Open Access
ARTICLE
Combining QTL Mapping and Multi-Omics Identify Candidate Genes for Nutritional Quality Traits during Grain Filling Stage in Maize
1 Jiangsu Key Laboratory of Crop Genetics and Physiology, Key Laboratory of Plant Functional Genomics of the Ministry of Education, Jiangsu Key Laboratory of Crop Genomics and Molecular Breeding, Agricultural College of Yangzhou University, Yangzhou, 225009, China
2 Jiangsu Co-Innovation Center for Modern Production Technology of Grain Crops, Yangzhou University, Yangzhou, 225009, China
* Corresponding Authors: Zefeng Yang. Email: ; Chenwu Xu. Email:
Phyton-International Journal of Experimental Botany 2024, 93(7), 1441-1453. https://doi.org/10.32604/phyton.2024.052219
Received 26 March 2024; Accepted 24 June 2024; Issue published 30 July 2024
Abstract
The nutritional composition and overall quality of maize kernels are largely determined by the key chemical components: protein, oil, and starch. Nevertheless, the genetic basis underlying these nutritional quality traits during grain filling remains poorly understood. In this study, the concentrations of protein, oil, and starch were studied in 204 recombinant inbred lines resulting from a cross between DH1M and T877 at four different stages post-pollination. All the traits exhibited considerable phenotypic variation. During the grain-filling stage, the levels of protein and starch content generally increased, whereas oil content decreased, with significant changes observed between 30 and 40 days after pollination. Quantitative trait locus (QTL) mapping was conducted and a total of 32 QTLs, comprising 14, 12, and 6 QTLs for grain protein, oil, and starch content were detected, respectively. Few QTLs were consistently detectable across different time points. By integrating QTL analysis, global gene expression profiling, and comparative genomics, we identified 157, 86, and 54 differentially expressed genes harboring nonsynonymous substitutions between the parental lines for grain protein, oil, and starch content, respectively. Subsequent gene function annotation prioritized 15 candidate genes potentially involved in regulating grain quality traits, including those encoding transcription factors (NAC, MADS-box, bZIP, and MYB), cell wall invertase, cellulose-synthase-like protein, cell division cycle protein, trehalase, auxin-responsive factor, and phloem protein 2-A13. Our study offers significant insights into the genetic architecture of maize kernel nutritional quality and identifies promising QTLs and candidate genes, which are crucial for the genetic enhancement of these traits in maize breeding programs.Keywords
Supplementary Material
Supplementary Material FileMaize ranks among the world’s key food crops, providing around 5% of the global intake of dietary calories and proteins [1]. Additionally, it serves as a crucial component in animal feed and a valuable raw material for various industrial products, including biofuel production [2]. The starch, protein, and oil content are three main storage components that have a direct impact on the quality of maize-based food and feed. Starch, the predominant storage component, accounts for 70%–75% of the kernel weight [3]. Maize starch is a versatile food ingredient, often employed for making sauces thicker and adding sweetness to sugars. It has also become an essential chemical feedstock for ethanol production. The second largest chemical component of the kernel is protein, which comprises 8%–10% of the grain composition [3]. The nutritional value of maize as a food source is mainly dictated by the amino acid composition of its protein. Maize oil content, which ranges from 3% to 18%, is rich in energy and polyunsaturated fatty acids, making it a valuable cooking oil [4]. Thus, grasping the natural variability in starch, protein, and oil, along with identifying QTLs and candidate genes linked to this diversity, is essential for enhancing maize grain quality.
