Open Access
ARTICLE
SteelGuard-yolo: Steel surface defect detection network based on improved YOLOv5s
1 Lanzhou University, Lanzhou, Gansu 730000, People’s Republic of China
* Corresponding Authors: Zheng Zhou (), Min Yuan (
)
Revista Internacional de Métodos Numéricos para Cálculo y Diseño en Ingeniería 2024, 40(2), 1-7. https://doi.org/10.23967/j.rimni.2024.05.011
Received 15 May 2024; Accepted 25 May 2024; Issue published 10 June 2024
Abstract
Steel is playing an increasingly important role in industry, and the detection of defects on its surface is also of great significance. The complex and diverse defects on the steel surface bring great challenges to the detection. In this paper, we propose a SteelGuard-yolo based steel surface defect detection model, whose main role is to improve the existing algorithms for detecting steel surface defects. First, we design the C2f module with weight aggregation and introduce the BiFormer attention mechanism to improve the feature extraction capability of the model. Second, we design a new up-sampling structure and introduce the Multi-Scale Dilated Attention (MSDA) module to effectively improve the feature fusion capability of the model. Finally, we introduce the Simam attention mechanism and use EIoU as a new loss function to improve the robustness and accuracy of the model.SteelGuard-yolo has a powerful multi-scale feature fusion capability and achieves an ideal balance between latency and accuracy. The algorithm proposed in this paper is tested on the NEU-DET dataset and achieves an average accuracy of 69.0%, which compares favorably with most one- and two-stage detection algorithms.Keywords
Cite This Article
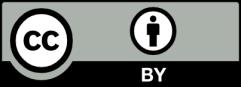
This work is licensed under a Creative Commons Attribution 4.0 International License , which permits unrestricted use, distribution, and reproduction in any medium, provided the original work is properly cited.