Open Access
ARTICLE
Characterization and Genetic Diversity of Tea (Camellia sinensis (L.) O. Kuntze) Genotypes for Waterlogging Tolerance
1 Department of Crop Botany, Bangladesh Agricultural University, Mymensingh, 2202, Bangladesh
2 Botany Division, Bangladesh Tea Research Institute, Moulvibazar, 3210, Bangladesh
* Corresponding Author: A. K. M. Golam Sarwar. Email:
Phyton-International Journal of Experimental Botany 2024, 93(12), 3411-3442. https://doi.org/10.32604/phyton.2024.058893
Received 23 September 2024; Accepted 28 November 2024; Issue published 31 December 2024
Abstract
Tea estates in Bangladesh are currently experiencing frequent waterlogging stress due to heavy rainfall or flooding under changing climate scenarios. The development of waterlogging-tolerant tea cultivars is, therefore, crucial for sustainable tea production with increased yield in waterlogging-affected areas. In this study, a total of two hundred tea genotypes were screened for waterlogging tolerance based on yield and quality potentials. Tea genotypes were raised at the nursery and evaluated with 13 traits at the seedling and mature stages. Descriptive and multivariate analysis of the recorded data of measured traits were performed for clustering the studied genotypes in relation to their yield and quality potential. The 200 tea genotypes were categorized into six clusters and the measured traits were classified into two groups. Cluster-5 comprised 46 closely related tea genotypes whereas other clusters-Cluster-1, 2, 3, 4, and 6 contained 39, 20, 24, 29, and 42 genotypes, respectively. PCA-biplot showed that the first two principal components (PC 1 and PC 2) cumulatively described 56.9% of total variations. From the genetic diversity analysis, the characteristics like green leaf yield (GLY), plucking points (PP), spreading of bush frame (SBF), plucking interval (PI), dormancy period (DR), black tea quality score (QSB), fermentation rate (SF) and number of pubescence (NP) exhibited high to moderate heritability, indicating to consider these traits as selection criteria for tea breeding program. The trait GLY showed a strong positive correlation with PP, and SBF and a negative association with PI and DR. The key quality trait, QSB had a positive correlation with SF and NP. Based on these studied descriptors, a total of 11 tea genotypes were selected, found potential for waterlogging tolerance, and can be considered for varietal improvement programs.Keywords
Abbreviation
BTRI | Bangladesh Tea Research Institute |
C | Cluster |
DR | Number of Dormancy Periods |
G | Group |
GA | Genetic Advance |
GAM | Genetic Advance as Percent of Mean |
GCV | Genotypic Coefficient of Variation |
GDP | Gross Domestic Product |
GLY | Green Leaf Yield |
h2b | Heritability in Broad Sense |
LB | Length of Bud |
NF | Number of Flower |
NP | Number of Pubescence of Leaf Bud |
PC | Principal Component |
PCA | Principal Component Analysis |
PCV | Phenotypic Coefficient of Variation |
PI | Number of Days of Plucking Interval |
PP | Number of Plucking Points |
Q1 | First Quartile |
Q3 | Third Quartile |
QSB | Quality Score of Black Tea |
QSW | Quality Score of White Tea |
r | Correlation Coefficient |
RCBD | Randomized Complete Block Design |
RP | Recovery Days after Pruning |
SBF | Spreading of Bush Frame |
SF | Score of Fermentation Rate of Leaf |
SP | Survivability Percentage |
TE | Tea Estate |
VP | Vegetative Propagated |
Tea (Camellia sinensis (L.) O. Kuntze) is one of the most popular drinks for health benefits worldwide [1]. China was the first place where tea was cultivated, and because of its restorative and rejuvenating properties, people have been drinking it for medical purposes since ancient times [2,3]. Tea contains more than 700 compounds including caffeine, catechin, different flavonoids, vitamins (C, E, K) with amino acids, etc., and these components are mainly responsible for the therapeutic value of tea [4].
