems Science and Engineering, vol. 41, no. 3, pp. 1241–1253, 2022.
23. A. Mehbodniya, S. Bhatia, A. Mashat, E. Mohanraj and S. Sudhakar, “Proportional fairness based energy- efficient routing in wireless sensor network,” Computer Systems Science and Engineering, vol. 41, no. 3, pp. 1071–1082, 2022.
25. D. Stalin David, M. Anam, K. Chandraprabha, S. Arun Mozhi Selvi, D. K. Sharma et al., “Cloud security service for identifying unauthorized user behaviour,” Computers, Materials & Continua, vol. 70, no. 2, pp. 2581–2600, 2022.
26. K. Rajakumari, M. Vinoth Kumar, G. Verma, S. Balu, D. K. Sharma et al., “Fuzzy based ant colony optimization scheduling in cloud computing,” Computer Systems Science and Engineering, vol. 40, no. 2, pp. 581–592, 2022.
27. R. Nithya, K. Amudha, A. Syed Musthafa, D. K. Sharma, E. H. R. Asis et al., “An optimized fuzzy-based ant colony algorithm for 5G-MANET,” Computers, Materials & Continua, vol. 70, no. 1, pp. 1069–1087, 2022.
28. M. Rajalakshmi, V. Saravanan, V. Arunprasad, C. A. T. Romero, O. I. Khalaf et al., “Machine learning for modeling and control of industrial clarifier process,” Intelligent Automation & Soft Computing, vol. 32, no. 1, pp. 339–359, 2022.
31. A. D. Salman, O. I. Khalaf and G. M. Abdulsahib, “An adaptive intelligent alarm system for wireless sensor network,” Indonesian Journal of Electrical Engineering and Computer Science, vol. 15, no. 1, pp. 142–147, 2019.
32. K. A. Ogudo, D. M. J. Nestor, O. I. Khalaf and H. D. Kasmaei, “A device performance and data analytics concept for smartphones’ IoT services and machine-type communication in cellular networks,” Symmetry, vol. 11, no. 4, pp. 593–609, 2019.
33. O. I. Khalaf and G. M. Abdulsahib, “Frequency estimation by the method of minimum mean squared error and P- value distributed in the wireless sensor network,” Journal of Information Science and Engineering, vol. 35, no. 5, pp. 1099–1112, 2019.
34. O. I. Khalaf and B. M. Sabbar, “An overview on wireless sensor networks and finding optimal location of nodes,” Periodicals of Engineering and Natural Sciences, vol. 7, no. 3, pp. 1096–1101, 2019.
35. G. M. Abdulsahib and O. I. Khalaf, “An improved algorithm to fire detection in forest by using wireless sensor networks,” International Journal of Civil Engineering and Technology (IJCIET), vol. 9, no. 11, pp. 369–377, 2018.
36. O. I. Khalaf and G. M. Abdulsahib, “Energy efficient routing and reliable data transmission protocol in WSN,” International Journal of Advances in Soft Computing and Its Application, vol. 12, no. 3, pp. 45–53, 2020.
37. O. I. Khalaf, G. M. Abdulsahib and B. M. Sabbar, “Optimization of wireless sensor network coverage using the bee algorithm,” Journal of Information Science and Engineering, vol. 36, no. 2, pp. 377–386, 2020.
38. G. M. Abdulsahib and O. I. Khalaf, “Accurate and effective data collection with minimum energy path selection in wireless sensor networks using mobile sinks,” Journal of Information Technology Management, vol. 13, no. 2, pp. 139–153, 2021.
39. O. I. Khalaf and G. M. Abdulsahib, “Optimized dynamic storage of data (ODSD) in IoT based on blockchain for wireless sensor networks,” Peer-to-Peer Networking and Applications, vol. 14, no. 5, pp. 2858–2873, 2021.
42. O. I. Khalaf, M. Sokiyna, Y. Alotaibi, A. Alsufyani and S. Alghamdi, “Web attack detection using the input validation method: DPDA theory,” Computers, Materials & Continua, vol. 68, no. 3, pp. 3167–3184, 2021.