Maize kernel composition exhibits extensive phenotypic variation among inbred lines [5,6]. Substantial efforts have been made to improve the content of kernel compositions, such as oil and protein. For instance, after over 100 generations of recurrent selection, the oil and protein content has risen to approximately 20% and 27%, respectively [7]. Similarly, after 18 cycles of selection, the oil content in the Beijing High Oil (BHO) population increased from 4.71% to 15.55% [8]. Several previous studies have revealed that the content of protein, oil, and starch are quantitative traits. QTL analysis is an effective method in genetics and genomics for pinpointing specific genomic regions linked to variations in these traits. In the maize nested association mapping population, a joint-linkage mapping and genome-wide association study (GWAS) was performed to explore the genetic basis for kernel starch, protein, and oil, identifying 21–26 QTLs [6]. Subsequently, unconditional and conditional QTL analyses for the levels of oil, protein and starch indicated a strong genetic relationship among these components in maize kernels [9]. In an RIL population, the phenotypic variation in kernel starch content is mainly influenced by a few large-effect QTLs and several minor-effect QTLs [10]. In a study of 368 maize inbred lines, 26 loci significantly associated with kernel oil concentration were identified, accounting for up to 83% of the phenotypic variation. Resequencing in a diverse germplasm collection revealed causal polymorphisms in four previously identified genes (FAD2, FATB, DGAT1-2 and WRI1a) and three newly identified genes (ACP, COPII and LACS) [11]. Using a candidate gene approach, natural variation and elite haplotypes of several starch biosynthesis genes, including bt2, sh1, and sh2 were identified for starch content and pasting properties [12]. Recently, a QTL (OsGluA2) associated with grain protein content (GPC) was cloned in rice. It was found that the causal SNP in the promoter affects its transcript expression level and GPC diversity and that the variants originated from wild rice [13]. It is important to note that grain development and nutritional quality traits are dynamic processes. The developmental process of maize kernels can be divided into three primary stages. The initial lag phase (approximately 0–15 DAP [day after pollination]) involves the formation of diploid embryos and triploid endosperms, alongside differentiation within kernel tissues. This is followed by the grain-filling phase (~12–40 DAP), characterized by substantial accumulation of starches, proteins, and oils in the endosperm, and significant growth of the embryo. Finally, the maturation phase is characterized by dehydration, acquisition of tolerance to desiccation, and the completion of embryonic development leading to the onset of dormancy [14]. However, research on the genetic characteristics influencing the content of proteins, oils, and starches during seed development remains relatively limited.
Identifying the natural variation in quality traits of maize can support efforts to enhance its nutritional value. In this study, the contents of protein (GPC), oil (GOC) and starch (GSC), were evaluated at four time points during the grain development in a RIL population. The objectives and innovative aspects included characterizing nutritional quality traits during maize grain development, identifying QTLs responsible for the contents of GPC, GOC and GSC, and identifying candidate genes related to GPC, GOC, and GSC by integrating QTL results with gene expression level and genetic variant.
2.1 Plant Materials, Field Experiments and Phenotyping Investigation
Using the single-seed descent method, the RIL population, comprising 204 F8 lines, was developed from a cross between DH1M and T877. In 2017, the experiment was carried out in a completely randomized block design with three replicates at Sanya (18°23′N, 109°44′E) in China. Each line had 78 plants arranged in plots of six rows, with each row being 3.0 meters long and spaced 0.5 meters apart, containing 13 plants per row. Pollination dates for individual RILs were recorded to schedule sampling times. In previous study [15], seed dry weight were recorded at 14 time points post-pollination (10, 15, 20, 25, 30, 35, 40, 43, 46, 49, 52, 55, 58, and 61 days after pollination), covering maize filling stage. We found that 30 DAP marked a period of rapid grain filling, while the period from 40 to 61 DAP exhibited significant variation in grain weight. Therefore, after 30 DAP, seed were taken approximately every 10 days (30, 40, 52 and 61 DAP) to measure content of protein, oil and starch [15]. For each sampling time, two similar ears for each line were selected, and the seeds were dried at 105°C for 1 h, then at 70°C–80°C to constant weight. Approximately 200 randomly chosen seeds were analyzed by a InfratecTM 1241 Grain Analyzer (FOSS, Hillerød, Denmark) for oil, protein and starch content [9]. The calibrations used in this study were developed by the manufacturer for maize seed, having a determination coefficient of 0.97 for protein, 0.97 for oil and 0.79 for starch based on 1661, 1436 and 955 samples, respectively. The descriptive statistics and phenotypic correlation of GPC, GOC, and GSC at four measurement times were implemented using the R software.
2.2 Construction of Genetic Linkage Maps and QTL Analysis
The RIL populations were genotyped using the MaizeSNP50 chip containing 56,110 SNPs. To remove redundant markers, a sliding-window approach [16] was employed to construct a bin map. The order of bin markers was verified with the ripple function in the qtl package [17]. Genetic distances between bin markers were calculated using the “Kosambi” function [18].
The observed GPC, GOC, and GSC at four different times were utilized for QTL analysis. QTL mapping was performed with a windows size of 10 cm and a step size of 1 cm by composite interval mapping (CIM) in R/qtl (v1.50) [17]. An empirical LOD threshold of 3.0 was set, and the support interval for each QTL was defined by a 1-LOD drop on either side of the peak. QTL were named following this format: q + trait name + chromosome + QTL number [19].
To pinpoint candidate gene, genetic and physical maps of QTL were integrated. All the potential candidate genes in QTL interval were identified. The reference genome based on the maize B73 RefGen_V3 genome. Gene functions were assigned using annotation information from the maizeGDB database (http://www.maizegdb.org, accessed on 10/05/2024).