The tea industry in Bangladesh has immense contributions to the national economy by contributing 1% to the Gross Domestic Product [5]. Commercial tea cultivation in Bangladesh was started in 1854 [6], tea industry in Bangladesh has touched the milestone of record production of 102.92 million kg in 2023 [7]. The increase in Bangladesh’s average daily intake of tea, one of the main cash crops for export, has converted tea into an import. To restore our lost glory of exports, tea production in our limited land must be increased to such an extent, so that, there is a surplus after meeting domestic demand. But our yield has not increased significantly compared to other tea-producing countries due to various reasons such as climate extremes related to climate change, low soil organic carbon, less use of high-yielding and tolerant varieties, improper agronomic management, different kinds of biotic and abiotic stresses and so on [8,9].
Different kinds of abiotic stresses are responsible for lower yields in many crops. Among the various abiotic stresses, waterlogged stress is one of the major factors for yield reduction. The waterlogging situation stunts growth by creating abiotic conditions in the root zone [10]. It can reduce 7.48%–57.42% of rice production [11], 7.75%–16.30% in wheat [12], and 7%–80% in maize [13] throughout different growth stages of the crop. Waterlogging has become a serious issue for tea cultivation reducing yield by 15%–20% annually in North-East India [14]. In Bangladesh, almost all tea estates of the greater Sylhet division have suffered from waterlogging to various extents. However, in this country, till now, there has been a lack of waterlogging-tolerant varieties or sufficient research for the development of waterlogging-tolerant varieties of tea. So, the collection of germplasm from waterlogged areas, screening them by genetic diversity analysis with consideration of yield and quality as well as further screening them based on waterlogging tolerant ability could be a possible solution to face waterlogging problems by selection breeding strategy.
A successful breeding technique comprises several steps, such as collecting germplasms, evaluating the performance of germplasms by different analyses, and conserving them for future research. So, the evaluation of genetic variation through multivariate analysis is the base of any crop improvement program [15]. Genetic multivariate diversity analyses measure the extent and pattern of variations that exist among the genotypes [16]. Conservation of germplasms also requires analysis of genetic variability to avoid duplication within existing genotypes. Estimation of genetic and phenotypic variability is also important to realize the effect of the environment on phenotypic expression which is related to productivity [17]. Characters associated with economic yield with less environmental interaction and higher heritability from parent to offspring with high genetic gain (genetic advance) of a particular crop are the basic interest of any breeder [18]. The objective of the research was to identify important characters as well as genotypes with high yield and quality potential for waterlogging tolerance through characterization and analysis of genetic variability.
2.1 Genotypes and Collection Site
A total of 200 tea genotypes were collected from waterlogged areas of twenty-one Tea Estates (TEs) spread over different agroecological zones of Sylhet division from 2021–2023 by selection breeding process. Plantations of tea estates comprise both seedling and clonal planting materials; therefore, the current germplasm selection process was carried out in old seedling population areas to exploit more natural variations. The collection of sources (name of the TE) of germplasms with genotype names and code names were provided in Table A1. Some important agrometeorological data like monthly total precipitation (mm), monthly maximum and minimum temperature (°C), monthly total evaporation (mm), monthly average sunshine hour, and monthly average relative humidity (%) of germplasm collection sites (greater Sylhet) from 2021 to 2023 [19,20] are given in Table A2.
Currently, in Bangladesh, 168 tea estates are having a production of 102.92 million kg in 2023 [7]. Almost 73% of the total output came from three districts of the Sylhet division (Moulvibazar district: 50%, Habiganj district: 18% and Sylhet district: 5%). On the other hand, 16% of production came from the Northern region of the country, which is generally flat lands planting clonal varieties with limited waterlogging problems. The remaining 11% of tea production came from the Chattogram district [21]. Till now, few records have been found for waterlogging problems in the tea estates of the Chattogram region. So, current research was conducted mainly focusing on the Sylhet division, in which some areas are now severely affected by waterlogging problems and collected the tea genotypes from these affected areas.
2.2 Experimental Design and Data Collection Parameters
Collected genotypes from naturally waterlogged areas (standing plantations) of tea estates were raised at the Vegetative Propagated (VP) nursery of Bangladesh Tea Research Institute (BTRI), Sreemangal, Moulvibazar. Plants were raised by following standard procedure [22]. The experiment was conducted by Randomized Complete Block Design with four replications. Data were collected under 13 parameters (characters) from plants of one-year saplings at BTRI VP nursery as well as mature tea bushes from estates and BTRI New Germplasm Center. Measured characters with brief descriptions and calculation methods are given below.
