Open Access
REVIEW
Exploring the Applications of Digital Twin Technology in Enhancing Sustainability in Civil Engineering: A Review
1 School of Civil Engineering, Chongqing Jiaotong University, Chongqing, 400074, China
2 School of Civil Engineering and Architecture, NingboTech University, Ningbo, 315100, China
3 School of Civil Engineering, Lanzhou University of Technology, Lanzhou, 730050, China
* Corresponding Author: Ping Wu. Email:
Structural Durability & Health Monitoring 2024, 18(5), 577-598. https://doi.org/10.32604/sdhm.2024.050338
Received 03 February 2024; Accepted 10 April 2024; Issue published 19 July 2024
Abstract
With the advent of the big data era and the rise of Industrial Revolution 4.0, digital twins (DTs) have gained significant attention in various industries. DTs offer the opportunity to combine the physical and digital worlds and aid the digital transformation of the civil engineering industry. In this paper, 605 documents obtained from the search were first analysed using CiteSpace for literature visualisation, and an author co-occurrence network, a keyword co-occurrence network, and a keyword clustering set were obtained. Next, through a literature review of 86 papers, this paper summarises the current status of DT application in civil engineering based on a review of the origins, concepts, and implementation techniques of DTs, and it introduces the application of DTs in the full project lifecycle. This study shows that DTs have great potential to address many of the challenges faced by civil engineering. In this regard, the paper also presents some thoughts on the future directions of DT research.Keywords
Nomenclature
DT | Digital twin |
DTS | Digital twin scene |
ML | Machine learning |
AR | Augmented reality |
VR | Virtual reality |
BIM | Building information modelling |
IoT | Internet of things |
RFID | Radio frequency identification |
O&M | Operation and maintenance |
HBIMs | Heritage building information models |
With the prevalence of the Internet, widespread use of sensors, the emergence of big data, the development of e-commerce, the rise of information communities, and the integration of data and knowledge with social and physical spaces and cyberspace, the information environment for the development of artificial intelligence has undergone profound changes [1,2]. Information technology continues to innovate, driving the deep development of digitization, networking, and intelligentization, while also propelling the traditional civil engineering industry into a critical period of transformation and upgrading. Civil engineering has higher requirements in terms of talent, technology, and management, and it is also facing unprecedented challenges: informatization, digitization, networking, and intelligentization will become indispensable factors for the industry’s development.
A digital twin (DT) is a dynamic digital representation of a physical component that mimics its real-world behaviour [3]. By combining various data sources and integrating intelligent capabilities (such as artificial intelligence (AI), machine learning (ML), and data analytics) and digital models (such as building information modelling (BIM) models), a DT can represent and forecast current and future conditions. DTs date back to NASA’s development of two identical vehicles in the late 1960s, in which it used twins to simulate real-time behaviour for the remote updating, maintenance, and monitoring of the Apollo 13 spacecraft [4]. In 2013, Michael Grieves introduced the DT concept while at the University of Michigan [5]. In 2015, the DT concept was gradually applied and expanded to other areas, partly due to the rapid growth of the Internet of Things (IoT) in many industries [6].
The use of digital twins enables real-time correlation between digital models and physical structures, facilitating simulation and optimization of structures. By integrating digitalization, networking, intelligence, and informatization, the application of digital twin technology provides more accurate, reliable, and efficient analysis and decision support for civil engineering.
In traditional civil engineering projects, decisions are often based on experience and statistical data, which can lead to less satisfactory results. However, the emergence of digital twins has changed this situation. It enables precise modelling and analysis of civil engineering projects by real-time monitoring and collection of large amounts of data, providing decision-makers with more accurate and reliable information. Digital twins can not only help designers optimize the structure and layout of civil engineering projects but also predict and evaluate their behaviour and performance under different conditions. Almusaed et al. [7] discussed the application of artificial intelligence and digital twins in architectural design. AI algorithms can analyse various data of buildings to provide design options, while digital twins, as digital replicas of buildings, can be used to simulate and evaluate the effects and potential problems of these options, as well as predict their impact on building efficiency, comfort, and safety. During construction, digital twins can not only perform construction site simulations similar to Building Information Modelling (BIM), including risk prevention and site operations [8] but also simulate site debris path prediction for risk assessment [9], enable remote control and tracking monitoring of construction machinery [10], and predict potential component conflicts [11].
Digital twins also play an important role in the operation and maintenance management of civil engineering. Traditional inspection and maintenance often rely on manual inspections and periodic maintenance plans, which are inefficient and prone to overlooking issues. With digital twins, engineers can implement intelligent monitoring and maintenance for civil engineering, obtaining real-time information about the state and health condition of structures. Leveraging advanced sensor technologies and data analysis algorithms, digital twins can detect potential problems in structures in advance, reduce accident risks, and enable more effective maintenance strategies. In the field of roads and bridges, researchers have used unmanned aerial photography, 3D laser scanning, sensors, and digital twins to monitor and maintain road surfaces or bridges for detection of defects [12–16]. In the field of building construction, Huynh et al. [17] developed a building digital twin system using a web app to record the energy consumption data of buildings. From a design perspective, it can be highly customized to meet various user needs, while from a management perspective, it can maintain stable building performance and save energy consumption. Hosamo et al. [18] proposed a digital twin approach that integrates Building Information Modelling (BIM) with real-time sensor data and Bayesian networks to detect and predict HVAC issues that could affect comfort. Furthermore, utilizing machine learning algorithms for proactive maintenance and predictive control of buildings is also a promising direction.
However, the application of digital twins in the field of civil engineering faces some challenges and difficulties. First, the modelling process of digital twins requires high-quality input data, which may be difficult to acquire and organize. Second, the models and algorithms of digital twins are still evolving and need further research and validation.
Based on a comprehensive and systematic review of the literature, this paper presents directions for exploring the future of DTs in civil engineering, with the main aim of constructing a framework for the application of DTs in the civil engineering industry. To help in combing the literature, three objectives are specified:
(1) To collate the origins, definitions, and implementation techniques of DTs;
(2) To determine the application of DTs in the last decade from 2012 to 2023;
(3) To study the potential of DTs for application throughout the project lifecycle.
The rest of this paper is structured as follows: Section 2 describes the methodology used in this study. Section 3 introduces the origins, concepts and techniques of DTs. Section 4 discusses the current state of DT application in the civil engineering industry and the potential of DT application throughout the project lifecycle. Finally, the full text is summarised in Section 5, which presents an exploration of directions for future research on DTs.
2.1 Literature Search and Selection
As there are few papers in the Chinese literature dealing with both DTs and civil engineering, this paper focuses on literature produced elsewhere and uses Web of Science to conduct a literature search. The search subject term consists of two parts, which are connected by the relationship of “and”. The first part is “digital twin”. The “subject words” in the second part are “civil engineering”, “buildings”, “tunnel”, “bridge” and “tower”. In the second part, all subject words are connected by the “or” relation. The specific search method is as follows: TS = “digital twin” and TS = (“civil engineering” or “buildings” or “tunnel” or “bridge” or “tower”).
As the application of DTs in civil engineering is still in its early stages, the time frame for publication was set to 2012 to 2023. In addition, the type of literature was set to “thesis” or “review” to select more representative and authoritative articles for reading. A total of 605 articles were searched.
After completing the literature search, the obtained documents were included in the initial review. This involved skimming through the titles, abstracts, and keywords to select studies most closely aligned with the research topic. To avoid overlooking relevant material, after the initial screening, a search using the keywords “Digital twins” and “Civil engineering” was conducted using Scopus, and the references of the selected literature were further examined to identify potential related papers. In the end, a total of 86 documents were obtained.