To investigate the expression profiles of candidate genes within QTL, we systematically collected seed samples from T877 and DH1M lines at developmental stages of 10, 20, 30, 40, and 50 DAP. Seeds from the central portion of two comparable ears were selected for RNA isolation and subsequent RNA-seq analysis [15]. RNA-seq library was constructed using a TruSeq RNA sample preparation kit (Illumina). By removing adapters, low-quality reads, and reads containing poly-N from the raw data, the clean data were then aligned to the sequence of B73 RefGen_V3 reference genome using HISAT2 (V2.1.0). Read counts mapped to each gene were determined using FeatureCounts in the Subread package (v1.6.5). The FPKM (fragments per kilobase of exon model per million mapped reads) of each gene was calculated. Differentially expressed genes (DEGs) were identified using the R package ‘edgeR’, with criteria set at an adjusted p value < 0.05 and |log2(fold change)| ≥ 1.
Whole genome sequencing of two parental line were conducted using Illumina HiSeq 2000 [20]. Clean reads were aligned to the B73 reference genome (ftp://ftp.ensemblgenomes.org/pub/plants/release-24/fasta/zea_mays/dna/, accessed on 10/05/2024) using the BWA-MEM aligner (v0.7.17), which provides accurate alignments for both short and long reads. SNP calling was performed by SAMTools (v1.14). After filtering out low-quality SNPs, SNPs were annotated using snpEff (v4_5) and 19,155 nonsynonymous SNPs in exons were identified between T877 and DH1M. Differentially expressed genes in QTL interval with nonsynonymous variants were selected as candidate genes.
3.1 Phenotypic Variation of Grain Quality Traits in Maize
Three grain compositions, including protein (GPC), oil (GOC) and starch content (GSC), were determined by near-infrared (NIR) spectroscopy in the RIL population during the grain filling stage. Significant differences between the two parents were observed at 30, 40, 52 and 61 DAP: DH1M showed higher GPC and GOC, but lower GSC than T877 (Table 1). Within the RIL population, considerable phenotypic variations of the three grain quality traits were observed, with the coefficient of variation (CV) ranging from 5.84% to 6.73%, 8.37% to 12.30% and 1.63% to 2.24% for GPC, GOC and GSC, respectively. During the grain filling stage, GPC and GSC generally increased, while GOC decreased, with a significant change observed from 30 to 40 DAP (Fig. 1). A significant positive correlation was observed between different time points for the same trait. Additionally, protein and oil content exhibited a significant positive correlation, while starch content showed a significant negative correlation with both protein and oil content (Fig. 2).
Figure 1: Phenotypic distribution of GPC, GOC and GSC in the kernel of the RIL population at 30, 40, 52, and 61 days after pollination. The abbreviations used in the figure are as follows: GPC, grain protein content; GOC, grain oil content; and GSC, grain starch content
Figure 2: Heatmap for phenotypic correlation among GPC, GOC and GSC in the RIL population at 30, 40, 52, and 61 days after pollination. Significant correlations between traits are indicated by “**” (p < 0.01) and “*” (p < 0.05)
3.2 Genetic Linkage Map and QTL Mapping
Between the two parental lines, 9780 high-quality polymorphic SNPs were allocated to 3227 bin markers. These markers were used to construct a genetic linkage map of the DH1M × T877 RIL population (Fig. S1). The genetic map spanned a total length of 2450 cm with an average marker interval of 0.76 cm. The number of markers per chromosome ranged from 111 on chromosome 2 to 503 on chromosome 1. The sizes of the linkage groups varied, with chromosome 2 being 102.29 cm and chromosome 1 extending to 373.06 cm (Table S1). This genetic map provides a foundation for mapping QTLs associated with grain quality traits in the RIL population.
Across all 10 maize chromosomes, a total of 32 QTLs related to the traits studied during the grain filling stage were identified, including 14, 12 and 6 QTLs for GPC, GOC and GSC, respectively (Table 2 and Fig. 3). These QTLs could explain 1.59% to 19.39% of the phenotypic variation, with 8 QTLs having a phenotypic contribution exceeding 10%. The number of QTLs identified on each chromosome varied between one and five. Chromosome 1 had the most QTLs with a total of five, whereas chromosomes 3, 6, and 10 each contained four QTLs. Chromosomes 2, 7, 8, and 9 each harbored three QTLs, while chromosome 4 had two, and chromosome 5 had only one QTL.