2.2.1 Green Leaf Yield-GLY (g Plant−1)
GLY was the average weight of green leaf (g) per plant [23] of eight plucking rounds. Based on the average GLY of a minimum of eight plucking rounds, the genotypes were categorized as high (over 200 g plant−1), average (150 to 200 g plant−1), and low yielder (less than 150 g plant−1) [24].
2.2.2 Number of Plucking Points-PP (m−2)
PP was the number of plucking points per square meter area of bush [25].
2.2.3 Number of Days of Plucking Interval-PI (Days)
PI referred to as the plucking interval is the number of days between two successive plucking rounds [26].
2.2.4 Spreading of Bush Frame-SBF (cm2)
The size or spreading of the tea bush area [27] was calculated by the area (C) = 2πr formula (where C is the circumference, r is the radius of the bush, and π ≈ 3.14).
2.2.5 Number of Dormancy Period-DR
The dormancy period or dormancy cycle or banjhi period is the alternate period of flushing which ranges from 2 to 4 in a cropping year [28].
2.2.6 Number of Pubescence of Leaf Bud-NP
Number of pubescence of a single leaf bud which is an indicator of made tea quality [29] was recorded by compound microscope.
2.2.7 Score of Fermentation Rate of Leaf-SF
The rate of fermentation (oxidation) by chloroform (Sigma-Aldrich, USA, supplied by Supelco) test [30] was done out of 10 marks.
LB refers to the length of a single bud (cm) which has a relation with quality and economic use [31].
2.2.9 Quality Score of Black Tea-QSB
Quality Scoring of CTC black tea of each genotype was recorded out of 50 marks by organoleptic tasting method [32]. Genotypes are classified based on quality scores, such as excellent quality (score more than 34), average (32–34), and below average (less than 32).
2.2.10 Quality Score of White Tea-QSW
White tea of each genotype was manufactured and scored out of 50 marks by organoleptic tasting method [33].
2.2.11 Recovery Days after Pruning-RP (Days)
RP was the days to reach bush height to tipping height after the pruning operation.
2.2.12 Number of Flower-NF (Plant−1)
NF refers to the number of flowers of every plant during the flowering season.
2.2.13 Survivability Percentage-SP (Percent)
Cuttings from selected bushes were planted in the nursery and then the survival percentage of cuttings of each genotype was calculated by SP = (Cuttings survived ÷ Cuttings planted) × 100 formula.
2.3 Statistical Analysis of Experimental Data
The range, first quartile (Q1), median, third quartile (Q3), mean and outliers were graphically presented by boxplot through descriptive statistical analysis [34,35] by R software (version 4.3.2). The data were standardized and a hierarchical clustering heatmap was constructed using R software (version 4.3.2). The principal component analysis (PCA) [36] and Pearson’s correlation matrix [37–39] were made using fviz_pca and the corrplot package of the R program.
The genotypic coefficient of variation (GCV) is expressed as percent and calculated by [(square root of genotypic variance ÷ mean value of the character) × 100] formula, while the phenotypic coefficient of variation (PCV) is determined by [square root of phenotypic variance ÷ mean value of the character) × 100] formula also expressed as percent [40]. GCV and PCV were classified as low, moderate, and high when the values were less than 10%, 10%–20%, and more than 20% respectively [41]. Heritability in a Broad sense (h2b) is a percent ratio of genotypic variance and phenotypic variance [42] which is classified as high (>75%), moderate or medium (50%–75%), and low (<50%) [43]. Genetic advance (GA) is classified as low (less than 10%), moderate (10%–20%), and high (greater than 20%) which is obtained by multiplication of three factors: selection differential which is standardized at a 5% level of intensity, phenotypic standard deviation and h2b [44]. Genetic advance as percent of mean (GAM) is the ratio of GA and mean value which is also expressed as percent [45]. In this study, genetic diversity analysis and analysis of variance with multiple comparisons (Tukey’s HSD test) was performed by R statistical software (version 4.3.2).
