Open Access
ARTICLE
A Comparative Study of Bayes Classifiers for Blade Fault Diagnosis in Wind Turbines through Vibration Signals
Research Associate, School of Mechanical and Building Sciences (SMBS), VIT University, Chennai
Campus, Vandalur-Kelambakkam Road, Chennai 600127, India. E-mail: joshuva1991@gmail.com.
Associate Professor, School of Mechanical and Building Sciences (SMBS), VIT University, Chennai
Campus, Vandalur-Kelambakkam Road, Chennai 600127, India. E-mail: v_sugu@yahoo.com.
Structural Durability & Health Monitoring 2017, 11(1), 69-90. https://doi.org/10.3970/sdhm.2017.012.069
Abstract
Renewable energy sources are considered much in energy fields because of the contemporary energy calamities. Among the important alternatives being considered, wind energy is a durable competitor because of its dependability due to the development of the innovations, comparative cost effectiveness and great framework. To yield wind energy more proficiently, the structure of wind turbines has turned out to be substantially bigger, creating conservation and renovation works troublesome. Due to various ecological conditions, wind turbine blades are subjected to vibration and it leads to failure. If the failure is not diagnosed early, it will lead to catastrophic damage to the framework. In order to increase safety observations, to reduce down time, to bring down the recurrence of unexpected breakdowns and related enormous maintenance, logistic expenditures and to contribute steady power generation, the wind turbine blade must be monitored now and then to assure that they are in good condition. In this paper, a three bladed wind turbine was preferred and using vibration source, the condition of a wind turbine blade is examined. The faults like blade crack, erosion, hub-blade loose connection, pitch angle twist and blade bend faults were considered and these faults are classified using Bayes Net (BN), Discriminative Multinomial Naïve Bayes (DMNB), Naïve Bayes (NB), Simple Naïve Bayes (SNB), and Updateable Naïve Bayes (UNB) classifiers. These classifiers are compared and better classifier is suggested for condition monitoring of wind turbine blades.Keywords
Cite This Article
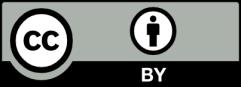
This work is licensed under a Creative Commons Attribution 4.0 International License , which permits unrestricted use, distribution, and reproduction in any medium, provided the original work is properly cited.