2.2 Literature Visualisation Analysis
The 605 documents obtained from the initial search were analysed using CiteSpace.
2.2.1 Author Co-Occurrence Analysis
As illustrated in Fig. 1 and summarized in Table 1, the analysis focused on authors who have contributed to multiple publications. Among them, 79 first authors stand out, each having published 2 papers. Notably, Liu emerges as the most prolific publisher, with 8 papers to their credit. In total, 222 authors have made contributions to at least one paper.
Figure 1: Author co-occurrence
Examining Fig. 1 reveals a concentration of close collaborations among authors such as Lee, Kim, Park, Chang, and Jiang in the lower right quadrant. Despite this result, the overall count of scholars engaged in close collaboration appears relatively limited.
This analysis underscores the growing interest in the application of digital twins within civil engineering and construction over the past 7 years. However, the research landscape appears fragmented, with a small number of authors contributing frequently. Collaborative efforts are observed to be more concentrated among authors with fewer publications, indicating a narrow base for cooperative research.
2.2.2 Keyword Co-Occurrence Analysis
To gain insights into the current research focal points of digital twins in civil engineering and construction engineering, a keyword co-occurrence map was generated using CiteSpace, where nodes represent keywords. Fig. 2 depicts the resulting map, comprising 295 nodes and 1482 edges. Keywords, as integral components of studies, encapsulate the essence of research content and, to a considerable extent, mirror the developmental focus of digital twins within the industry. This mapping result is invaluable for researchers seeking to comprehend the academic frontier.
Figure 2: Keyword co-occurrence
As outlined in Table 2, keywords with the highest frequencies are “digital twin(s),” “design,” “system,” “BIM,” “model,” “performance,” “framework,” “management,” “structural health monitoring,” and “internet.” These terms span diverse elements and dimensions of civil engineering, forming a logically connected framework within the study.
2.2.3 Keyword Cluster Analysis
On the basis of the keyword co-occurrence network, keyword clustering analysis was carried out. To better understand the specific information contained in hot words from only cluster names, it is necessary to include cluster sublabels beneath the clusters for in-depth analysis. These sublabels are extracted through methods such as co-occurrence analysis of literature or topic modelling, helping researchers understand the primary research directions or hot topics within a collection of literature. By observing the changes in cluster sublabels, one can identify the development trends in specific fields, as well as the changes and evolution of keywords.
A total of 19 classes were obtained, 10 of which were selected for presentation, as shown in Fig. 3. Combined with the tag words of each class, specific in-depth analysis was carried out, as shown in Table 3. For example, in the first category, “building”, the literature mainly focuses on digital twins, in situ surveys, footprint identification, sky view factors, tree canopies, digital twin augmented reality, mobile augmented reality, mobile systems, human–device interactions, and human behaviour. In the second category, “internet of things”, the literature focuses on digital twins, building information, the construction industry, cyber-physical systems, smart building or building information modelling, facility management, predictive maintenance, data-driven buildings, virtual reality, the use of digital information technology, and equipment and products.
Figure 3: Keyword clustering
2.3 Analysis of Selected Studies
The selected literature was analysed in terms of the publication date and journal of publication. The results are shown in Fig. 4. DT technology gained attention in civil engineering only in 2018, when a certain amount of research began to appear and gradually increased over a five-year period, which can be attributed to the rapid development of IoT technology in various industries and the urgent need for industry intelligence.
Figure 4: Distribution of the years of publication of the literature
The research directions of the 86 articles were analysed, and the results are shown in Tables 4 and 5 (Note: The citation of literature in the table only provides a brief classification of the research directions of the 86 papers and their application stages in the project, without involving conceptual explanations or other purposes). The application of DTs in civil engineering can involve buildings, bridges, tunnels, and concrete structures, while the construction industry is the main application area of DTs in civil engineering. Because there are far more completed projects than under construction or new projects, in the long-term operation and use, the structure will be affected by factors such as natural environment, load and loss, requiring more monitoring, maintenance and repair work, and digital twins can provide reliable and efficient solutions for these tasks. DTs in the project operation and maintenance (O&M) phase are the main direction of research on the whole project lifecycle.
To provide a better overview, this paper identifies three questions:
(1) What is a DT? What are its origins, concepts and implementation techniques?
(2) What is the current status of the application of DTs in civil engineering? How are they applied in the whole project lifecycle?
(3) What are the opportunities and challenges brought by DTs in civil engineering?
These three aspects are also described in the following section.
3 The Origins, Concepts and Implementation Techniques of Digital Twins
3.1 The Origins and Concepts of Digital Twins
With advancements such as the IoT, augmented reality (AR), 3D printing, AI, cloud computing, and other technologies that capture and analyse large data, Industry 4.0 has sparked a global race over the past ten years to modernise industry. These cutting-edge technologies merge the physical and digital worlds, giving rise to the DT concept in response to the many difficulties and complexities of industry as well as rising consumer demands.
Currently, there is no precise definition for a DT, but most scholars describe a DT as a digital copy of an object, process or system that can be used for various purposes [24]. In 2003, Michael Grievest introduced the “digital equivalent to a physical product”, which is a digital representation of a current physical product and is widely considered to be the first introduction of the DT concept [36]. In 2006, Hribernik [90] introduced the concept of “product incarnation”, meaning the development of an information management architecture to support a product-centric, two-way information flow. In 2012, Glaessegen and Stargel proposed a more complete definition: a DT is a multi-physical, multi-scale and integrated probabilistic simulation of a complex product, using the best available physical model, sensor updates, etc., to reflect the lifetime of its corresponding twin [6]. In 2018, Bacchiega simplified the DT concept by defining a DT as “a real-time digital copy of the physical object” [89].
It is the understanding of this paper that a DT is a “physical-information” ensemble that should consist of three components:
(1) The physical component can include assets, processes, systems, buildings or even services;
(2) Digital model: A digital copy or virtual counterpart of a physical component containing the structural model and the data core.
(3) Bi-directional exchange of data or information between the digital model and the physical entity: Real data from the “physical” part are uploaded to the digital model. The digital model leverages its inherent structure and AI to process and evolve this information dynamically to manage the daily use of the physical part [33].
In the field of civil engineering, a digital twin refers to the virtual representation of a physical structure or engineering. This virtual model combines real-time data from physical entities (projects, engineering, structures, components, etc.) with simulation technology to reflect their behaviour, performance, and characteristics, including their mechanical performance [67], environmental safety [76], energy consumption [29], structural health [44], etc. In civil engineering, digital twins enable engineers to monitor, analyse, and optimize the performance of structures throughout their entire lifecycle.
Some scholars equate digital twins with BIM. In fact, digital twins should be seen as a further enhancement or evolution of BIM or as one of the carriers of digital twins, with BIM solely fulfilling the role of the digital model. BIM is a digital representation method based on three-dimensional models, used for collaborative work and information sharing during the design and construction processes. Digital twins, on the other hand, have a broader scope, not only focusing on the geometric information of buildings but also encompassing physical and functional characteristics, as well as real-time data connected to physical entities. Digital twins collect real-time data through sensors and other data collection devices, interact with digital models, and enable real-time monitoring, prediction, and optimization. In addition, digital twins are dynamic, constantly changing models that support interactive processes [43]. In other words, digital twins go beyond BIM.