3.1 Descriptive Analysis of 200 Genotypes under 13 Measured Characters
The boxplots indicating lower and upper values, first quartile (Q1), median, third quartile (Q3), mean and outliers of 13 measured characters of 200 genotypes are presented in Fig. 1. The GLY per plant ranged from 168.34 to 200.72 g with mean value of 179.6 g of which 25% values were placed under 172.22 g (Q1) and 75% values were found under 187.37 g (Q3). PP and PI ranged from 61 to 83 in m−2 and 7.25 days to 12.75 days which had a mean value of 66.67 in m−2 and 10.66 days respectively. The character SBF had a mean value of 1.99 cm2 while DR values were found ranging from 2 to 4 with an average value of 3.27. The mean values of NP, SF, LB, QSB, QSW, NF, and SP were 1461.45, 6.60, 3.53 cm, 32.48%, 32.46%, 458.37% and 92.91%, respectively. The data were uniformly distributed in RP having mean values of 44.7 days while PI, LB, QSB, QSW, NF, and SP had some outliers (Fig. 1).
Figure 1: Descriptive summary of 200 Genotypes against 13 characters as presented by boxplots. The horizontal line, upper boundary, and lower boundary of each box indicated the median, 1st percentile (Q1), and 3rd percentile (Q3). The upper and lower whiskers and black circles denote the Q3 + 1.5 interquartile range, Q1 − 1.5 interquartile range, and mean value respectively. Interquartile range (IQR) = Q3 − Q1. Descriptions of the characters: GLY: Green leaf yield plant−1 (g), PP: Number of plucking points in m−2, PI: Number of days of plucking interval, SBF: Spreading of bush frame (cm2), DR: Number of dormancy period, NP: Number of pubescence of single leaf bud, SF: Score of fermentation rate of green leaf, LB: Length of single bud (cm), QSB: Quality score of black tea, QSW: Quality score of white tea, RP: Recovery days after pruning, NF: Number of flowers plant−1, SP: Survivability percentage of cuttings of each genotype
3.2 Cluster Analysis of Genotypes and Variables
Two hundred tea genotypes and their performance are illustrated in a two-way hierarchical clustering heatmap (Fig. 2). The genotypes and characters were divided into clusters and groups, respectively based on the variations that existed among them, but closely interlinked variables were placed within a single cluster or group. The left side of the heatmap represented the column of the dendrogram with six clusters while the genotypes within the corresponding clusters were presented on the right side of the heatmap. The maximum number (46) of closely associated genotypes were found in Cluster-5 (C-5) while the other clusters such as Cluster-1 (C-1), Cluster-2 (C-2), Cluster-3 (C-3), Cluster-4 (C-4) and Cluster-6 (C-6) comprised of 39, 20, 24, 29 and 42 genotypes, respectively. On the contrary, two groups (G-1 and G-2) of dendrogram were found at the upper side of the heatmap while the characters were placed group-wise at the bottom. The characters like GLY, PP, and SBF possessed genotypes closely associated in Cluster-1 and Cluster-2 with higher normalized values (deep green color). On the other hand, PI and DR comprised of genotypes with lower normalized values (deep red color) were also grouped into Cluster-1 and Cluster-2. However, the genotypes having better quality full black tea potential were found in Cluster-3, Cluster-4, and Cluster-1.
Figure 2: The two-way hierarchical clustering heatmap with dendrogram illustrates the grouping and association of genotypes and characters. 200 tea genotypes were grouped into six clusters (Cluster-1, Cluster-2, Cluster-3, Cluster-4, Cluster-5 and Cluster-6) with 2 groups of characters (Group-1 and Group-2). The mean values are normalized with a scale of 4 to (−) 4 of deep green to deep red color respectively to describe the intensity of the effect against each character. Descriptions of the characters: SF: Score of fermentation rate of green leaf, NP: Number of pubescence of single leaf bud, QSB: Quality score of black tea, PI: Number of days of plucking interval, DR: Number of dormancy period, QSW: Quality score of white tea, GLY: Green leaf yield plant−1 (g), PP: Number of plucking points in m−2, SBF: Spreading of bush frame (cm2), RP: Recovery days after pruning, NF: Number of flower plant−1, LB: Length of single bud (cm), SP: Survivability percentage of cuttings of each genotype
Mean values of 200 genotypes against 13 characters according to six clusters are presented in Table 1. GLY, PP, and SBF were found significantly higher while PI and DR were observed lower in Cluster-1 and Cluster-2. On the other hand, NP, SF, and QSB were noticed significantly higher in Cluster-3, Cluster-4, and Cluster-1.
