Open Access
ARTICLE
Structural System Identification Using Quantum behaved Particle Swarm Optimisation Algorithm
CSIR-Structural Engineering Research Centre, Council of Scientific and Industrial Research, Taramani, Chennai, India. - 600 113.
Email: arm2956@yahoo.com; arm@serc.res.in
Graduate Student Virginia Polytechnique Institute, USA.
Structural Durability & Health Monitoring 2013, 9(2), 99-128. https://doi.org/10.32604/sdhm.2013.009.099
Abstract
Development of efficient system identification techniques is highly relevant for large civil infrastructure for effective health monitoring, damage detection and vibration control. This paper presents a system identification scheme in time domain to estimate stiffness and damping parameters of structures using measured acceleration. Instead of solving the system identification problem as an inverse problem, we formulate it as an optimisation problem. Particle swarm optimisation (PSO) and its other variants has been a subject of research for the past few decades for solving complex optimisation problems. In this paper, a dynamic quantum behaved particle swarm optimisation algorithm (DQPSO) is proposed for the solution of the complex nonlinear optimisation problem associated with the system identification. If the uniqueness of the parametric solution is guaranteed for the assumed model, this heuristic method allows finding a solution without incurring restrictions of other classical optimisation methods, like the need for reliable initial estimates and convergence to local optima. In order to solve large size problems, we propose to use reduced order models. Proper orthogonal decomposition (POD) is used for the model reduction instead of traditional modal analysis. The validity of the proposed method is demonstrated by a numerical simulation study on a simply supported beam, 50 storey shear building model and a truss bridge. The robustness of the proposed system identification technique is investigated using a noise sensitivity study.Keywords
Cite This Article
Citations
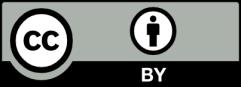
This work is licensed under a Creative Commons Attribution 4.0 International License , which permits unrestricted use, distribution, and reproduction in any medium, provided the original work is properly cited.