3.2 Implementation Techniques of Digital Twins
The utilisation of DTs involves the use of numerous high-fidelity modelling approaches, data collection techniques, simulation, or data processing techniques.
Digital models for DTs can be divided into semantic models and 3D models. Semantic models are usually implemented through programming languages, and 3D models can be subdivided into geometric modelling, physical modelling, and object behaviour modelling. 3D Studio Max is one of the most commonly used 3D modelling software programs in the world, while BIM is commonly used for modelling in the civil engineering industry. The fidelity of the model can be improved by adding physical properties (hardness, surface roughness, tolerances, density, etc.) [4].
The data information for a DT comes mainly from devices, people and the environment, and the data collected are divided into two categories: real-time data and historical data. Real-time data can be provided by wired connections (e.g., RS485, Ethernet), smart sensors (e.g., IoT), and wireless connections (e.g., radio frequency identification (RFID), Bluetooth, 4G, 5G). Methods such as laser scanning by drones and photogrammetry can also be used to collect external information on the “physical” part of a site. These technologies are constantly generating new structured, semi-structured, and unstructured data, which can be difficult and expensive to upload to the cloud due to their sheer size, and edge computing can be used to pre-process the data [36]. Furthermore, 5G technologies can be used to avoid data leakage during transmission and to ensure real-time data transfer.
To analyse these data and feed useful information back to the “physical” part of reality, AI is introduced into the DT framework for event recognition, fault diagnosis and automated decision making after data integration. Natural language processing (NLP) and clustering algorithms are used to discover failure modes from routine maintenance [34]. Finite element analysis (FEA) can be used to model changes such as object bending and deformation. Predictive analysis can be applied to larger datasets through regression and machine learning techniques, combining real-time and historical data to provide diagnostic scenarios that continuously improve the predictive internal logic of the model by identifying the fields that best explain the results for further optimisation [4].
Finally, AR technology can present real-time data and knowledge derived from digital models to users to help them monitor and respond to the “physical” part of the process over time.
4 Application of Digital Twins in Civil Engineering
4.1 Current Status of Application
Increased urbanisation is putting pressure on urban transport. The construction of underground tracks, the maintenance and repair of road surfaces, and the lifecycle monitoring of bridges are all issues that need urgent attention and solutions, leading to a gradual shift towards the modernisation and digitisation of the whole lifecycle of transport facilities. Therefore, the application of DTs in infrastructure is also mainly focused on the O&M phase. Lu et al. [53] proposed a digital model of existing reinforced concrete bridges based on a cluster of marker points to develop a bridge management system. Shim et al. [13] built a digital bridge model based on BIM technology; not only can changes to the project be tracked, but data can also be provided for the inspection of the bridge. In addition, all inspection data and damage records for the bridge are stored and updated in the model, and on this basis, the structural characteristics are evaluated to continuously ensure the performance of the entire bridge system throughout its lifecycle.
The most ambitious and complex application of DT technology is the “smart city” [16]. By linking digital models to data and information about a city, such as topographical data, existing and planned building data, census data, socio-economic data, energy consumption data, and maintenance management data, an information model of the city is created. In a digital city twin, not only can individual parameters within the city be analysed, such as the effects of hot and cold air movement, but design ideas from city residents, such as buildings, development areas, green spaces, or trees, can also be collected by creating 3D interactive applications. Then, the completed models can be submitted to the city administration [21]. A digital city twin is essentially a collection of multiple DTs that use IoT technology to connect and map entire cities onto digital models. Such models can be used for future assessments, for example, regarding the impact of changes in building density on the city’s climate or traffic, or for operational management, such as urban planning, to provide additional solutions that contribute to improving the quality of life of citizens.
In the last few years of research, DT technology has also been used for quality assessment of individual components or non-destructive testing of materials. Tran et al. [14] developed a new framework for the geometric quality assessment of prefabricated façade components during construction based on a DT approach using 3D models and semantic models, and they provided a visual quality assessment solution that can be used in digital models to locate construction errors and to identify missing and redundant elements in the digital models. During construction, this information enhances the closed-loop interaction and information exchange between the physical building façade and the digital building, which is of relevance for the construction of assembled buildings.
In addition, DTs can be used for risk management. Kamari et al. [9] proposed a computer vision-based risk assessment method for geometric DTs of site debris to analyse the safety risks and predict hazards of site debris to the nearby built environment. Using an intelligent drone path planning simulation system based on a construction site as a visualisation of the geometric DT to quantify the amount and impact of construction site debris, the method identifies potential risks to nearby communities from construction materials and can help mitigate damage and losses during extreme wind events by allowing practitioners to take better measures and protect the site before the events occur.
4.2 Application of Digital Twins to the Full Project Lifecycle
Digital twinning of the environment and surroundings, related existing projects, earlier generations of target projects, and already built target projects can be carried out during the design phase, during which some new design projects have not yet been built and retrofit projects have been built, to help with the design of new projects or retrofit designs [85].
According to Fig. 5, first, in the preparation phase, digital twins provide designers with powerful tools and resources to carefully plan and make decisions for potential projects. With the help of digital twin technology, designers can establish a realistic and dynamic digital model based on various data sources, reflecting the characteristics and conditions of the actual project. Through digital twins, designers can explore different options and set various constraints in the early stages of a project, including budget limitations, time requirements, sustainability goals, and specific technical requirements. Using digital twin models, designers can evaluate the feasibility and potential impacts of each option, providing guidance for future design processes. Furthermore, digital twins help designers define and optimize project objectives, ensuring a balance with the interests of all relevant stakeholders and providing a clear vision and direction. Designers can also interact with and visualize design decisions in real time on the digital models. This collaborative approach helps design teams work together to solve problems, explore innovative solutions, and ensure design consistency and accuracy.
Figure 5: Application of a digital twin in the design phase
Second, to help with conceptual, preliminary, and thorough design, the DT can incorporate data from numerous sources, including pertinent already existing projects, environments, surroundings, and design papers [91]. By collecting and integrating data from previous similar projects, such as project background, historical data, design files, etc., designers can have references and inspirations for the current project. Integrating relevant data to provide accurate environmental information helps designers fully consider and address these factors during the design process. Throughout the design process, digital twins provide continuous support and feedback through continuous evaluation and upgrading with design documents and real-world data. They can be compared and validated against actual construction processes and on-site monitoring data, helping designers verify the rationality of the design and make timely adjustments and improvements when needed.
Finally, the data collected make it possible to use DTs to estimate designs, reduce design flaws, and resolve inconsistencies between the actual and intended designs. Through setting the database structure and interaction mode in the system and the use of computer technologies such as artificial intelligence, machine learning, and deep learning, simulation work or experiments that cannot be carried out in reality can be simulated in the design process, such as building wind environment simulation, sound environment simulation, sunlight simulation, emergency evacuation simulation, building energy consumption simulation, building seismic performance simulation, and building carbon emission simulation [40]. The design is successfully redesigned, enhanced, updated, and verified based on the simulation results, which saves time, money, and labour through the use of data analysis and predictions.
In the digital information age, smart construction sites are developing very rapidly, and many digital tools are being implemented, such as cyber-physical systems (CPS), BIM, laser scanning, sensing, RFID, and web technology [85]. Boje et al. [33] defined a “construction DT” framework: (1) an enhanced version of construction site BIM; (2) a construction monitoring platform integrating all IoT devices; and (3) a DT for autonomous updating and autonomous learning using ML, deep learning, data mining and other technologies.
