Open Access
ARTICLE
TDCMDA: Tripartite graph-based integrating dual-layer contrast learning into graph convolutional network for miRNA-disease association identification
1 College of Informatics, Huazhong Agricultural University, Wuhan 430070, China
* Corresponding Authors: Jing Bai (), Ping Zhang (
), Li Li (
)
Revista Internacional de Métodos Numéricos para Cálculo y Diseño en Ingeniería 2023, 39(3), 1-9. https://doi.org/10.23967/j.rimni.2023.08.001
Accepted 18 July 2023; Issue published 28 August 2023
Abstract
MicroRNAs (miRNAs) play essential roles in various biological regulatory processes and are closely related to the occurrence and development of complex diseases. Identifying miRNA-disease associations (MDA) is of great value for revealing the molecular mechanisms of diseases and exploring therapeutic strategies and drug development. Recently, most computer-aided MDAs identification approaches design their models tend to base on a bipartite graph (i.e., miRNA-disease network), ignoring the endogenous RNAs(ceRNAs) hypothesis in post-transcriptional control such as gene negative regulation by targeting mRNAs. Besides, the existing MDA bipartite graph could not make convincing predictions for MDA, only relying on collaborative filtering followed by the recommended system. To address the above issues, we propose a TDCMDA (Tripartite graph-based integrating Dual- layer Contrast learning into graph neural network for MDA prediction), which aims to integrate dual-layer contrast learning into graph neural network under the miRNA-disease-gene tripartite graph. Different from the existing approaches, TDCMDA introduces not only rich biologic regulatory relationships hidden in ceRNAs by a tripartite graph but also employs self-supervised dual-layer contrast learning to alleviate sparse label disadvantage. TDCMDA can learn node feature representation across three subgraph spaces such that the link representation between miRNA and disease can be obtained more biology semantically. Comprehensive experiments indicate TDCMDA is superior to several state-of-the-art approaches, and the case studies show that TDCMDA can convincingly detect novel MDA pairs and can be a promising tool for MDA identification.Keywords
Cite This Article
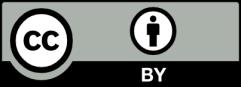
This work is licensed under a Creative Commons Attribution 4.0 International License , which permits unrestricted use, distribution, and reproduction in any medium, provided the original work is properly cited.