Open Access
ARTICLE
Intelligent Fault Diagnosis Method of Rolling Bearings Based on Transfer Residual Swin Transformer with Shifted Windows
1 The Institute of Marine Science and Technology, Shandong University, Qingdao, 26623, China
2 The School of Control Sciences and Engineering, Shandong University, Jinan, 250061, China
3 Thailand Institute of Scientific and Technological Research, Amphoe Khlong Luang, Pathum Thani, 12120, Thailand
* Corresponding Author: Qingmei Sui. Email:
Structural Durability & Health Monitoring 2024, 18(2), 91-110. https://doi.org/10.32604/sdhm.2023.041522
Received 26 April 2023; Accepted 02 August 2023; Issue published 22 March 2024
Abstract
Due to their robust learning and expression ability for complex features, the deep learning (DL) model plays a vital role in bearing fault diagnosis. However, since there are fewer labeled samples in fault diagnosis, the depth of DL models in fault diagnosis is generally shallower than that of DL models in other fields, which limits the diagnostic performance. To solve this problem, a novel transfer residual Swin Transformer (RST) is proposed for rolling bearings in this paper. RST has 24 residual self-attention layers, which use the hierarchical design and the shifted window-based residual self-attention. Combined with transfer learning techniques, the transfer RST model uses pre-trained parameters from ImageNet. A new end-to-end method for fault diagnosis based on deep transfer RST is proposed. Firstly, wavelet transform transforms the vibration signal into a wavelet time-frequency diagram. The signal’s time-frequency domain representation can be represented simultaneously. Secondly, the wavelet time-frequency diagram is the input of the RST model to obtain the fault type. Finally, our method is verified on public and self-built datasets. Experimental results show the superior performance of our method by comparing it with a shallow neural network.Keywords
Cite This Article
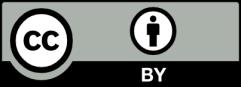
This work is licensed under a Creative Commons Attribution 4.0 International License , which permits unrestricted use, distribution, and reproduction in any medium, provided the original work is properly cited.