Open Access
ARTICLE
Rapid and Accurate Identification of Concrete Surface Cracks via a Lightweight & Efficient YOLOv3 Algorithm
1 College of Mechanics and Engineering Science, Hohai University, Nanjing, 211100, China
2 Department of Civil Engineering and Natural Hazards, University of Natural Resources and Life Sciences, Vienna, 1180, Austria
3 The First Geological Brigade of the Bureau of Geology and Mineral Resources of Jiangsu, Nanjing, 210041, China
4 Control Technology Group Co., Nanjing, 210041, China
5 Department of Technical Sciences, Civil Engineering, State University of Novi Pazar, Novi Pazar, 36300, Serbia
* Corresponding Author: Maosen Cao. Email:
Structural Durability & Health Monitoring 2024, 18(4), 363-380. https://doi.org/10.32604/sdhm.2024.042388
Received 28 May 2023; Accepted 01 November 2023; Issue published 05 June 2024
Abstract
Concrete materials and structures are extensively used in transformation infrastructure and they usually bear cracks during their long-term operation. Detecting cracks using deep-learning algorithms like YOLOv3 (You Only Look Once version 3) is a new trend to pursue intelligent detection of concrete surface cracks. YOLOv3 is a typical deep-learning algorithm used for object detection. Owing to its generality, YOLOv3 lacks specific efficiency and accuracy in identifying concrete surface cracks. An improved algorithm based on YOLOv3, specialized in the rapid and accurate identification of concrete surface cracks is worthy of investigation. This study proposes a tailored deep-learning algorithm, termed MDN-YOLOv3 (MDN: multi-dilated network), of which the MDN is formulated based on three retrofit techniques, and it provides a new backbone network for YOLOv3. The three specific retrofit techniques are briefed: (i) Depthwise separable convolution is utilized to reduce the size of the backbone network; (ii) The dilated-down sampling structure is proposed and used in the backbone network to achieve multi-scale feature fusion; and (iii) The convolutional block attention module is introduced to enhance feature extraction ability. Results show that the proposed MDN-YOLOv3 is 97.2% smaller and 41.5% faster than YOLOv3 in identifying concrete surface cracks, forming a lightweight & efficient YOLOv3 algorithm for intelligently identifying concrete surface cracks.Keywords
Cite This Article
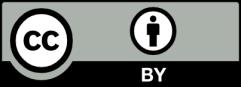
This work is licensed under a Creative Commons Attribution 4.0 International License , which permits unrestricted use, distribution, and reproduction in any medium, provided the original work is properly cited.