According to Fig. 6, in the pre-construction stage, the digital model is used to simulate construction progress and key difficult construction plans, and the construction plans are reasonably revised and updated based on the simulation results and data analysis. By combining DTs and AR, virtual construction sites, outdoor construction environments and worker training environments can also be simulated. Because the construction DT allows immersion in virtual construction site simulations and risk-free experience of hazardous situations and learning opportunities, it can safeguard workers’ safe behaviour and avoid risks during real-life production processes. In addition, a DT combined with AR can be used for risk prediction and safety management by developing AR systems that alert operators at the workplace in real time to hazardous events occurring around them for timely analysis and treatment [8].
Figure 6: Application of a digital twin in the construction phase
During the construction process, as the physical parts of the target project are not yet completed, digital twinning can be carried out for other related existing projects, related environments, associated environments, and parts of the target project under construction to facilitate construction monitoring and management, including monitoring and management of construction progress, quality, safety, workers, machinery and materials. On the one hand, digital twinning can be combined with machine vision technology to achieve timely, efficient and accurate model reconstruction for quality monitoring and on-site worker management; on the other hand, it can be combined with IoT technology for connectivity and real-time communication between physical parts, virtual parts and the central database on site.
In addition, visualisation and computer vision technologies allow detailed interior construction progress to be monitored. By visualising and comparing the as-built 3D model in BIM with as-built photographs, the as-built interior construction objects are decomposed, and the status of construction progress is automatically generated [92].
4.2.3 Operation and Maintenance Phases
Civil engineering structures undergo evolution and changes during usage, for example, due to natural aging, load variations, or external factors. The characteristics of digital twin virtual interaction and real-time feedback are beneficial for real-time monitoring and tracking of structural changes, providing corresponding prediction and optimization strategies, and enhancing the informatization and automation of project operation and maintenance management.
Peng et al. [34] developed a digital twin for a hospital that was primarily designed for the operations and maintenance (O&M) phase. This digital twin seamlessly integrates both static and dynamic information across the pre-design, construction, and O&M phases. Furthermore, it incorporates mixed reality (MR) applications, merging digital representations with real-world scenarios and presenting them in a visually intuitive interface for the managerial observation of the dynamic conditions in hospitals. To enhance decision-making, an AI-driven diagnostic engine is introduced, capable of assimilating vast amounts of dynamic information and synchronizing with real-world data.
The application of digital twins in the O&M stage is primarily characterized by the integration of static and dynamic data from the pre-project phase, enabling real-time updates and responses. Leveraging artificial intelligence, deep learning, and finite element simulation technologies, these integrated data sets can be utilized to predict potential risks, failures, and defects or simulate factors such as energy consumption and the building environment. Subsequently, employing database technology, case-based reasoning, rule recognition, and other methods facilitates swift decision-making for managers. In essence, the digital twin in the O&M phase should support three fundamental functions: monitoring, analysis, and decision-making, as illustrated in Fig. 7. This process entails various levels, encompassing data collection, transmission, processing, modelling, simulation, and decision analysis. Successful implementation requires the synergistic use of technologies and tools such as the Internet of Things (IoT), artificial intelligence, and big data.
Figure 7: Application of a digital twin in the O&M phase
At this stage, no examples of the application of digital twins can be found. However, the Dunhuang Research Institute has been engaging in “Digital Dunhuang” research activities since 2016. Apart from creating a digital backup of the cultural heritage as a whole and developing the functionality of an online museum, digital twins may also be discovered to have the potential for application in the monitoring, protection, and restoration of architectural cultural heritage. Vuoto et al. [43] proposed the application roadmap of digital twin models in the preservation of architectural cultural heritage. Kong et al. [59] integrated photogrammetry techniques and point cloud processing algorithms into a digital twin framework to perform structural health monitoring on a historic stone arch bridge.
Using 3D laser scanning technology to accurately record the spatial position, dimensions, and textures of façades as well as components of heritage buildings for conservation, creating 3D reconstructions of historic buildings that are realistic in style, and simulating the best restoration solution for such projects through AI algorithms will help relevant practitioners work on difficult-to-restore ancient buildings.
In addition, as the DT model retains all data from the full lifecycle of the project during the end-of-life phase, it can be used in the next phase of the rebuilding project to solve problems in this phase that are similar to those that occurred in the previous phases of the project. This aspect also shows the considerable potential of DTs in urban regeneration and old city renovation. With the DT concept, a city information modelling (CIM) platform can be established to provide an accurate reference for old city regeneration projects, livelihood improvement, and urban management projects by analysing the current business situation, public service resources, and supporting infrastructure in a specific area to achieve simultaneous planning, construction, and renovation of the digital city and the real city and to improve the efficiency of renovation projects.
4.3 Application of Digital Twins to Structural Durability and Health Monitoring
In terms of structural durability and health monitoring, digital twins allow engineers to continuously assess the condition of structures and predict their behaviour over time. Currently, numerous scholars are attempting to integrate digital twin technology into the structural health monitoring of facilities such as buildings [44], historical architectural heritage [59], and bridges [55].
Cheok et al. [50], addressing the structural issues of X-type welded circular steel tube nodes under axial support forces, proposed a localized digital twin based on strain interfaces. They applied a series of machine learning algorithms to identify and locate cracks and quantify the severity of the cracks. Feng et al. [49] introduced a neural network-guided particle filtering algorithm for crack monitoring in welded plate connections, offering a potential solution for crack size monitoring in the context of digital infrastructure.
Combining digital twin technology with structural health monitoring methods involves, first, utilizing DT technology to digitize and visualize elements of structural health monitoring, such as geometric dimensions, structural behaviour, and damage. By integrating sensors, monitoring devices, and data analysis into a digital twin, engineers can track parameters such as stress, strain, vibration, temperature, and corrosion. Subsequently, damage can be identified and quantified using algorithms such as machine learning. Analysis and prediction of future structural behaviour, including state and lifespan predictions, may be conducted to proactively detect potential issues or risks, allowing for active maintenance and intervention to prevent structural failures or degradation. Furthermore, digital twins can simulate various scenarios to assess the impact of environmental factors, operating conditions, and ageing on structural integrity. Finally, the combination of artificial intelligence and big data technology enables decision-making based on predictions. Through computational models and predictive analysis, engineers can optimize maintenance plans, enhance safety, and extend the lifespan of infrastructure. In summary, digital twins play a crucial role in improving the durability, resilience, and sustainability of civil engineering structures.
This paper introduces DTs in terms of their origin, definition, and implementation, and it analyses the current status of their application in civil engineering. There is no exact DT concept, and the definition of DT may vary slightly from field to field. In civil engineering, a DT is generally considered to be an extension and derivative of BIM technology. According to current research, from the perspective of the physical component, DTs can be used in a number of ways, such as smart cities, infrastructure, facilities and equipment, and material components. From the virtual technology perspective, DTs use a large amount of modelling, simulation and analysis software and a variety of big data algorithms. The paper also discusses the application of DTs in various processes of civil engineering based on the design, construction, and O&M phases of a project.
5.1 Opportunities and Challenges
Over the past few years, an increasing number of scholars have focused on the potential of DTs in civil engineering and have explored various directions in the field to some extent. DTs offer the opportunity to combine the physical world with the digital world, helping to address many of the challenges faced in civil engineering today and helping to unite civil engineering with other industries. However, most research is still at the theoretical stage of framework construction, emphasising “what” and missing the key part of “how”.
