Open Access
ARTICLE
Optimization of titanium cranial implant designs using generalized reduced gradient method, analysis of finite elements, and artificial neural networks
1 Doctorado en Ciencias de la Ingeniería, Tecnológico Nacional de México en Celaya, Celaya, Guanajuato, Mexico
2 Laboratorio Nacional de Proyección Térmica (CENAPROT), Centro de Investigación y de Estudios Avanzados del IPN (CINVESTAV), Unidad Querétaro, Libramiento Norponiente #2000, Fraccionamiento Real de Juriquilla, 76230 Santiago de Querétaro, Mexico
3 Maestría en Innovación Aplicada, Tecnológico Nacional de México en Celaya, Celaya, Guanajuato, Mexico
4 Departamento de Ingeniería Industrial, Tecnológico Nacional de México en Celaya, Celaya, Guanajuato, Mexico
5 Departamento de Diseño, Universidad Autónoma de Ciudad Juárez, Ciudad Juárez, Chihuahua, Mexico
6 Doctorado en Ciencias de la Ingeniería, Tecnológico Nacional de México en Celaya, Celaya, Guanajuato, Mexico
Revista Internacional de Métodos Numéricos para Cálculo y Diseño en Ingeniería 2022, 38(2), 1-16. https://doi.org/10.23967/j.rimni.2022.06.004
Received 24 December 2021; Accepted 14 June 2022; Issue published 22 June 2022
Abstract
When cranial bone needs to be removed or lost, subsequent reconstruction of the defect is necessary to protect the underlying brain, correct aesthetic deformities, or both. Cranioplasty surgical procedures are performed to correct the skull defects requiring reconstruction of form and function. Personalized cranial implants can repair severe injuries to the skull can be done through This study presents the optimization of cranial titanium implants. A total of sixty different models were subjected to a simulation by Finite Element Analysis (FEA) applying the mechanical properties of a grade 5 titanium alloy (Ti6Al4V) implant material. The material was subjected to intracranial pressure (ICP) conditions, with a typical range (10 mm Hg) and twelve fixation points in the boundary conditions. An artificial neural network (ANN) was created to connect the designs, obtaining maximum displacements. Optimal designs were obtained using a generalized reduced gradient that minimizes the amount of material, maintaining as a restriction a maximum displacement of 0.1 mm for the 5th to 95t percentiles, which represent the group of individuals under study.Keywords
Cite This Article
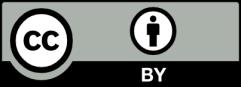
This work is licensed under a Creative Commons Attribution 4.0 International License , which permits unrestricted use, distribution, and reproduction in any medium, provided the original work is properly cited.