Figure 3: QTL mapping of GPC, GOC and GSC at 30, 40, 52 and 61 DAP. DAP 30, 40, 52 and 61 are indicated by red, green, blue and purple. GPC, GOC and GSC are indicated by rectangle, triangle and inverted triangle
A total of 14 QTLs associated with GPC were detected in maize grain. Among these QTLs, six were identified at 30 DAP and located on chromosomes 3, 5, 6, 8, 9, and 10. Notably, qGPC30-6 exhibited the highest phenotypic variation, explaining 15.41% of variance, while the other five were small-effect QTLs. Notably, the favorable alleles of these five QTLs identified at 30 DAP originated from DH1M. Four QTLs were detected at 40 DAP, including one large-effect QTL (qGPC40-1) and three small-effect QTLs. Two QTLs were detected at 52 DAP, located on chromosomes 2 and 9, which explained 10.73% and 3.86% of the phenotypic variation respectively with all favorable alleles originating from DH1M. Additionally, two QTLs were detected at 61 DAP, located on chromosomes 3 and 8, with the highest PVE of 9.93% (Table 2 and Fig. 3). For GOC, twelve QTLs were identified, explaining between 2.91% and 12.23% of the total phenotypic variation. Only one QTL was associated with GOC at 30 DAP. However, six QTLs were detected at 40 DAP, with five carrying favorable allele originating from DH1M. At 40 DAP, GOC was controlled by two large-effect QTLs and four small-effect QTLs. At 52 DAP, three QTLs were identified for GOC, with qGOC52-6 explaining 14.62% of the total phenotypic variation. All favorable alleles of QTLs for GOC at 61 DAP were derived from DH1M. Regarding GSC, six QTLs were identified and explained 2.59%–19.39% of the total phenotypic variation. Two major QTLs, qGSC40-2 and qGSC52-4, explained 15.94% and 19.39% of the total phenotypic variation, respectively. Four QTLs carried the favorable allele originating from T877. Hardly any QTLs could be detected across different time points for GPC, GOC and GSC.
3.3 Integrated Multi-Omics Analysis to Identify Candidate Genes
To further investigate the genetic basis of nutritional quality traits, we integrated the QTL mapping results with transcriptome and genome variation data to identify candidate genes associated with GPC, GOC, and GSC. A total of 4035 candidate genes were identified within the confidence interval of the QTLs (Fig. 4a, Table S1). Furthermore, multi-omics data were used to narrow down the list of candidate genes. In a previous study, we compared global gene expression of T877 and DH1M at 10, 20, 30, 40, and 50 DAP, detecting 1465 (DAP10) to 6980 (DAP40) DEGs [15,20]. Additionally, whole genome resequencing was conducted for T877 and DH1M, resulting in the annotation of 8598 nonsynonymous substitutions. By integrating QTL mapping, gene expression profiles, and comparative genomics data, we identified 157, 86, and 54 DEGs with nonsynonymous substitutions between parental lines associated with GPC, GOC, and GSC, respectively (Fig. 4a, Tables S2–S4). Subsequently, we performed gene function annotation, which resulted in the identification of 15 candidate genes potentially involved in regulating grain quality traits (Table 3).
Figure 4: Identification of candidate genes by integrating QTL mapping, gene expression, co-expression analysis and comparative genome for GPC, GOC and GSC. (a) The QTL candidate gene prediction process. (b) The expression level of candidate gene for content of protein (GPC); content of oil (GOC); content of starch (GSC) in different accessions
GRMZM5G813651, the candidate gene for qGPC30-3 associated with GPC at 30 DAP (Table 3), encodes a NAC transcription factor. The expression level showed a significant difference between T877 and DH1M at 50 DAP, and one nonsynonymous SNP was observed in the third exon. The candidate genes for qGPC30-5 and qGPC30-6, GRMZM2G178753 and GRMZM2G474777, encode Leucine-rich repeat protein kinases. These genes showed significant differential expression between T877 and DH1M at 10 and 40 DAP, respectively (Fig. 4b and Table 3), with each containing a nonsynonymous SNP in exon 1. GRMZM5G814279, associated with qGPC30-9, encodes a MADS-box transcription factor. The expression level showed a significant difference between T877 and DH1M at 10 and 30 DAP, with a nonsynonymous SNP observed in the seventh exon. For qGPC30-10, GRMZM2G123633 encodes a cell wall invertase, showing significantly different between T877 and DH1M at 30 DAP. Additionally, BURP domain-containing protein GRMZM2G108422, and two transcription factors GRMZM2G073427 and GRMZM2G051256 were associated with GPC at 40 DAP. GRMZM2G169820, the candidate gene for qGOC40-1 associated with GOC at 40 DAP (Fig. 4b and Table 3), encodes an auxin response factor, and the expression level showed a significant difference between T877 and DH1M at 40 and 50 DAP. One nonsynonymous SNP was observed in the 13th exon of GRMZM2G169820. GRMZM2G173759 and GRMZM2G147966 were located in qGOC52-7 and encode Cellulose-synthase-like and Riboflavin synthase-like superfamily protein, respectively. GRMZM2G149994, the candidate gene for qGSC30-10 associated with GSC at 30 DAP (Fig. 4b and Table 3), is involved in the cell division cycle, and the expression level showed a significant difference between T877 and DH1M at 30 and 40 DAP. One nonsynonymous SNP was observed in the eighth exon of GRMZM2G149994. GRMZM2G162690, GRMZM2G060257 and GRMZM2G040743 were located in qGSC40-1 and encode trehalase, phloem protein 2-A13 and Calcium-dependent protein kinase family protein, respectively.