3.3 Principal Component Analysis (PCA)
The first four principal components (PCs) having eigenvalue of more than one, were responsible for cumulatively 75.6% of total variations (Table 2). The first two components (PC 1 and PC 2) cumulatively described 56.9% of total variations (Table 2). The characters GLY, PP, PI, SBF, and DR mostly contributed to PC 1 which explained 38% of the total variation (Table 2 and Fig. 3a,c). These characteristics were mainly referred to as yield-contributing traits in the case of tea. On the other hand, the characters QSB, NP, and SF were indicated as quality contributing characters which were strongly associated with PC 2 (Fig. 3b,c). PC 2 accounted for 18.9% of total variation (Table 2 and Fig. 3c).
Figure 3: Principal component analysis (PCA) considering 13 traits of 200 tea genotypes. (a) Contributions of variables to PC 1, (b) Contributions of variables to PC 2. Red dashes are referred to as reference lines and the variables that lie above the reference lines affect the particular component most, (c) PCA-biplot showing the degree of association between the variables and clusters of genotypes. Descriptions of the variables: GLY: Green leaf yield plant−1 (g), PP: Number of plucking points in m−2, PI: Number of days of plucking interval, SBF: Spreading of bush frame (cm2), DR: Number of dormancy period, NP: Number of pubescence of single leaf bud, SF: Score of fermentation rate of green leaf, LB: Length of single bud (cm), QSB: Quality score of black tea, QSW: Quality score of white tea, RP: Recovery days after pruning, NF: Number of flowers plant−1, SP: Survivability percentage of cuttings of each genotype
A PCA-biplot was constructed where 200 genotypes are presented with six clusters along with 13 contributing characters of respective PCs (Fig. 3c). PC 1 was strongly positively associated with GLY, PP, and SBF while negatively with PI and DR. However, the variables QSB, NP, and SF were highly contributed to PC 2. The genotypes under Cluster-1 and Cluster-2 possessed more yield potentials, on the contrary, genotypes under Cluster-3 and Cluster-4 had more quality potentials (Fig. 3c).
The correlation matrix with the value of correlation coefficient (r) is demonstrated in Fig. 4 to understand the pattern and degree of relationship among the 13 characters. GLY were found to be related significantly, positively, and strongly with both PP (0.97) and SBF (0.90) while negatively with PI (−0.91) and DR (−0.86). It was also observed that PP had a positive strong correlation with SBF (0.85) but negative with DR (−0.82). PI possessed a positive association with DR (0.83) but negatively related with both PP (−0.89) and SBF (−0.89). No significant relationship was observed between the characters like GLY, NP, SF, and RP. So, it can be concluded that the yield potential of tea genotypes is mostly associated with GLY, PP, SBF, PI, and DR. On the other hand, QSB was significantly and positively related to both NP (0.88) and SF (0.72), while SF was strongly related to NP (0.68). QSB had no relationship of significance with NF, LB, and RP. So, it can be assumed that black tea quality was closely associated with QSB, SF, and NP.