On the one hand, a DT is an enormous project, and a successful twin should involve all the processes of a project from birth to death, with real-time changes in the process, making it difficult to operate. The static and dynamic data of physical entities are redundant, and data collection is difficult, leading to a lack of hierarchy and depth in terms of application. On the other hand, a digital city twin involves 5G, big data-connected IoT technologies, big data loading technologies, cloud computing collaboration technologies, simulation technologies, etc. The current technological maturity is not high, and the standardisation of platform models lags behind. These are all issues that need to be addressed in future research.
5.2 Future Research Directions
DTs show great potential in a wide range of industries. As the civil engineering industry transforms and changes, new opportunities for future research on DTs will emerge.
(1) Urban regeneration. Urban renewal is a necessary path to sustainable urban development, and the digital transformation of cities is an important goal and content of urban renewal today, as well as a powerful tool and instrument to support it. Establishing an urban DT, integrating the city’s cultural positioning and planning objectives into the digital world, building a concise mapping of urban regeneration, and establishing an open platform are conducive to increasing attention and participation in urban regeneration and absorbing evaluation and suggestions for urban planning. At the same time, a DT can efficiently integrate urban resource data, provide timely monitoring and response to urban disease problems, and improve the efficiency of urban regeneration by retaining old city data in the next phase (new construction and projects under construction) during renovation. In addition, through the creation of a city app and other vehicles, the DT system will be made available to the community, enabling virtual browsing, information disclosure, cultural publicity, business subscriptions and other cultural, commercial and social services, creating a unique intellectual property (IP) attribute of the city.
(2) Heritage O&M. DTs can be combined with cultural heritage building information models (HBIMs). First, a detailed HBIM can be developed using AR and virtual reality (VR) to create an “online museum” that will increase users’ interest in cultural tourism, enable wider display, dissemination, and sharing of buildings, and promote the refinement, publicisation and sustainability of architectural heritage conservation. Second, the DT and HBIM approach can also expand the information dimension of cultural heritage buildings, continuously integrate the digital models of heritage and historical buildings, integrate their historical information, help managers to monitor the safety of heritage, survey diseases, identify potential dangers, develop technical solutions, and realise intelligent O&M management. For damaged and destroyed relics that are difficult to repair, a model of the relics can be established, and repair solutions can be continuously simulated to find the optimal solution.
(3) Low-carbon construction. The 2020 Global Status Report on Construction states that in 2019, the total carbon emissions generated by the global construction sector were approximately 1 billion tonnes, accounting for 28% of total global carbon emissions. Additionally, if the carbon emissions from the production of construction materials were taken into account, the construction sector would account for 38% of total global carbon emissions, the highest of all sectors. Carbon emissions from construction have attracted international attention, and therefore, construction cannot be developed in the same crude manner as in the past, consuming large amounts of resources and producing a large amount of carbon emissions. By establishing a BIM model of building construction, introducing low-carbon information such as a visualisation of carbon emissions, the low-carbon cost, and carbon emission analysis to establish a detailed low-carbon information base, and integrating existing construction data information (building function, schedule, cost, resource consumption, etc.), a real-time measurement of carbon emissions and calculation of the corresponding low-carbon cost as well as an analysis of the degree of impact of each construction action on carbon emissions can be realised.
(4) Lifecycle cost optimization of civil engineering projects. Digital twin technology provides monitoring, optimization, and management capabilities throughout the entire lifecycle of a project, integrating information from different stages into a single model to facilitate improved asset management [29]. During the design phase, selecting economical and sustainable design solutions and utilizing DT technology to optimize structures, materials, construction processes, and energy consumption help reduce construction costs. In the construction phase, real-time monitoring of construction process data compared to the expected model allows for the timely identification and resolution of issues and prevents delays, cost overruns, and safety risks, thereby reducing construction costs. During the operation and maintenance phase, continuous monitoring of the operational status of engineering facilities, prediction of maintenance needs, and optimization of maintenance plans minimize maintenance costs and downtime. By simulating the impact of different decision scenarios, digital twin technology can assist project managers in formulating the most cost-effective strategy for the entire lifecycle, contributing to the sustainable improvement of resilience in civil engineering projects.
Acknowledgement: None.
Funding Statement: The authors wish to acknowledge that they were supported by the Key Research and Development Program of Zhejiang (Grant No. 2023C03183), the Natural Science Foundation of Zhejiang Province (Grant No. LY23E080005) and Science and Technology Project of Zhejiang Provincial Department of Transport (Grant No. 202225).
Author Contributions: The authors confirm contribution to the paper as follows: Writing—original draft, Investigation, Data collection: Jiamin Huang; Conceptualization, Methodology, Investigation: Ping Wu; Writing—review & editing: Wangxin Li; Funding acquisition: Jun Zhang; Study conception and design, Funding acquisition: Yidong Xu. All authors reviewed the results and approved the final version of the manuscript.
Availability of Data and Materials: The data and materials utilized in this study are available upon request from the corresponding author, Ping Wu, upon reasonable inquiry. For inquiries regarding the availability of specific data or materials, please contact Ping Wu at wuping@nit.zju.edu.cn.
Conflicts of Interest: The authors declare that they have no conflicts of interest to report regarding the present study.
References
1. Pan YJE. Heading toward Artificial Intelligence 2.0. Engineering. 2016;2(4):409–13. [Google Scholar]
