Open Access
ARTICLE
Application of Machine Learning for Tool Condition Monitoring in Turning
1 School of Mechanical and Building Sciences, VIT University, Chennai, Tamil Nadu, 600127, India
2 Department of Mechanical Engineering, College of Engineering, Pune, 411005, India
* Corresponding Author: R. Jegadeeshwaran. Email:
Sound & Vibration 2022, 56(2), 127-145. https://doi.org/10.32604/sv.2022.014910
Received 07 November 2020; Accepted 26 May 2021; Issue published 25 March 2022
Abstract
The machining process is primarily used to remove material using cutting tools. Any variation in tool state affects the quality of a finished job and causes disturbances. So, a tool monitoring scheme (TMS) for categorization and supervision of failures has become the utmost priority. To respond, traditional TMS followed by the machine learning (ML) analysis is advocated in this paper. Classification in ML is supervised based learning method wherein the ML algorithm learn from the training data input fed to it and then employ this model to categorize the new datasets for precise prediction of a class and observation. In the current study, investigation on the single point cutting tool is carried out while turning a stainless steel (SS) workpeice on the manual lathe trainer. The vibrations developed during this activity are examined for failure-free and various failure states of a tool. The statistical modeling is then incorporated to trace vital signs from vibration signals. The multiple-binary-rule-based model for categorization is designed using the decision tree. Lastly, various tree-based algorithms are used for the categorization of tool conditions. The Random Forest offered the highest classification accuracy, i.e., 92.6%.
Keywords
Cite This Article
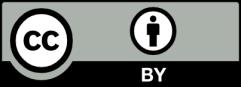
This work is licensed under a Creative Commons Attribution 4.0 International License , which permits unrestricted use, distribution, and reproduction in any medium, provided the original work is properly cited.