Open Access
ARTICLE
Early detection of breast cancer in mammograms using the lightweight modification of efficientNet B3
1 Department of Mathematical Sciences, Faculty of Science and Technology, Universiti Kebangsaan Malaysia, Selangor, Malaysia
2 Department of Mathematical Sciences, Faculty of Science & Technology, Universiti Kebangsaan Malaysia, 43600, Bangi, Selangor,
Malaysia
3 Department of Radiology, Kulliyyah of Medicine, International Islamic University Malaysia
4 Department of Fundamental Dental and Medical Sciences, Kulliyyah of Dentistry, International Islamic University Malaysia, Kuantan, Pahang 25200, Malaysia
5 Cluster of Cancer Research Initiative IIUM (COCRII), Kulliyyah of Dentistry, International Islamic University Malaysia, Kuantan,
Pahang 25200, Malaysia
* Corresponding Author: Saiful Izzuan Hussain ()
Revista Internacional de Métodos Numéricos para Cálculo y Diseño en Ingeniería 2023, 39(3), 1-7. https://doi.org/10.23967/j.rimni.2023.08.002
Accepted 25 July 2023; Issue published 01 September 2023
Abstract
Breast cancer is one of the leading causes of death in women worldwide and early detection is critical to improving survival rates. In this study, we present a modified deep learning method for automatic feature detection for breast mass classification on mammograms. We propose to use EfficientNet, a Convolutional Neural Network (CNN) architecture that requires minimal parameters. The main advantage of EfficientNet is the small number of parameters, which allows efficient and accurate classification of mammogram images. Our experiments show that EfficientNet, with an overall accuracy of 86.5 percent, has the potential to be the basis for a fully automated and effective breast cancer detection system in the future. Our results demonstrate the potential of EfficientNet to improve the accuracy and efficiency of breast cancer detection compared to other approaches.Keywords
Cite This Article
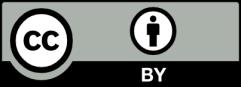
This work is licensed under a Creative Commons Attribution 4.0 International License , which permits unrestricted use, distribution, and reproduction in any medium, provided the original work is properly cited.