Open Access
ARTICLE
A deep learning-based prognostic framework for aeroengine exhaust gas temperature margin
1 Aviation Engineering Institute, Civil Aviation Flight University of China
2 School of Mechanical Engineering, Southwest Jiaotong University, Chengdu, China
3 Southwest Jiaotong University, Chengdu, China
4 Technology and Equipment of Rail Transit Operation and Maintenance Key Laboratory of Sichuan Province, Chengdu, China
* Corresponding Authors: Weigang Fu (" />" />" />,
" />" />" />), Xiang Tan (
" />" />" />,
" />" />" />), Liangzhong Ao (
" />" />" />,
" />" />" />), Yaoming Fu (
" />" />" />,
" />" />" />)
Revista Internacional de Métodos Numéricos para Cálculo y Diseño en Ingeniería 2023, 39(2), 1-9. https://doi.org/10.23967/j.rimni.2023.05.002
Accepted 08 May 2023; Issue published 16 May 2023
Abstract
The value of the gas-path parameter, exhaust gas temperature margin (EGTM), is the critical index for predicting aeroengine performance degradation. Accurate predictions help to improve engine maintenance, replacement schedules, and flight safety. The outside air temperature (OAT), altitude of the airport, the number of flight cycles, and water washing information were chosen as the sample input variables for the data-driven prognostic model for predicting the take-off EGTM of the on-wing engine. An attention-based deep learning framework was proposed for the aeroengine performance prediction model. Specifically, the multiscale convolutional neural network (CNN) structure is designed to initially learn sequential features from raw input data. Subsequently, the long short-term memory (LSTM) structure is employed to further extract the features processed by the multiscale CNN structure. Furthermore, the proposed attention mechanism is adopted to learn the influence of features and time steps, assigning different weights according to their importance. The actual operation data of the aeroengine are used to conduct experiments, where the experimental results verify the effectiveness of our proposed method in EGTM prediction.Cite This Article
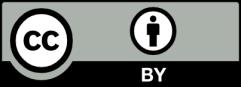
This work is licensed under a Creative Commons Attribution 4.0 International License , which permits unrestricted use, distribution, and reproduction in any medium, provided the original work is properly cited.