Open Access
ARTICLE
Breast Calcifications and Histopathological Analysis on Tumour Detection by CNN
1 Department of Computer Science and Engineering, Paavai Engineering College, Pachal, Namakkal, 637018, Tamil Nadu, India
2 Al-Nahrain University, Al-Nahrain Nanorenewable Energy Research Center, Baghdad, Iraq
3 COMBA R&D Laboratory, Faculty of Engineering, Universidad Santiago de Cali, 76001, Cali, Colombia
4 Department of Computer Science and Engineering, Kongu Engineering College, Perundurai, 638060, Tamil Nadu, India
5 Department of Mathematics, Jaypee University of Engineering and Technology, Guna, 473226, Madhya Pradesh, India
* Corresponding Author: D. Banumathy. Email:
Computer Systems Science and Engineering 2023, 44(1), 595-612. https://doi.org/10.32604/csse.2023.025611
Received 30 November 2021; Accepted 30 December 2021; Issue published 01 June 2022
A correction of this article was approved in:
Correction: Breast Calcifications and Histopathological Analysis on Tumour Detection by CNN
Read correction
Abstract
The most salient argument that needs to be addressed universally is Early Breast Cancer Detection (EBCD), which helps people live longer lives. The Computer-Aided Detection (CADs)/Computer-Aided Diagnosis (CADx) system is indeed a software automation tool developed to assist the health professions in Breast Cancer Detection and Diagnosis (BCDD) and minimise mortality by the use of medical histopathological image classification in much less time. This paper purposes of examining the accuracy of the Convolutional Neural Network (CNN), which can be used to perceive breast malignancies for initial breast cancer detection to determine which strategy is efficient for the early identification of breast cell malignancies formation of masses and Breast microcalcifications on the mammogram. When we have insufficient data for a new domain that is desired to be handled by a pre-trained Convolutional Neural Network of Residual Network (ResNet50) for Breast Cancer Detection and Diagnosis, to obtain the Discriminative Localization, Convolutional Neural Network with Class Activation Map (CAM) has also been used to perform breast microcalcifications detection to find a specific class in the Histopathological image. The test results indicate that this method performed almost 225.15% better at determining the exact location of disease (Discriminative Localization) through breast microcalcifications images. ResNet50 seems to have the highest level of accuracy for images of Benign Tumour (BT)/Malignant Tumour (MT) cases at 97.11%. ResNet50’s average accuracy for pre-trained Convolutional Neural Network is 94.17%.Keywords
Cite This Article
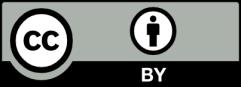