43. S. Palanisamy, B. Thangaraju, O. I. Khalaf, Y. Alotaibi, S. Alghamdi et al., “A novel approach of design and analysis of a hexagonal fractal antenna array (HFAA) for next-generation wireless communication,” Energies, vol. 14, no. 6204, pp. 1–18, 2021.
44. S. N. Alsubari1, S. N. Deshmukh, A. A. Alqarni, N. Alsharif, T. H. H. Aldhyani et al., “Data analytics for the identification of fake reviews using supervised learning,” Computers, Materials & Continua, vol. 70, no. 2, pp. 3189–3204, 2022.
45. S. Bharany, S. Sharma, S. Badotra, O. I. Khalaf, Y. Alotaibi et al., “Energy-efficient clustering scheme for flying ad-hoc networks using an optimized leach protocol,” Energies, vol. 14, no. 6016, pp. 1–20, 2021.
46. C. A. T. Romero, J. H. Ortiz, O. I. Khalaf and A. R. Prado, “Business intelligence: Business evolution after industry 4. 0,” Sustainability, vol. 13, no. 10026, pp. 1–12, 2021.
47. N. Veeraiah, O. I. Khalaf, C. V. P. R. Prasad, Y. Alotaibi, A. Alsufyani et al., “Trust aware secure energy-efficient hybrid Protocol for MANET,” IEEE Access, vol. 9, pp. 120996–121005, 2021.
49. N. Jha, D. Prashar, O. I. Khalaf, Y. Alotaibi, A. Alsufyani et al., “Blockchain-based crop insurance: A decentralized insurance system for modernization of Indian farmers,” Sustainability, vol. 13, no. 16, pp. 1–17, 2021.
51. C. A. T. Romero, J. H. Ortiz, O. I. Khalaf and W. M. Ortega, “Software architecture for planning educational scenarios by applying an agile methodology,” International Journal of Emerging Technologies in Learning, vol. 16, no. 8, pp. 132–144, 2021.
55. O. Wisesa, A. Andriansyah and O. I. Khalaf, “Prediction analysis for business to business (B2B) sales of telecommunication services using machine learning techniques,” Majlesi Journal of Electrical Engineering, vol. 14, no. 4, pp. 145–153, 2020.
56. A. T. Hoang, X. P. Nguyen, O. I. Khalaf, T. X. Tran, M. Q. Chau et al., “Thermodynamic simulation on the change in phase for carburizing process,” Computers, Materials & Continua, vol. 68, no. 1, pp. 1129–1145, 2021.
57. X. Wang, J. Liu, O. I. Khalaf and Z. Liu, “Remote sensing monitoring method based on BDS-based maritime joint positioning model,” CMES-Computer Modeling in Engineering & Sciences, vol. 127, no. 2, pp. 801–818, 2021.
58. C. A. T. Romero, D. F. Castro, J. H. Ortiz, O. I. Khalaf and M. A. Vargas, “Synergy between circular economy and industry 4.0: A literature review,” Sustainability, vol. 13, no. 8, pp. 4331, 2021.
59. A. T. Hoang, X. P. Nguyen, O. I. Khalaf, T. X. Tran and M. Q. Chau, “Thermodynamic simulation on the change in phase for carburizing process,” Computers Materials and Continua, vol. 68, no. 1, pp. 1129–1145, 2021.
60. G. M. Abdulsahib and O. I. Khalaf, “An improved cross-layer proactive congestion in wireless networks,” International Journal of Advances in Soft Computing and Its Applications, vol. 13, no. 1, pp. 178–192, 2021.
61. G. M. Abdulsahib and O. I. Khalaf, “Accurate and effective data collection with minimum energy path selection in wireless sensor networks using mobile sink,” Journal of Information Technology Management, vol. 13, no. 2, pp. 139–153, 2021.
62. M. Krichen, S. Mechti, R. Alroobaea, E. Said, P. Singh et al., “A formal testing model for operating room control system using internet of things,” Computers, Materials & Continua, vol. 66, no. 3, pp. 2997–3011, 2021.
63. E. N. A. Khanak, S. P. Lee, S. U. R. Khan, N. Behboodian and O. I. Khalaf, “A heuristics-based cost model for scientific workflow scheduling in cloud,” Computers Materials and Continua, vol. 67, no. 3, pp. 3265–3282, 2021.