Figure 4: Correlation matrix with correlation coefficient (r) values of 13 measured characters of 200 genotypes. Pearson’s correlation is displayed on a scale of −1 to 1 and the color gradient represents the extent of positive (blue) and negative (red) correlations among the characters. Non-significant, significant at 0.1%, significant at 1%, and significant at 5% level of probability are referred to as ns, *, **, and ***, respectively. Descriptions of the traits: GLY: Green leaf yield plant−1 (g), PP: Number of plucking points in m−2, PI: Number of days of plucking interval, SBF: Spreading of bush frame (cm2), DR: Number of dormancy period, NP: Number of pubescence of single leaf bud, SF: Score of fermentation rate of green leaf, LB: Length of single bud (cm), QSB: Quality score of black tea, QSW: Quality score of white tea, RP: Recovery days after pruning, NF: Number of flowers plant−1, SP: Survivability percentage of cuttings of each genotype
3.5 Genetic Diversity Analysis
The genotypic coefficient of variation (GCV), phenotypic coefficient of variation (PCV), the difference between PCV and GCV, heritability in a broad sense (h2b), genetic advance (GA) and genetic advance as percent of mean (GAM) are presented in Table 3. The range of GCV (%) and PCV (%) was found from 1.34% to 23.87% and 1.42% to 24.65%, respectively. Higher GCV values (more than 20%) were observed in SBF (23.87%) and DR (22.7%) as well as moderate (10% to 20%) in PI and LB. In the case of PCV%, a higher value was observed also in SBF (24.65%) and DR (23.04%) as well as moderate in PI (15.05%), LB (12.25%), NF (10.54%), and PP (10.33%). The difference between PCV (%) and GCV (%) revealed that PCV was consistently higher than GCV, indicating that all the characters were influenced by environmental factors. However, the lower differences illustrated that the influence of environment was found to a small extent, therefore the performances of all characters can be further improved by selection breeding.
Heritability is an important tool for a crop improvement program that calculates the influence of parents on the next generation and measures the rate of successful transfer of the characters to offspring. Heritability in broad sense (h2b) ranged from 39% to 99%. A lower value of h2b (less than 50%) was observed in RP, a medium value (50% to 75%) in NF, and the rest of the characters were found to have high heritability (Table 3). The high heritability of characters indicated that the genotypic expressions of the characters were less influenced by various environmental factors and can be important tools for crop improvement programs due to their higher domination to the next generations.
GA is the measurement of the genetic gain of offspring over parents through a selection process. Higher GA values (more than 20%) were observed in NP (147.5%) and NF (68.86%) while moderate values (10% to 20%) in GLY (19.71%) and PP (10.75%). On the other hand, high heritability with high GAM was found in SBF (47.59%), DR (46.06%), PI (24.16%), LB (22.65%), PP (16.13%), NF (15.02%), SF (12.58%), GLY (10.97%) and NP (10.09%) which implies that selection of genotypes by those characters could be beneficial because of successful transfer to next generation and higher performance over parents (Table 3).
3.6 Yield and Quality Contributing Characters with Best-Performed Genotypes
The most important yield contributing characters were identified as GLY, PP, PI, SBF, and DR from PCA (Fig. 3a,c) and correlation matrix (Fig. 4). Mean values of best performed (top ten) genotypes against important yield contributing characters (GLY, PP, PI, SBF, and DR) were presented in Tables 4 and A3. The genotypes G1 to G10 were high green leaf-yielding genotypes ranging from 200.72 g to 199.02 g plant−1 (Tables 4 and A3) where the lower value of GLY was 168.34 g plant−1 (Fig. 1 and Table A3). PP and SBF values of the top ten genotypes differed from 83 in m−2 (G4 and G2) to 79 in m−2 (G12) and 2.85 cm2 (G9) to 2.76 cm2 (G31) (Table 4). PI and DR both had a negative effect on GLY (Fig. 4), therefore lower value of PI and DR was responsible for higher GLY. Less number of days of PI was observed in G2 (7.25) while the top ten genotypes shared a similar number (2) of DR (Table 4).
The quality of black tea mostly depends on QSB, NP, and SF characters (Fig. 3b,c and Fig. 4). The quality contributing characters (QSB, NP, and SF) with mean value of best performed (top ten) genotypes are demonstrated in Table 5. A higher score of QSB was noticed in G28 (34.66) to G102 (33.81) (Table 5) while a lower score was observed in G24 (31.01) (Fig. 1 and Table A3). The pubescence number (NP) varied from G28 (1682) to G172 (1591) in better performed ten genotypes (Table 5). A remarkable score during fermentation (SF) was observed in G156 (7.85) to G4 (7.32) (Table 5) while lower score was found in G17, G32, G62, and G110 (6.01) (Fig. 1 and Table A3).
Hybridization and mass selection are the most commonly practiced breeding techniques to introduce new cultivars of tea. Naturally huge number of variations are found because tea is a highly cross-pollinated crop [46]. Any breeding operation with fewer parents can generate a result of the population having a narrow genetic base [47]. So, seeking and identifying the parents having longer distinct genetic relationships is very essential for a substantial breeding technique.
