Open Access
ARTICLE
Predicting Reliability and Remaining Useful Life of Rolling Bearings Based on Optimized Neural Networks
School of Automation and Electrical Engineering, Dalian Jiaotong University, Dalian, 116028, China
* Corresponding Author: Tiantian Liang. Email:
Structural Durability & Health Monitoring 2023, 17(5), 433-455. https://doi.org/10.32604/sdhm.2023.029331
Received 13 February 2023; Accepted 13 April 2023; Issue published 07 September 2023
Abstract
In this study, an optimized long short-term memory (LSTM) network is proposed to predict the reliability and remaining useful life (RUL) of rolling bearings based on an improved whale-optimized algorithm (IWOA). The multi-domain features are extracted to construct the feature dataset because the single-domain features are difficult to characterize the performance degeneration of the rolling bearing. To provide covariates for reliability assessment, a kernel principal component analysis is used to reduce the dimensionality of the features. A Weibull distribution proportional hazard model (WPHM) is used for the reliability assessment of rolling bearing, and a beluga whale optimization (BWO) algorithm is combined with maximum likelihood estimation (MLE) to improve the estimation accuracy of the model parameters of the WPHM, which provides the data basis for predicting reliability. Considering the possible gradient explosion by training the rolling bearing lifetime data and the difficulties in selecting the key network parameters, an optimized LSTM network called the improved whale optimization algorithm-based long short-term memory (IWOA-LSTM) network is proposed. As IWOA better jumps out of the local optimization, the fitting and prediction accuracies of the network are correspondingly improved. The experimental results show that compared with the whale optimization algorithm-based long short-term memory (WOA-LSTM) network, the reliability prediction and RUL prediction accuracies of the rolling bearing are improved by the proposed IWOA-LSTM network.Graphic Abstract
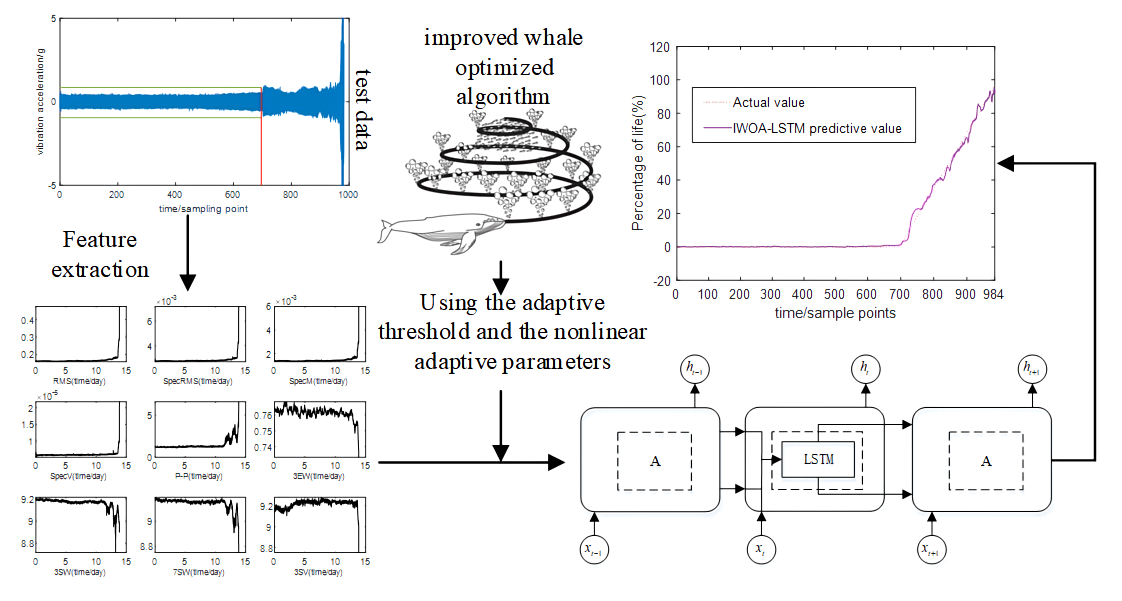
Keywords
Cite This Article
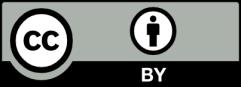
This work is licensed under a Creative Commons Attribution 4.0 International License , which permits unrestricted use, distribution, and reproduction in any medium, provided the original work is properly cited.