Grain nutritional quality traits in maize are dynamic and complex quantitative traits, governed by numerous genes. Significant effort has been invested in developing maize varieties that meet market demands for altered kernel composition [6]. Identifying QTLs for grain nutritional quality traits will aid Marker-Assisted Selection (MAS) breeding and the identification of key genes and elite alleles from natural variations. In this study, a total of 14, 12, and 6 QTLs for GPC, GOC, and GSC during the grain-filling stage were identified, respectively. Previous QTLs studies have identified a broad range in the number of QTLs associated with kernel composition [6,10,21]. Based on the phenotypic variance, the genetic architecture of grain composition is controlled by a few large-effect QTLs (r2 > 10%) and numerous small-effect QTLs. This finding is consistent with other studies in the bi-parent populations [9,21].
QTL mapping is an effective technique for identifying natural variations in complex traits; however, its accuracy is constrained by the limited number of recombination events [22]. In this study, the average length of the QTL confidence interval is 7.74 cm (8.8 Mb); without time-consuming fine mapping, it is challenging to identify the causal gene among the thousands of candidate genes [23]. Multi-omics analysis serves as a robust approach for predicting functional genes [24,25]. Here, by combining QTL mapping, gene expression profiling, and genetic variants, 157, 86, and 54 genes were detected for the GPC, GOC, and GSC, respectively. Among the genes for GPC, four genes encoded transcription factors, including NAC, MADS-box, bZIP, and MYB. GRMZM5G813651 encoded a NAC transcription factor associated with GPC at 30 DAP. Two endosperm-specific NAC transcription factors, ZmNAC128 and ZmNAC130, regulate the accumulation of starch and protein in maize seeds [26]. In rice, OsNAC20 and OsNAC26 affect storage protein accumulation by directly controlling the expression of genes involved in storage protein synthesis [27]. The maize kernel-specific transcription factor Opaque2, a bZIP protein, controls zein synthesis [28]. Additionally, ZmMADS47 has been found to regulate zein gene transcription by interacting with Opaque2 [29]. In this study, we identified that GRMZM2G073427 encodes a bZIP transcription factor, while GRMZM5G814279 encodes a MADS-box transcription factor. GRMZM2G051256 associated with GPC at 40 DAP, encoded a MYB transcription factor. In rice, OsMYB5 could bind to the promoters of seed storage protein genes [30]. Another gene, GRMZM2G108422, encodes a BURP domain-containing protein. In Arabidopsis thaliana, the BURP domain protein AtUSPL1 is targeted to the protein storage vacuoles [31].
GRMZM2G169820, associated with GOC at 40 DAP, is predicted to encode an auxin response factor (ARF). ARFs play essential roles in the auxin signaling pathway, influencing various plant biological processes. In camelina, CsARF8 can regulate CsFAD3 expression either directly or via the ABI3-bZIP12 pathway, thereby impacting α-linolenic acid accumulation [32]. In the qGOC52-7 region, we identified two candidate genes: GRMZM2G173759, which encodes a cellulose-synthase-like protein, and GRMZM2G147966, which encodes a riboflavin synthase-like superfamily protein. In Arabidopsis, Reducing the expression of AtCESA1 resulted in approximately a 3% decrease in oil content [33]. In maize, Dek33 encodes a pyrimidine reductase that is crucial for riboflavin biosynthesis and essential for oil-body formation [34].
GRMZM2G149994 participants in the cell division cycle are involved in GSC at 30 DAP. In Chlamydomonas, the two Cell Division Cycle 5 mutants, crcdc5-1 and crcdc5-2 were found to accumulate significantly higher levels of starch and oil [35]. GRMZM2G162690, GRMZM2G060257, and GRMZM2G040743 were identified as candidate genes in qGSC40-1. GRMZM2G162690 encodes a trehalase, the only enzyme that catalyzes the hydrolysis of trehalose to glucose. Trehalose, an important compatible solute in plants, may regulate carbohydrate allocation [36]. GRMZM2G040743 encodes a calcium-dependent protein kinase family protein. The calcium-dependent seed-specific protein kinase (SPK) is crucial for seed development and plays a role in the metabolic pathway that converts sucrose to storage starch in immature seeds [37]. These candidate genes have the potential to influence the accumulation of protein, oil, and starch in maize seeds. As a next step, we plan to conduct functional assays such as gene knockout or overexpression studies in maize to verify the roles of these candidate genes in regulating grain quality traits.
