Open Access
ARTICLE
A empirical research on AI-powered athletic posture detection in sports training
1 Xikyo College Physical Education Center Xi'an. 710123, China
2 Department of Public Studies, Xi'an Traffic Engineering University Xi'an, 710300, China
* Corresponding Author: Shunyong Wang ()
Revista Internacional de Métodos Numéricos para Cálculo y Diseño en Ingeniería 2024, 40(2), 1-6. https://doi.org/10.23967/j.rimni.2024.06.002
Received 27 May 2024; Accepted 12 June 2024; Issue published 26 June 2024
Abstract
The current investigation delineates the efficacy of AI-facilitated detection of athletic postures within the realm of sports training. Employing a synthesis of literature review and empirical methodologies, data were amassed and scrutinized, affirming the study’s validity. The salient outcomes are manifold: (1) The frame difference algorithm efficaciously discerns inter-frame variances, evidencing pronounced adaptability and robustness, thereby enabling the recognition of weightlifting postures. (2) Confronting the challenge of negligible inter-frame disparities inherent in the frame difference algorithm, the research introduces a novel detection technique predicated on the cumulative inter-frame differences, which precisely pinpoints regions of posture alteration in weightlifting athletes. (3) Leveraging the dynamic space model of optical flow, the study ascertains the directional channel predicated on optical flow trajectory analyses, facilitating the identification of three distinct weightlifting postures: squatting, descending, and standing. (4) In alignment with the distinctive postural attributes of weightlifting athletes, a human posture paradigm was formulated, and a BP neural network classifier was deployed for both training and evaluative purposes, culminating in the successful differentiation of athlete from non-athlete entities within the training milieu. (5) The application of AI in posture recognition was extended to the scrutiny of pivotal postures and motions in weightlifting athletes, with experimental findings revealing a 98.21% accuracy rate in the recognition of force-exertion postures via the inter-frame difference method, and a flawless 100% accuracy in the identification of the apex and squatting postures. The enumeration of detected postures—encompassing knee extension, knee flexion, force application, squatting, and standing—through the poselet keyframe extraction approach, corresponded with the video count. Prospectively, AI’s role in athletic posture detection promises to augment coaches’ and athletes’ comprehension of their proficiencies and deficiencies, thereby steering training refinement and bolstering both the efficacy of training and the athletes’ caliber.Keywords
Cite This Article
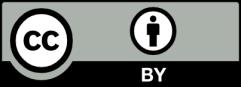
This work is licensed under a Creative Commons Attribution 4.0 International License , which permits unrestricted use, distribution, and reproduction in any medium, provided the original work is properly cited.