Open Access
ARTICLE
Ensemble Recurrent Neural Network-Based Residual Useful Life Prognostics of Aircraft Engines
School of Naval Architecture and Ocean Engineering, Huazhong University of Science and Technology, Wuhan, 430074, China. 1School of Naval Architecture and Ocean Engineering, Huazhong University of Science and Technology, Wuhan, 430074, China.
School of Mechanical Science and Engineering, Huazhong University of Science and Technology, Wuhan, 430074, China.
China Electronic Product Reliability and Environmental Testing Research Institute, Guangzhou, 510610, China.
*Corresponding Author: Jun Wu. Email: ;
.
Structural Durability & Health Monitoring 2019, 13(3), 317-329. https://doi.org/10.32604/sdhm.2019.05571
Abstract
Residual useful life (RUL) prediction is a key issue for improving efficiency of aircraft engines and reducing their maintenance cost. Owing to various failure mechanism and operating environment, the application of classical models in RUL prediction of aircraft engines is fairly difficult. In this study, a novel RUL prognostics method based on using ensemble recurrent neural network to process massive sensor data is proposed. First of all, sensor data obtained from the aircraft engines are preprocessed to eliminate singular values, reduce random fluctuation and preserve degradation trend of the raw sensor data. Secondly, three kinds of recurrent neural networks (RNN), including ordinary RNN, long short-term memory (LSTM), and gated recurrent unit (GRU), are individually constructed. Thirdly, ensemble learning mechanism is designed to merge the above RNNs for producing a more accurate RUL prediction. The effectiveness of the proposed method is validated using two characteristically different turbofan engine datasets. Experimental results show a competitive performance of the proposed method in comparison with typical methods reported in literatures.Keywords
Cite This Article
Citations
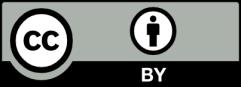
This work is licensed under a Creative Commons Attribution 4.0 International License , which permits unrestricted use, distribution, and reproduction in any medium, provided the original work is properly cited.