Acknowledgement: Not applicable.
Funding Statement: This work was supported by the Key Research and Development Program of Jiangsu Province (BE2022343), the Seed Industry Revitalization Project of Jiangsu Province (JBGS[2021]009), the National Natural Science Foundation of China (32061143030 and 31972487), Jiangsu Province University Basic Science Research Project (21KJA210002), the Innovative Research Team of Universities in Jiangsu Province, the High-End Talent Project of Yangzhou University, the Priority Academic Program Development of Jiangsu Higher Education Institutions (PAPD), and Qing Lan Project of Jiangsu Province.
Author Contributions: Zefeng Yang, Chenwu Xu and Pengcheng Li conceived and designed the study. Pengcheng Li and Shuangyi Yin designed the experiments. Tianze Zhu, Xinjie Zhu, Minggang Ji and Wenye Rui conducted experiments. Pengcheng Li, Shuangyi Yin, Yunyun Wang and Tianze Zhu analyzed the data. Tianze Zhu, Pengcheng Li, Shuangyi Yin, Yunyun Wang and Houmiao Wang wrote the paper. All authors reviewed the results and approved the final version of the manuscript.
Availability of Data and Materials: The raw data of genome resequencing for two parents have been deposited into the NCBI Sequence Read Archive (SRA, https://www.ncbi.nlm.nih.gov/sra/, accessed on 10/05/2024) under accession number PRJNA645641. RNA-seq data was deposited in the Gene Expression Omnibus (GEO, https://www.ncbi.nlm.nih.gov/geo/, accessed on 10/05/2024) under accession number GSE130930.
Ethics Approval: Not applicable.
Conflicts of Interest: The authors declare that they have no conflicts of interest to report regarding the present study.
Supplementary Materials: The supplementary material is available online at https://doi.org/10.32604/phyton.2024.052219.
References
1. Erenstein O, Jaleta M, Sonder K, Mottaleb K, Prasanna B. Global maize production, consumption and trade: trends and R&D implications. Food Secur. 2022;14(5):1295–319. doi:10.1007/s12571-022-01288-7. [Google Scholar] [CrossRef]
2. Kaushal M, Sharma R, Vaidya D, Gupta A, Saini HK, Anand A, et al. Maize: an underexploited golden cereal crop. Cereal Res Commun. 2023;51(1):3–14. doi:10.1007/s42976-022-00280-3. [Google Scholar] [CrossRef]
3. Prasanthi P, Naveena N, Vishnuvardhana Rao M, Bhaskarachary K. Compositional variability of nutrients and phytochemicals in corn after processing. J Food Sci Technol. 2017;54:1080–90. doi:10.1007/s13197-017-2547-2. [Google Scholar] [PubMed] [CrossRef]
4. James M, Myers A. Seed starch synthesis. In: Bennetzen JL, Hake SC editors. Handbook of maize: its biology. New York: Springer; 2009. p. 439–56. [Google Scholar]
5. Karn A, Gillman JD, Flint-Garcia SA. Genetic analysis of teosinte alleles for kernel composition traits in maize. G3: Genes Genom Genet. 2017;7:1157–64. doi:10.1534/g3.117.039529. [Google Scholar] [PubMed] [CrossRef]
6. Cook JP, McMullen MD, Holland JB, Tian F, Bradbury P, Ross-Ibarra J, et al. Genetic architecture of maize kernel composition in the nested association mapping and inbred association panels. Plant Physiol. 2012;158(2):824–34. doi:10.1104/pp.111.185033. [Google Scholar] [PubMed] [CrossRef]
7. Moose SP, Dudley JW, Rocheford TR. Maize selection passes the century mark: a unique resource for 21st century genomics. Trends Plant Sci. 2004;9(7):358–64. doi:10.1016/j.tplants.2004.05.005. [Google Scholar] [PubMed] [CrossRef]
8. Song T, Chen S. Long term selection for oil concentration in five maize populations. Maydica. 2004;49(1):9–14. [Google Scholar]
9. Guo Y, Yang X, Chander S, Yan J, Zhang J, Song T, et al. Identification of unconditional and conditional QTL for oil, protein and starch content in maize. Crop J. 2013;1(1):34–42. doi:10.1016/j.cj.2013.07.010. [Google Scholar] [CrossRef]
