Open Access
ARTICLE
Outlier Detection and Forecasting for Bridge Health Monitoring Based on Time Series Intervention Analysis
College of Civil Engineering, Putian University, Putian, 351100, China
* Corresponding Author: Bing Qu. Email:
Structural Durability & Health Monitoring 2022, 16(4), 323-341. https://doi.org/10.32604/sdhm.2022.021446
Received 14 January 2022; Accepted 18 July 2022; Issue published 03 January 2023
Abstract
The method of time series analysis, applied by establishing appropriate mathematical models for bridge health monitoring data and making forecasts of structural future behavior, stands out as a novel and viable research direction for bridge state assessment. However, outliers inevitably exist in the monitoring data due to various interventions, which reduce the precision of model fitting and affect the forecasting results. Therefore, the identification of outliers is crucial for the accurate interpretation of the monitoring data. In this study, a time series model combined with outlier information for bridge health monitoring is established using intervention analysis theory, and the forecasting of the structural responses is carried out. There are three techniques that we focus on: (1) the modeling of seasonal autoregressive integrated moving average (SARIMA) model; (2) the methodology for outlier identification and amendment under the circumstances that the occurrence time and type of outliers are known and unknown; (3) forecasting of the model with outlier effects. The method was tested with a case study using monitoring data on a real bridge. The establishment of the original SARIMA model without considering outliers is first discussed, including the stationarity, order determination, parameter estimation and diagnostic checking of the model. Then the time-by-time iterative procedure for outlier detection, which is implemented by appropriate test statistics of the residuals, is performed. The SARIMA-outlier model is subsequently built. Finally, a comparative analysis of the forecasting performance between the original model and SARIMA-outlier model is carried out. The results demonstrate that proper time series models are effective in mining the characteristic law of bridge monitoring data. When the influence of outliers is taken into account, the fitted precision of the model is significantly improved and the accuracy and the reliability of the forecast are strengthened.Keywords
Cite This Article
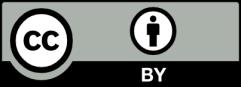
This work is licensed under a Creative Commons Attribution 4.0 International License , which permits unrestricted use, distribution, and reproduction in any medium, provided the original work is properly cited.