2. Li BH, Hou BC, Yu WT, Lu XB, Yang CW. Applications of artificial intelligence in intelligent manufacturing: a review. Front Inf Technol Electron Eng. 2017;18(1):86–96. [Google Scholar]
3. Lu Q, Chen L, Li S, Pitt M. Semi-automatic geometric digital twinning for existing buildings based on images and CAD drawings. Autom Constr. 2020;115(4):103183. doi:10.1016/j.autcon.2020.103183. [Google Scholar] [CrossRef]
4. He F, Ong SK, Nee AYC. An integrated mobile augmented reality digital twin monitoring system. Computers. 2021;10(8):99. doi:10.3390/computers10080099. [Google Scholar] [CrossRef]
5. Minerva R, Lee GM, Crespi N. Digital twin in the IoT context: a survey on technical features, scenarios, and architectural models. Proc IEEE. 2020;108(10):1785–824. doi:10.1109/jproc.2020.2998530. [Google Scholar] [CrossRef]
6. D’Amico D, Ekoyuncu J, Addepalli S, Smith C, Keedwell E, Sibson J, et al. Conceptual framework of a digital twin to evaluate the degradation status of complex engineering systems. Procedia CIRP. 2019;86(9):61–7. doi:10.1016/j.procir.2020.01.043. [Google Scholar] [CrossRef]
7. Almusaed A, Yitmen I. Architectural reply for smart building design concepts based on artificial intelligence simulation models and digital twins. Sustainability. 2023;15(6):4955. doi:10.3390/su15064955. [Google Scholar] [CrossRef]
8. Schiavi B, Havard V, Beddiar K, Baudry D. BIM data flow architecture with AR/VR technologies: use cases in architecture, engineering and construction. Autom Constr. 2022;134:104054. doi:10.1016/j.autcon.2021.104054. [Google Scholar] [CrossRef]
9. Kamari M, Kim J, Ham Y. Analyzing safety risk imposed by jobsite debris to nearby built environments using geometric digital twins and vision-based deep learning. J Comput Civ Eng. 2022;36(6):04022033. doi:10.1061/(asce)cp.1943-5487.0001044. [Google Scholar] [CrossRef]
10. Hasan SM, Lee K, Moon D, Kwon S, Jinwoo S, Lee S. Augmented reality and digital twin system for interaction with construction machinery. J Asian Archit Build Eng. 2021;21(2):564–74. doi:10.1080/13467581.2020.1869557. [Google Scholar] [CrossRef]
11. Rausch C, Haas C. Automated shape and pose updating of building information model elements from 3D point clouds. Autom Constr. 2021;124:103561. doi:10.1016/j.autcon.2021.103561. [Google Scholar] [CrossRef]
12. Wang W, Xu X, Peng J, Hu W, Wu D. Fine-grained detection of pavement distress based on integrated data using digital twin. Appl Sci. 2023;13(7):4549. doi:10.3390/app13074549. [Google Scholar] [CrossRef]
13. Shim CS, Dang NS, Lon S, Jeon CH. Development of a bridge maintenance system for prestressed concrete bridges using 3D digital twin model. Struct Infrastruct Eng. 2019;15(10):1319–32. doi:10.1080/15732479.2019.1620789. [Google Scholar] [CrossRef]
14. Tran H, Nguyen TN, Christopher P, Bui DK, Khoshelham K, Ngo TD. A digital twin approach for geometric quality assessment of as-built prefabricated façades. J Build Eng. 2021;41(3):102377. doi:10.1016/j.jobe.2021.102377. [Google Scholar] [CrossRef]
15. Fokaides P, Jurelionis A, Spudys P. Boosting research for a smart and carbon neutral built environment with digital twins (SmartWins). In: 2022 IEEE International Smart Cities Conference (ISC22022; Pafos, Cyprus. [Google Scholar]
16. Bado MF, Tonelli D, Poli F, Zonta D, Casas JR. Digital twin for civil engineering systems: an exploratory review for distributed sensing updating. Sensors. 2022;22(9):3168. doi:10.3390/s22093168. [Google Scholar] [PubMed] [CrossRef]
17. Huynh D, Nguyen-Ky S. Engaging building automation data visualisation using building information modelling and progressive web application. Open Eng. 2020;10(1):434–42. doi:10.1515/eng-2020-0054. [Google Scholar] [CrossRef]
18. Hosamo HH, Nielsen HK, Kraniotis D, Svennevig PR, Svidt K. Improving building occupant comfort through a digital twin approach: a Bayesian network model and predictive maintenance method. Energy Build. 2023;288(1):112992. doi:10.1016/j.enbuild.2023.112992. [Google Scholar] [CrossRef]
19. Gelder J. Uniclass 2015 for smart cities. In: Proceedings of the International Conference of Architectural Science Association Auckland, 2020; Auckland, New Zealand. [Google Scholar]
20. Lehner H, Dorffner L. Digital geoTwin Vienna: towards a digital twin city as geodata hub. PFG-J Photogramm Rem. 2020;88(1):63–75. doi:10.1007/s41064-020-00101-4. [Google Scholar] [CrossRef]
21. Schrotter G, Hürzeler C. The digital twin of the city of zurich for urban planning. PFG-J Photogramm Rem. 2020;88(1):99–112. doi:10.1007/s41064-020-00092-2. [Google Scholar] [CrossRef]
22. Visconti E, Tsigkanos C, Hu Z, Ghezzi C. Model-driven engineering city spaces via bidirectional model transformations. Softw Syst Model. 2021;20(6):2003–22. doi:10.1007/s10270-020-00851-0. [Google Scholar] [PubMed] [CrossRef]
23. Keskin B, Salman B, Koseoglu O. Architecting a BIM-based digital twin platform for airport asset management: a model-based system engineering with SysML approach. J Constr Eng Manag. 2022;148(5):04022020. doi:10.1061/(asce)co.1943-7862.0002271. [Google Scholar] [CrossRef]
24. Tchana Y, Ducellier G, Remy S. Designing a unique digital twin for linear infrastructures lifecycle management. Procedia CIRP. 2019;84:545–9. doi:10.1016/j.procir.2019.04.176. [Google Scholar] [CrossRef]
25. Ariyachandra MRMF, Samarakkody A, Perera BAKS. Real-virtual synchronisation: a review on the state-of-the-art geometric digital twinning of infrastructure. In: Proceedings of the 8th World Construction Symposium, 2019; Colombo, Sri Lanka. p. 502–12. [Google Scholar]
26. Callcut M, Cerceau Agliozzo JP, Varga L, McMillan L. Digital twins in civil infrastructure systems. Sustainability. 2021;13(20):11549. doi:10.3390/su132011549. [Google Scholar] [CrossRef]
27. Alibrandi U. Risk-informed digital twin of buildings and infrastructures for sustainable and resilient urban communities. ASCE-ASME J Risk Uncertain Eng Syst, Part A: Civil Eng. 2022;8(3):04022032. doi:10.1061/ajrua6.0001238. [Google Scholar] [CrossRef]
28. Naderi H, Shojaei A. Civil infrastructure digital twins: multi-level knowledge map, research gaps, and future directions. IEEE Access. 2022;10:122022–37. doi:10.1109/access.2022.3223557. [Google Scholar] [CrossRef]
29. Kaewunruen S, Peng SJ, Phil-Ebosie O. Digital twin aided sustainability and vulnerability audit for subway stations. Sustainability. 2020;12(19):7873. doi:10.3390/su12197873. [Google Scholar] [CrossRef]
30. Chiachío M, Megía M, Chiachío J, Fernandez J, Jalón ML. Structural digital twin framework: formulation and technology integration. Autom Constr. 2022;140(2):104333. doi:10.1016/j.autcon.2022.104333. [Google Scholar] [CrossRef]
31. Wynne Z, Stratford T, Reynolds TPS. Perceptions of long-term monitoring for civil and structural engineering. Struct. 2022;41(3):1616–23. doi:10.1016/j.istruc.2022.05.090. [Google Scholar] [CrossRef]
32. Wernerová E, Endel S, Kutá D. Implementation of the BIM method at the VŠB—Technical University of Ostrava. Int J Eng Res Afr. 2020;47:133–8. doi:10.4028/www.scientific.net/JERA.47.133. [Google Scholar] [CrossRef]
