Open Access
ARTICLE
Using Audiometric Data to Weigh and Prioritize Factors that Affect Workers’ Hearing Loss through Support Vector Machine (SVM) Algorithm
1 Student Research Committee, Kerman University of Medical Sciences, Kerman, Iran
2 Associate Professor, Department of Occupational Health, School of Public Health, Kerman University of Medical Sciences, Kerman, Iran
3 Assistant Professor, Department of Occupational Health, School of Public Health, Kerman University of Medical Sciences, Kerman, Iran
4 Assistant Professor, Department of Computer Engineering, Shahid Bahonar University, Kerman, Iran
* Corresponding Author: Sajad Zare. Email:
Sound & Vibration 2020, 54(2), 99-112. https://doi.org/10.32604/sv.2020.08839
Received 15 October 2019; Accepted 10 December 2019; Issue published 09 May 2020
Abstract
Workers’ exposure to excessive noise is a big universal work-related challenges. One of the major consequences of exposure to noise is permanent or transient hearing loss. The current study sought to utilize audiometric data to weigh and prioritize the factors affecting workers’ hearing loss based using the Support Vector Machine (SVM) algorithm. This cross sectional-descriptive study was conducted in 2017 in a mining industry in southeast Iran. The participating workers (n = 150) were divided into three groups of 50 based on the sound pressure level to which they were exposed (two experimental groups and one control group). Audiometric tests were carried out for all members of each group. The study generally entailed the following steps: (1) selecting predicting variables to weigh and prioritize factors affecting hearing loss; (2) conducting audiometric tests and assessing permanent hearing loss in each ear and then evaluating total hearing loss; (3) categorizing different types of hearing loss; (4) weighing and prioritizing factors that affect hearing loss based on the SVM algorithm; and (5) assessing the error rate and accuracy of the models. The collected data were fed into SPSS 18, followed by conducting linear regression and paired samples t-test. It was revealed that, in the first model (SPL < 70 dBA), the frequency of 8 KHz had the greatest impact (with a weight of 33%), while noise had the smallest influence (with a weight of 5%). The accuracy of this model was 100%. In the second model (70 < SPL < 80 dBA), the frequency of 4 KHz had the most profound effect (with a weight of 21%), whereas the frequency of 250 Hz had the lowest impact (with a weight of 6%). The accuracy of this model was 100% too. In the third model (SPL > 85 dBA), the frequency of 4 KHz had the highest impact (with a weight of 22%), while the frequency of 250 Hz had the smallest influence (with a weight of 3%). The accuracy of this model was 100% too. In the fourth model, the frequency of 4 KHz had the greatest effect (with a weight of 24%), while the frequency of 500 Hz had the smallest effect (with a weight of 4%). The accuracy of this model was found to be 94%. According to the modeling conducted using the SVM algorithm, the frequency of 4 KHz has the most profound effect on predicting changes in hearing loss. Given the high accuracy of the obtained model, this algorithm is an appropriate and powerful tool to predict and model hearing loss.Keywords
Cite This Article
Citations
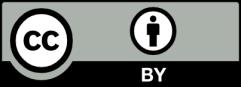