Open Access
ARTICLE
Multi-Scale Mixed Attention Tea Shoot Instance Segmentation Model
1 School of Automation, Hangzhou Dianzi University, Hangzhou, 310018, China
2 Tea Research Institute, Chinese Academy of Agriculture Sciences, Hangzhou, 310018, China
3 School of Information Engineering, Zhejiang University of Water Resources and Electric Power, Hangzhou, 310018, China
* Corresponding Author: Kaihua Wu. Email:
Phyton-International Journal of Experimental Botany 2024, 93(2), 261-275. https://doi.org/10.32604/phyton.2024.046331
Received 27 September 2023; Accepted 25 December 2023; Issue published 27 February 2024
Abstract
Tea leaf picking is a crucial stage in tea production that directly influences the quality and value of the tea. Traditional tea-picking machines may compromise the quality of the tea leaves. High-quality teas are often handpicked and need more delicate operations in intelligent picking machines. Compared with traditional image processing techniques, deep learning models have stronger feature extraction capabilities, and better generalization and are more suitable for practical tea shoot harvesting. However, current research mostly focuses on shoot detection and cannot directly accomplish end-to-end shoot segmentation tasks. We propose a tea shoot instance segmentation model based on multi-scale mixed attention (Mask2FusionNet) using a dataset from the tea garden in Hangzhou. We further analyzed the characteristics of the tea shoot dataset, where the proportion of small to medium-sized targets is 89.9%. Our algorithm is compared with several mainstream object segmentation algorithms, and the results demonstrate that our model achieves an accuracy of 82% in recognizing the tea shoots, showing a better performance compared to other models. Through ablation experiments, we found that ResNet50, PointRend strategy, and the Feature Pyramid Network (FPN) architecture can improve performance by 1.6%, 1.4%, and 2.4%, respectively. These experiments demonstrated that our proposed multi-scale and point selection strategy optimizes the feature extraction capability for overlapping small targets. The results indicate that the proposed Mask2FusionNet model can perform the shoot segmentation in unstructured environments, realizing the individual distinction of tea shoots, and complete extraction of the shoot edge contours with a segmentation accuracy of 82.0%. The research results can provide algorithmic support for the segmentation and intelligent harvesting of premium tea shoots at different scales.Keywords
Cite This Article
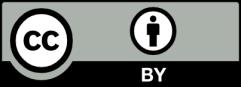
This work is licensed under a Creative Commons Attribution 4.0 International License , which permits unrestricted use, distribution, and reproduction in any medium, provided the original work is properly cited.