33. Boje C, Guerriero A, Kubicki S, Rezgui Y. Towards a semantic construction digital twin: directions for future research. Autom Constr. 2020;114:103179. doi:10.1016/j.autcon.2020.103179. [Google Scholar] [CrossRef]
34. Peng Y, Zhang M, Yu F, Xu J, Gao S, Rodrigues H. Digital twin hospital buildings: an exemplary case study through continuous lifecycle integration. Adv Civil Eng. 2020;2020:1–13. doi:10.1155/2020/8846667. [Google Scholar] [CrossRef]
35. Desogus G, Quaquero E, Rubiu G, Gatto G, Perra C. BIM and IoT sensors integration: a framework for consumption and indoor conditions data monitoring of existing buildings. Sustainability. 2021;13(8):4496. doi:10.3390/su13084496. [Google Scholar] [CrossRef]
36. Opoku D-GJ, Perera S, Osei-Kyei R, Rashidi M. Digital twin application in the construction industry: a literature review. J Build Eng. 2021;40(3):102726. doi:10.1016/j.jobe.2021.102726. [Google Scholar] [CrossRef]
37. Zhao Y, Wang N, Liu Z, Mu E. Construction theory for a building intelligent operation and maintenance system based on digital twins and machine learning. Buildings. 2022;12(2):87. doi:10.3390/buildings12020087. [Google Scholar] [CrossRef]
38. Hosamo HH, Nielsen HK, Alnmr AN, Svennevig PR, Svidt K. A review of the digital twin technology for fault detection in buildings. Front Built Environ. 2022;8:1013196. doi:10.3389/fbuil.2022.1013196. [Google Scholar] [CrossRef]
39. Azfar T, Weidner J, Raheem A, Ke R, Cheu RL. Efficient procedure of building university campus models for digital twin simulation. IEEE J Radio Freq Identification. 2022;6:769–73. doi:10.1109/jrfid.2022.3212957. [Google Scholar] [CrossRef]
40. Huang H, Zeng X, Zhao L, Qiu C, Wu H, Fan L. Fusion of building information modeling and blockchain for metaverse: a survey. IEEE Open J Comput Soc. 2022;3(12):195–207. doi:10.1109/ojcs.2022.3206494. [Google Scholar] [CrossRef]
41. Sepasgozar SME, Khan AA, Smith K, Romero JG, Shen X, Shirowzhan S, et al. BIM and digital twin for developing convergence technologies as future of digital construction. Buildings. 2023;13(2):441. doi:10.3390/buildings13020441. [Google Scholar] [CrossRef]
42. Ni Z, Liu Y, Karlsson M, Gong S. Enabling preventive conservation of historic buildings through cloud-based digital twins: a case study in the city theatre, norrköping. IEEE Access. 2022;10:90924–39. doi:10.1109/access.2022.3202181. [Google Scholar] [CrossRef]
43. Vuoto A, Funari MF, Lourenço PB. On the use of the digital twin concept for the structural integrity protection of architectural heritage. Infrastructures. 2023;8(5):86. doi:10.3390/infrastructures8050086. [Google Scholar] [CrossRef]
44. Xu J, Shu X, Qiao P, Li S, Xu J. Developing a digital twin model for monitoring building structural health by combining a building information model and a real-scene 3D model. Measurement. 2023;217(104149):112955. doi:10.1016/j.measurement.2023.112955. [Google Scholar] [CrossRef]
45. Ding Y, Zhang Y, Huang X. Intelligent emergency digital twin system for monitoring building fire evacuation. J Build Eng. 2023;77:107416. doi:10.1016/j.jobe.2023.107416. [Google Scholar] [CrossRef]
46. Haag S, Anderl R. Digital twin—proof of concept. Manuf Lett. 2018;15(3):64–6. doi:10.1016/j.mfglet.2018.02.006. [Google Scholar] [CrossRef]
47. Corrêa F, Maciel AR. A Methodology for the development of interoperable BIM-based cyber-physical systems. In: Proceedings of the 35th International Symposium on Automation and Robotics in Construction (ISARC2018; Berlin, Germany. [Google Scholar]
48. Zhao Y, Cao C, Liu Z. A framework for prefabricated component hoisting management systems based on digital twin technology. Buildings. 2022;12(3):276. doi:10.3390/buildings12030276. [Google Scholar] [CrossRef]
49. Feng LY, Qian XD. Enhanced real-time crack monitoring and updating in welded structural components with limited measurement data. Mech Syst Signal Process. 2023;198(2):110403. doi:10.1016/j.ymssp.2023.110403. [Google Scholar] [CrossRef]
50. Cheok EWW, Qian XD, Chen C, Quek ST, Si MBI. A local digital twin approach for identifying, locating and sizing cracks in CHS X-joints subjected to brace axial loading. Eng Struct. 2024;299(5):117085. doi:10.1016/j.engstruct.2023.117085. [Google Scholar] [CrossRef]
51. Ding K, Shi H, Hui J, Liu Y, Zhu B, Zhang F, et al. Smart steel bridge construction enabled by BIM and internet of things in Industry 4.0: a framework. In: 15th IEEE International Conference on Networking, Sensing and Control (ICNSC2018; Zhuhai, China. [Google Scholar]
52. Ye C, Butler L, Calka B, Iangurazov M, Lu Q, Gregory A, et al. A digital twin of bridges for structural health monitoring. In: 12th International Workshop on Structural Health Monitoring, 2019; Stanford, USA. [Google Scholar]
53. Lu R, Brilakis I. Digital twinning of existing reinforced concrete bridges from labelled point clusters. Autom Constr. 2019;105(2):102837. doi:10.1016/j.autcon.2019.102837. [Google Scholar] [CrossRef]
54. Rojas-Mercedes N, Erazo K, di Sarno LJE. Seismic fragility curves for a concrete bridge using structural health monitoring and digital twins. Earthq Struct. 2022;22(5):503–15. [Google Scholar]
55. Nicoletti V, Martini R, Carbonari S, Gara F. Operational modal analysis as a support for the development of digital twin models of bridges. Infrastructures. 2023;8(2):24. doi:10.3390/infrastructures8020024. [Google Scholar] [CrossRef]
56. Hu K, Han D, Qin G, Zhou Y, Chen L, Ying C, et al. Semi-automated generation of geometric digital twin for bridge based on terrestrial laser scanning data. Adv Civil Eng. 2023;2023(2):1–13. doi:10.1155/2023/6192001. [Google Scholar] [CrossRef]
57. Mohammadi M, Rashidi M, Yu Y, Samali B. Integration of TLS-derived bridge information modeling (BrIM) with a decision support system (DSS) for digital twinning and asset management of bridge infrastructures. Comput Ind. 2023;147:103881. doi:10.1016/j.compind.2023.103881. [Google Scholar] [CrossRef]
58. Li J, Li Q, Mao Q, Xu H. Digital-twin-based high-precision assembly of a steel bridge tower. Buildings. 2023;13(1):257. doi:10.3390/buildings13010257. [Google Scholar] [CrossRef]
59. Kong X, Huck RG. Preserving our heritage: a photogrammetry-based digital twin framework for monitoring deteriorations of historic structures. Autom Constr. 2023;152(25):104928. doi:10.1016/j.autcon.2023.104928. [Google Scholar] [CrossRef]
60. Guo XY, Fang SE. Digital twin based lifecycle modeling and state evaluation of cable-stayed bridges. Eng Comput. 2023;34:1–15. doi:10.1007/s00366-023-01835-6. [Google Scholar] [CrossRef]
61. Lin K, Xu YL, Lu X, Guan Z, Li J. Digital twin-based collapse fragility assessment of a long-span cable-stayed bridge under strong earthquakes. Autom Constr. 2021;123(2):103547. doi:10.1016/j.autcon.2020.103547. [Google Scholar] [CrossRef]
62. Lin K, Xu YL, Lu X, Guan Z, Li J. Digital twin-based life-cycle seismic performance assessment of a long-span cable-stayed bridge. Bull Earthq Eng. 2023;21(2):1203–27. doi:10.1007/s10518-022-01567-w. [Google Scholar] [CrossRef]
