Open Access
ARTICLE
Data-Driven Method for Predicting Remaining Useful Life of Bearings Based on Multi-Layer Perception Neural Network and Bidirectional Long Short-Term Memory Network
1 CRRC Qingdao Sifang Co., Ltd., Qingdao, 266111, China
2 School of Control Science and Engineering, Shandong University, Jinan, 250061, China
3 Public (Innovation) Experimental Teaching Center, Shangdong University, Qingdao, 266237, China
4 Engineering Training Center, Shangdong University, Jinan, 250061, China
* Corresponding Author: Faye Zhang. Email:
(This article belongs to the Special Issue: Health Monitoring and Rapid Evaluation of Infrastructures)
Structural Durability & Health Monitoring 2025, 19(2), 365-383. https://doi.org/10.32604/sdhm.2024.053998
Received 15 May 2024; Accepted 31 July 2024; Issue published 15 January 2025
Abstract
The remaining useful life prediction of rolling bearing is vital in safety and reliability guarantee. In engineering scenarios, only a small amount of bearing performance degradation data can be obtained through accelerated life testing. In the absence of lifetime data, the hidden long-term correlation between performance degradation data is challenging to mine effectively, which is the main factor that restricts the prediction precision and engineering application of the residual life prediction method. To address this problem, a novel method based on the multi-layer perception neural network and bidirectional long short-term memory network is proposed. Firstly, a nonlinear health indicator (HI) calculation method based on kernel principal component analysis (KPCA) and exponential weighted moving average (EWMA) is designed. Then, using the raw vibration data and HI, a multi-layer perceptron (MLP) neural network is trained to further calculate the HI of the online bearing in real time. Furthermore, The bidirectional long short-term memory model (BiLSTM) optimized by particle swarm optimization (PSO) is used to mine the time series features of HI and predict the remaining service life. Performance verification experiments and comparative experiments are carried out on the XJTU-SY bearing open dataset. The research results indicate that this method has an excellent ability to predict future HI and remaining life.Keywords
Cite This Article
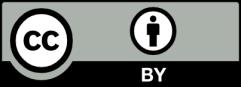
This work is licensed under a Creative Commons Attribution 4.0 International License , which permits unrestricted use, distribution, and reproduction in any medium, provided the original work is properly cited.