Open Access
REVIEW
Review of Artificial Neural Networks for Wind Turbine Fatigue Prediction
Windstorm Impact, Science and Engineering (WISE) Research Lab, Louisiana State University, Baton Rouge, LA 70803, USA
* Corresponding Author: Aly Mousaad Aly. Email:
Structural Durability & Health Monitoring 2024, 18(6), 707-737. https://doi.org/10.32604/sdhm.2024.054731
Received 01 February 2024; Accepted 07 June 2024; Issue published 20 September 2024
Abstract
Wind turbines have emerged as a prominent renewable energy source globally. Efficient monitoring and detection methods are crucial to enhance their operational effectiveness, particularly in identifying fatigue-related issues. This review focuses on leveraging artificial neural networks (ANNs) for wind turbine monitoring and fatigue detection, aiming to provide a valuable reference for researchers in this domain and related areas. Employing various ANN techniques, including General Regression Neural Network (GRNN), Support Vector Machine (SVM), Cuckoo Search Neural Network (CSNN), Backpropagation Neural Network (BPNN), Particle Swarm Optimization Artificial Neural Network (PSO-ANN), Convolutional Neural Network (CNN), and nonlinear autoregressive networks with exogenous inputs (NARX), we investigate the impact of average wind speed on stress transfer function and fatigue damage in wind turbine structures. Our findings indicate significant precision levels exhibited by GRNN and SVM, making them suitable for practical implementation. CSNN demonstrates superiority over BPNN and PSO-ANN in predicting blade fatigue life, showcasing enhanced accuracy, computational speed, precision, and convergence rate towards the global minimum. Furthermore, CNN and NARX models display exceptional accuracy in classification tasks. These results underscore the potential of ANNs in addressing challenges in wind turbine monitoring and fatigue detection. However, it’s important to acknowledge limitations such as data availability and model complexity. Future research should explore integrating real-time data and advanced optimization techniques to improve prediction accuracy and applicability in real-world scenarios. In summary, this review contributes to advancing the understanding of ANNs’ efficacy in wind turbine monitoring and fatigue detection, offering insights and methodologies that can inform future research and practical applications in renewable energy systems.Keywords
Cite This Article
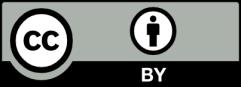
This work is licensed under a Creative Commons Attribution 4.0 International License , which permits unrestricted use, distribution, and reproduction in any medium, provided the original work is properly cited.