Open Access
ARTICLE
Comparative Study on Tool Fault Diagnosis Methods Using Vibration Signals and Cutting Force Signals by Machine Learning Technique
1 National Institute of Technology Karnataka, Mangaluru, 575 025, India
2 Vellore Institute of Technology University, Chennai, 600 127, India
* Corresponding Author: Hemantha Kumar. Email:
Structural Durability & Health Monitoring 2020, 14(2), 127-145. https://doi.org/10.32604/sdhm.2020.07595
Received 10 June 2019; Accepted 15 July 2019; Issue published 23 June 2020
Abstract
The state of cutting tool determines the quality of surface produced on the machined parts. A faulty tool produces poor surface, inaccurate geometry and non-economic production. Thus, it is necessary to monitor tool condition for a machining process to have superior quality and economic production. In the present study, fault classification of single point cutting tool for hard turning has been carried out by employing machine learning technique. Cutting force and vibration signals were acquired to monitor tool condition during machining. A set of four tooling conditions namely healthy, worn flank, broken insert and extended tool overhang have been considered for the study. The machine learning technique was applied to both vibration and cutting force signals. Discrete wavelet features of the signals have been extracted using discrete wavelet transformation (DWT). This transformation represents a large dataset into approximation coefficients which contain the most useful information of the dataset. Significant features, among features extracted, were selected using J48 decision tree technique. Classification of tool conditions was carried out using Naïve Bayes algorithm. A 10 fold cross validation was incorporated to test the validity of classifier. A comparison of performance of classifier was made between cutting force and vibration signal to choose the best signal acquisition method in classifying tool fault conditions using machine learning technique.Keywords
Cite This Article
Citations
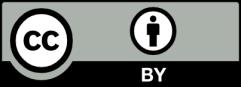