10. Wang T, Wang M, Hu S, Xiao Y, Tong H, Pan Q, et al. Genetic basis of maize kernel starch content revealed by high-density single nucleotide polymorphism markers in a recombinant inbred line population. BMC Plant Biol. 2015;15(1):1–12. [Google Scholar]
11. Li H, Peng Z, Yang X, Wang W, Fu J, Wang J, et al. Genome-wide association study dissects the genetic architecture of oil biosynthesis in maize kernels. Nat Genet. 2013;45(1):43–50. doi:10.1038/ng.2484. [Google Scholar] [PubMed] [CrossRef]
12. Wilson LM, Whitt SR, Ibaéñez AM, Rocheford TR, Goodman MM, Buckler ES IV. Dissection of maize kernel composition and starch production by candidate gene association. Plant Cell. 2004;16(10):2719–33. doi:10.1105/tpc.104.025700. [Google Scholar] [PubMed] [CrossRef]
13. Yang Y, Guo M, Sun S, Zou Y, Yin S, Liu Y, et al. Natural variation of OsGluA2 is involved in grain protein content regulation in rice. Nat Commun. 2019;10(1):1949. doi:10.1038/s41467-019-09919-y. [Google Scholar] [PubMed] [CrossRef]
14. Wu H, Becraft PW, Dannenhoffer JM. Maize endosperm development: tissues, cells, molecular regulation and grain quality improvement. Front Plant Sci. 2022;13:852082. doi:10.3389/fpls.2022.852082. [Google Scholar] [PubMed] [CrossRef]
15. Yin S, Li P, Xu Y, Liu J, Yang T, Wei J, et al. Genetic and genomic analysis of the seed-filling process in maize based on a logistic model. Heredity. 2020;124(1):122–34. doi:10.1038/s41437-019-0251-x. [Google Scholar] [PubMed] [CrossRef]
16. Huang X, Feng Q, Qian Q, Zhao Q, Wang L, Wang A, et al. High-throughput genotyping by whole-genome resequencing. Genome Res. 2009;19(6):1068–76. doi:10.1101/gr.089516.108. [Google Scholar] [PubMed] [CrossRef]
17. Arends D, Prins P, Jansen RC, Broman KW. R/qtl: high-throughput multiple QTL mapping. Bioinformatics. 2010;26(23):2990–2. doi:10.1093/bioinformatics/btq565. [Google Scholar] [PubMed] [CrossRef]
18. Kosambi DD. The estimation of map distances from recombination values. In: Kosambi DD, editor. Selected works in mathematics and statistics. New Delhi: Springer; 2016. p. 125–30. [Google Scholar]
19. McCouch SR. Report on QTL nomenclature. Rice Genet Newsl. 1997;14:11–3. [Google Scholar]
20. Li P, Yin S, Wang Y, Zhu T, Zhu X, Ji M, et al. Dynamics and genetic regulation of macronutrient concentrations during grain development in maize. J Integr Agr. 2024;23(3):781–94. doi:10.1016/j.jia.2023.11.003. [Google Scholar] [CrossRef]
21. Lu X, Zhou Z, Wang Y, Wang R, Hao Z, Li M, et al. Genetic basis of maize kernel protein content revealed by high-density bin mapping using recombinant inbred lines. Front Plant Sci. 2022;13:1045854. doi:10.3389/fpls.2022.1045854. [Google Scholar] [PubMed] [CrossRef]
22. Mackay TF, Anholt RR. Pleiotropy, epistasis and the genetic architecture of quantitative traits. Nat Rev Genet. 2024;52:1–19. [Google Scholar]
23. Bazakos C, Hanemian M, Trontin C, Jiménez-Gómez JM, Loudet O. New strategies and tools in quantitative genetics: how to go from the phenotype to the genotype. Annu Rev Plant Biol. 2017;68:435–55. doi:10.1146/arplant.2017.68.issue-1. [Google Scholar] [CrossRef]
24. Schaefer RJ, Michno J-M, Jeffers J, Hoekenga O, Dilkes B, Baxter I, et al. Integrating coexpression networks with GWAS to prioritize causal genes in maize. Plant Cell. 2018;30(12):2922–42. doi:10.1105/tpc.18.00299. [Google Scholar] [PubMed] [CrossRef]
25. Sekhon RS, Saski C, Kumar R, Flinn BS, Luo F, Beissinger TM, et al. Integrated genome-scale analysis identifies novel genes and networks underlying senescence in maize. Plant Cell. 2019;31(9):1968–89. doi:10.1105/tpc.18.00930. [Google Scholar] [PubMed] [CrossRef]