63. Dan D, Ying Y, Ge L. Digital twin system of bridges group based on machine vision fusion monitoring of bridge traffic load. IEEE Trans Intell Transp Syst. 2022;23(11):22190–22205. doi:10.1109/tits.2021.3130025. [Google Scholar] [CrossRef]
64. Hosamo HH, Hosamo MH. Digital twin technology for bridge maintenance using 3D laser scanning: a review. Adv Civil Eng. 2022;2022:1–15. doi:10.1155/2022/2194949. [Google Scholar] [CrossRef]
65. Hu G, Zhou Y, Xiang Z, Zhao L, Chen G, Li T, et al. Fast and accurate generation method of geometric digital twin model of RC bridge with box chambers based on terrestrial laser scanning. Remote Sens. 2023;15(18):4440. doi:10.3390/rs15184440. [Google Scholar] [CrossRef]
66. Nhamage IAA, Dang NS, Horas CS, Martins JP, Matos JA, Calcada R. Performing Fatigue state characterization in railway steel bridges using digital twin models. Appl Sci. 2023;13(11):6741. doi:10.3390/app13116741. [Google Scholar] [CrossRef]
67. Jasinski M, Lazinski P, Piotrowski D. The concept of creating digital twins of bridges using load tests. Sensors. 2023;23(17):7349. doi:10.3390/s23177349. [Google Scholar] [PubMed] [CrossRef]
68. Song H, Yang G, Li H, Zhang T, Jiang A. Digital twin enhanced BIM to shape full life cycle digital transformation for bridge engineering. Autom Constr. 2023;147(4):104736. doi:10.1016/j.autcon.2022.104736. [Google Scholar] [CrossRef]
69. Wu J, Zhu J, Zhang J, Dang P, Li W, Guo Y, et al. A dynamic holographic modelling method of digital twin scenes for bridge construction. Int J Digit Earth. 2023;16(1):2404–25. doi:10.1080/17538947.2023.2229792. [Google Scholar] [CrossRef]
70. Chacón Flores RA, Sánchez Juny M, Real Saladrigas E, Gironella Cobos X, Puigagut Juárez J, Ledesma Villalba A. Digital twins in civil and environmental engineering classrooms. In: EUCEET 2018: 4th International Conference on Civil Engineering Education: Challenges for the Third Millennium, 2018; Barcelona, Spain. [Google Scholar]
71. Wahbeh W, Kunz D, Hofmann J, Bereuter P. Digital twinning of the built environment—an interdisciplinary topic for innovation in didactics. ISPRS Annal Photogramm Remote Sens Spat Inf Sci. 2020;4:231–7. doi:10.5194/isprs-annals-V-4-2020-231-2020. [Google Scholar] [CrossRef]
72. Chacón R. Designing Construction 4.0 activities for AEC classrooms. Buildings. 2021;11(11):511. doi:10.3390/buildings11110511. [Google Scholar] [CrossRef]
73. Kim J, Kim SA. Lifespan prediction technique for digital twin-based noise barrier tunnels. Sustainability. 2020;12(7):2940. doi:10.3390/su12072940. [Google Scholar] [CrossRef]
74. Lee J, Lee Y, Hong C. Development of geospatial data acquisition, modeling, and service technology for digital twin implementation of underground utility tunnel. Appl Sci. 2023;13(7):4343. doi:10.3390/app13074343. [Google Scholar] [CrossRef]
75. Park S, Hong C, Hwang I, Lee J. Comparison of single-camera-based depth estimation technology for digital twin model synchronization of underground utility tunnels. Appl Sci. 2023;13(4):2106. doi:10.3390/app13042106. [Google Scholar] [CrossRef]
76. Ye Z, Ye Y, Zhang C, Zhang Z, Li W, Wang X, et al. A digital twin approach for tunnel construction safety early warning and management. Comput Ind. 2023;144:103783. doi:10.1016/j.compind.2022.103783. [Google Scholar] [CrossRef]
77. Zhao Y, Wang N, Liu Z. An established theory of digital twin model for tunnel construction safety assessment. Appl Sci. 2022;12(23):12256. doi:10.3390/app122312256. [Google Scholar] [CrossRef]
78. Wu Z, Chang Y, Li Q, Cai R. A novel method for tunnel digital twin construction and virtual-real fusion application. Electronics. 2022;11(9):1413. doi:10.3390/electronics11091413. [Google Scholar] [CrossRef]
79. Latif K, Sharafat A, Seo J. Digital twin-driven framework for TBM performance prediction, visualization, and monitoring through machine learning. Appl Sci. 2023;13(20):11435. doi:10.3390/app132011435. [Google Scholar] [CrossRef]
80. Tita EE, Watanabe G, Shao P, Arii K. Development and application of digital twin-BIM technology for bridge management. Appl Sci. 2023;13(13):7435. doi:10.3390/app13137435. [Google Scholar] [CrossRef]
81. Grosse CU. Monitoring and inspection techniques supporting a digital twin concept in civil engineering. In: Proceedings of the 5th International Conference on Sustainable Construction Materials and Technologies (SCMT52019; London, UK. [Google Scholar]
82. Sepasgozar SME, Karimi R, Shirowzhan S, Mojtahedi M, Ebrahimzadeh S, McCarthy D. Delay causes and emerging digital tools: a novel model of delay analysis, including integrated project delivery and PMBOK. Buildings. 2019;9(9):191. doi:10.3390/buildings9090191. [Google Scholar] [CrossRef]
83. de Amicis R, Bernstein WZ, Scholz J, Radkowski R, Simões B, Lieberman J, et al. Merging geospatial technologies with cross reality in the context of smart manufacturing systems. In: IEEE International Symposium on Mixed and Augmented Reality Adjunct (ISMAR-Adjunct2020; Recife, Brazil. [Google Scholar]
84. Vrana J. The core of the fourth revolutions: industrial internet of things, digital twin, and cyber-physical loops. J Nondestruct. Eval. 2021;40(2):1–34. doi:10.1007/s10921-021-00777-7. [Google Scholar] [CrossRef]
85. Jiang F, Ma L, Broyd T, Chen K. Digital twin and its implementations in the civil engineering sector. Autom Constr. 2021;130(2):103838. doi:10.1016/j.autcon.2021.103838. [Google Scholar] [CrossRef]
86. Meža S, Mauko Pranjić A, Vezočnik R, Osmokrović I, Lenart S, Biancardo SA. Digital twins and road construction using secondary raw materials. J Adv Transport. 2021;2021(1):1–12. doi:10.1155/2021/8833058. [Google Scholar] [CrossRef]
87. Vieira J, Poças Martins J, Marques de Almeida N, Patrício H, Gomes Morgado J. Towards resilient and sustainable rail and road networks: a systematic literature review on digital twins. Sustainability. 2022;14(12):7060. doi:10.3390/su14127060. [Google Scholar] [CrossRef]
88. Wei Y, Lei Z, Altaf S. An off-site construction digital twin assessment framework using wood panelized construction as a case study. Buildings. 2022;12(5):566. doi:10.3390/buildings12050566. [Google Scholar] [CrossRef]
89. Rathore MM, Shah SA, Shukla D, Bentafat E, Bakiras S. The role of AI, machine learning, and big data in digital twinning: a systematic literature review, challenges, and opportunities. IEEE Access. 2021;9:32030–52. doi:10.1109/access.2021.3060863. [Google Scholar] [CrossRef]
90. Hribernik KA, Rabe L, Schumacher J, Thoben KD. The product avatar as a product-instance-centric information management concept. Int Conf Prod Life Cycle Manag. 2006;1(4):367–79. [Google Scholar]
91. Liu Q, Liu B, Wang G, Zhang C. A comparative study on digital twin models. In: 3rd International Conference on Advances in Materials, Machinery, Electronics (AMME2019; Wuhan, China. [Google Scholar]
92. Roh S, Aziz Z, Pena-Mora F. An object-based 3D walk-through model for interior construction progress monitoring. Autom Constr. 2011;20(1):66–75. doi:10.1016/j.autcon.2010.07.003. [Google Scholar] [CrossRef]
Cite This Article
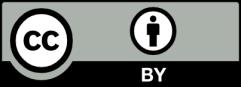