Open Access
ARTICLE
Prediction of Sound Transmission Loss of Vehicle Floor System Based on 1D-Convolutional Neural Networks
1 Guangzhou Automobile Group Co., Ltd., Automotive Research and Design Centre, Guangzhou, 511434, China
2 School of Mechanical Engineering, Southwest Jiaotong University, Chengdu, 610031, China
* Corresponding Author: Haibo Huang. Email:
Sound & Vibration 2024, 58, 25-46. https://doi.org/10.32604/sv.2024.046940
Received 19 October 2023; Accepted 02 January 2024; Issue published 06 February 2024
Abstract
The Noise, Vibration, and Harshness (NVH) experience during driving is significantly influenced by the sound insulation performance of the car floor acoustic package. As such, accurate and efficient predictions of its sound insulation performance are crucial for optimizing related noise reduction designs. However, the complex acoustic transmission mechanisms and difficulties in characterizing the sound absorption and insulation properties of the floor acoustic package pose significant challenges to traditional Computer-Aided Engineering (CAE) methods, leading to low modeling efficiency and prediction accuracy. To address these limitations, a hierarchical multi-objective decomposition system for predicting the sound insulation performance of the floor acoustic package has been developed based on an analysis of the noise transmission path. This approach involves introducing a 1D-Convolutional Neural Network (1D-CNN) model for predicting the sound insulation performance of the floor acoustic package, thereby avoiding the limitations of conventional CAE approaches that rely solely on “data-driven” methods. The proposed method was applied and tested using specific vehicle models, and the results demonstrated the effectiveness and superiority of the proposed approach relative to those obtained using 2D-CNN and Support Vector Regression (SVR) models.Keywords
Cite This Article
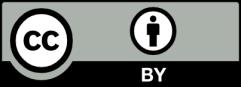
This work is licensed under a Creative Commons Attribution 4.0 International License , which permits unrestricted use, distribution, and reproduction in any medium, provided the original work is properly cited.