26. Zhang Z, Dong J, Ji C, Wu Y, Messing J. NAC-type transcription factors regulate accumulation of starch and protein in maize seeds. Proc Natl Acad Sci U S A. 2019;116(23):11223–8. doi:10.1073/pnas.1904995116. [Google Scholar] [PubMed] [CrossRef]
27. Wang J, Chen Z, Zhang Q, Meng S, Wei C. The NAC transcription factors OsNAC20 and OsNAC26 regulate starch and storage protein synthesis. Plant Physiol. 2020;184(4):1775–91. doi:10.1104/pp.20.00984. [Google Scholar] [PubMed] [CrossRef]
28. Chen E, Yu H, He J, Peng D, Zhu P, Pan S, et al. The transcription factors ZmNAC128 and ZmNAC130 coordinate with Opaque2 to promote endosperm filling in maize. Plant Cell. 2023;35(11):4066–90. doi:10.1093/plcell/koad215. [Google Scholar] [PubMed] [CrossRef]
29. Qiao Z, Qi W, Wang Q, Yn F, Yang Q, Zhang N, et al. ZmMADS47 regulates zein gene transcription through interaction with Opaque2. PLoS Genet. 2016;12(4):e1005991. doi:10.1371/journal.pgen.1005991. [Google Scholar] [PubMed] [CrossRef]
30. Suzuki A, Wu CY, Washida H, Takaiwa F. Rice MYB protein OSMYB5 specifically binds to the AACA motif conserved among promoters of genes for storage protein glutelin. Plant Cell Physiol. 1998;39(5):555–9. doi:10.1093/oxfordjournals.pcp.a029404. [Google Scholar] [PubMed] [CrossRef]
31. Van Son L, Tiedemann J, Rutten T, Hillmer S, Hinz G, Zank T, et al. The BURP domain protein AtUSPL1 of Arabidopsis thaliana is destined to the protein storage vacuoles and overexpression of the cognate gene distorts seed development. Plant Mol Biol. 2009;71:319–29. doi:10.1007/s11103-009-9526-6. [Google Scholar] [PubMed] [CrossRef]
32. Na G, Mu X, Grabowski P, Schmutz J, Lu C. Enhancing micro RNA 167A expression in seed decreases the α-linolenic acid content and increases seed size in Camelina sativa. Plant J. 2019;98(2):346–58. doi:10.1111/tpj.2019.98.issue-2. [Google Scholar] [CrossRef]
33. Jayawardhane KN, Singer SD, Ozga JA, Rizvi SM, Weselake RJ, Chen G. Seed-specific down-regulation of Arabidopsis CELLULOSE SYNTHASE 1 or 9 reduces seed cellulose content and differentially affects carbon partitioning. Plant Cell Rep. 2020;39:953–69. doi:10.1007/s00299-020-02541-z. [Google Scholar] [PubMed] [CrossRef]
34. Dai D, Tong H, Cheng L, Peng F, Zhang T, Qi W, et al. Maize Dek33 encodes a pyrimidine reductase in riboflavin biosynthesis that is essential for oil-body formation and ABA biosynthesis during seed development. J Exp Bot. 2019;70(19):5173–87. doi:10.1093/jxb/erz268. [Google Scholar] [PubMed] [CrossRef]
35. Torres-Romero I, Kong F, Légeret B, Beisson F, Peltier G, Li-Beisson Y. Chlamydomonas cell cycle mutant crcdc5 over-accumulates starch and oil. Biochimie. 2020;169:54–61. doi:10.1016/j.biochi.2019.09.017. [Google Scholar] [PubMed] [CrossRef]
36. Gabriel C, Fernhout JJ, Fichtner F, Feil R, Lunn JE, Kossmann J, et al. Genetic manipulation of trehalose-6-phosphate synthase results in changes in the soluble sugar profile in transgenic sugarcane stems. Plant Direct. 2021;5(11):e358. doi:10.1002/pld3.v5.11. [Google Scholar] [CrossRef]
37. Niu B, Zhang Z, Zhang J, Zhou Y, Chen C. The rice LEC1-like transcription factor OsNF-YB9 interacts with SPK, an endosperm-specific sucrose synthase protein kinase, and functions in seed development. Plant J. 2021;106(5):1233–46. doi:10.1111/tpj.v106.5. [Google Scholar] [CrossRef]
Cite This Article
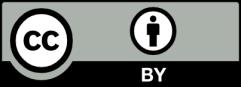
This work is licensed under a Creative Commons Attribution 4.0 International License , which permits unrestricted use, distribution, and reproduction in any medium, provided the original work is properly cited.