64. T. X. Tran, X. P. Nguyen, D. N. Nguyen, D. T. Vu, M. Q. Chau et al., “Effect of poly-alkylene-glycol quenchant on the distortion, hardness, and microstructure of 65 mn steel,” Computers Materials and Continua, vol. 67, no. 3, pp. 3249–3264, 2021.
65. C. A. T. Romero, J. H. Ortiz, O. I. Khalaf and A. R. Prado, “Web application commercial design for financial entities based on business intelligence,” Computers Materials and Continua, vol. 67, no. 3, pp. 3177–3188, 2021.
66. M. J. Awan, M. S. Mohd Rahim, H. Nobanee, A. Yasin, O. I. Khalaf et al., “A big data approach to Black Friday sales,” Intelligent Automation and Soft Computing, vol. 27, no. 3, pp. 785–797, 2021.
67. O. I. Khalaf, K. A. Ogudo and M. A. Singh, “Fuzzy-based optimization technique for the energy and spectrum efficiencies trade-off in cognitive radio-enabled 5G network,” Symmetry, vol. 13, no. 1, pp. 1–47, 2021.
68. O. I. Khalaf, F. Ajesh, A. A. Hamad, G. N. Nguyen and D. N. Le, “Efficient dual-cooperative bait detection scheme for collaborative attackers on mobile ad-hoc networks,” IEEE Access, vol. 8, pp. 227962–227969, 2020.
70. S. Sudhakar, O. I. Khalaf, G. R. K. Rao, D. K. Sharma, K. Amarendra et al., “Security-aware routing on wireless communication for e-health records monitoring using machine learning,” International Journal of Reliable and Quality E-Healthcare (IJRQEH), vol. 11, no. 3, pp. 1–10, 2022.
2. Delete content referencing References [7], [22], [23], [25–28], [31–39], [42–47], [49], [51], [55–68], [70] in the main text:
“The estimated number of people diagnosed with cancer would rise much more in the following years, hitting roughly 50% in 2040 than 2020 [5–9].”
“In mammography diagnostics, accurate abnormality diagnosis is critical. EBCD can make a significant difference in a patient’s long-term survival rate [20,21].”
“Microcalcification is tiny and has poor contrast with the mammography background because of its small size and low contrast, and It is sometimes challenging and time-consuming, which requires the radiologist to do a thorough assessment of microcalcifications [22,23].”
“The goal is to enhance CNN’s Discriminative Localization capacity to detect hazardous microcalcification zones in mammograms by applying Class ActivationMapping (CAM).”
“Gray Level Co-occurrence Matrix, and Curvelet Transform takes utilization of the positive characteristics of several extractors of characterization; the cumulated feature is demonstrated to be efficacious in the biopsy picture relegation challenge [24].”
“Medical diagnostic functions, for example, demand a high caliber of precision and dependability; as a result, the repudiation thresholds at each step would be set to a high caliber to ascertain that the diagnosis is proper [25].”
“This footage shows ebony backgrounds with thick white patches, possibly calcifications/masses [26,27].”
“Biopsies include removing cells or a tiny amount of breast tissue and sending it to a lab for pathologists to analyse in order to acquire conclusive results [28–30].”
“However, semi-supervised learning and Reinforcement Learning (RL) are irrelevant to the purpose of BCDD.”
“The method was evaluated on 322 mammography pictures from the MIAS database, yielding 94.59% sensitivity and 3.90% false positives per image data set.”
“Furthermore, the technique was tested 260 mammogram masses accumulated from the Dokuz Eylul Mammography Set (DEMS), and it attained 95.06% [31].”
“This network is benign since it requires less human labour and pre-processing [32].”
“As a result, early detection of breast cancer can be obtained by authorizing it to be treated at an inferior stage before it further spreads [33,34].”
The authors state that the scientific conclusions are unaffected. This correction was approved by the Computer Systems Science and Engineering Editorial Office. The original publication has also been updated.
Cite This Article
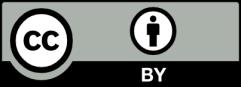
This work is licensed under a Creative Commons Attribution 4.0 International License , which permits unrestricted use, distribution, and reproduction in any medium, provided the original work is properly cited.