The yield of tea can be hampered by many abiotic factors, such as drought conditions, waterlogging stress, cold stress, etc. In recent times, waterlog due to excessive rainfall has been one of the major stresses responsible for the reduction of yield in many countries, such as India [48,49], Sri Lanka [50], and China [51]. In the current study, 200 genotypes were collected from naturally waterlogged areas of different tea estates to analyze the inherent variations present in them which could be useful in further breeding programs under waterlogged conditions.
Descriptive Statistical Analysis is very helpful in interpreting the pattern, behavior, and distribution of data sets by summarizing them graphically or numerically [52–54]. This analysis is very helpful in interpreting the pattern, behavior, and distribution of data sets by summarizing them graphically or numerically [55]. In the current study, GLY per plant ranged from 168.34 to 200.72 g where only six genotypes were above 200 g (Fig. 1 and Table A3) which can be a good indicator of being a high-yielding variety [24]. The green leaf yield of each plant is very important to determine the productivity of tea [56–58]. The number of plucking points (PP) per square meter area of tea bush ranged from 61 to 83 with a mean value of 66.67 (Fig. 1). Higher number of plucking points always leads to higher yield, therefore, the number of plucking points is always closely associated with yield in tea plants [59,60]. The number of days of plucking interval (PI) has a great impact on both yield and quality of tea which ranges from 7 days to 21 days (or even more) in different countries [61,62]. In this experiment, the average plucking interval of eight plucking rounds ranged from 7.25 days to 12.75 days with a mean value of 10.66 days. Spreading of the bush frame (SBF) is an essential criterion for tea yield, effective use of land as well as maintaining enough foliage for the physiological processes of plants [63]. On the other hand, the number of dormancy periods (DR) or ‘banjhi period’ is an endogenous rhythm of tea bushes that causes a continuous flushing cycle followed by several dormancy periods. In this study, SBF and DR ranged from 1.11 to 2.85 cm2 and 2 to 4, respectively. A number of pubescence of leaf bud (NP), a score of fermentation rate of the leaf (SF), and length of bud (LB) are generally associated with made tea quality. Generally longer bud contains numerous pubescence which leads to higher fermentation scores as well as better-made tea quality [29–31]. Here, the NP, SF, and LB ranged from 1258 to 1682, 6.01 to 7.85, and 2.13 to 4.92 cm with a mean value of 1461.45, 6.60, and 3.53 cm respectively. The quality score of black tea (QSB) and white tea (QSW) is the scoring of teas by organoleptic tasting or sensory method ranging from 31.01 to 34.66 and 31.01 to 33.89 which mainly varies with the different genotypes in many studies [64,65]. The lower and higher values of recovery days after pruning (RP) were 41 days and 48 days, which were uniformly distributed (Fig. 1) with an average value of 44.7 days. The flowering habit or number of flowers (NF) can be changed in many crops under different stress conditions [66,67]. The survivability percentage of cuttings (SP) is also an inherited character that can differ along with different varieties of tea [68]. In the present study, NF and SP ranged from 351 to 730 and 90.06% to 98.72% with a mean value of 458.37% and 92.91%, respectively. The survival percentage of cuttings in nursery beds is a prime criterion for selection as well as extension programs because vegetative propagation is the most commonly used technique for rapid multiplication and regeneration in tea [69].
Principal component analysis (PCA) is a sort of analysis where a huge number of interrelated data sets are transformed into different components or dimesons to explain the total variations [70]. In most of the cases, the first few principal components (PCs) reflect most of the variations having higher eigenvalue [71]. It was found that the first two components (PC 1 and PC 2) are responsible for 56.9% of total variations (Table 2). Out of thirteen characters, the characters like GLY, PP, PI, SBF, and DR were found above the reference line in PC 1 (Fig. 3a). So, it can be concluded that GLY, PP, PI, SBF, and DR were the main contributing characters for PC 1. The characters GLY, PP, PI, SBF, and DR were mainly associated with yield and, therefore, identified as yield-contributing characters. The shoot weight of different leaves is also mentioned as yield contributing characters in many studies [72–74]. On the other hand, characters like QSB, NP, and SF were noticed above the line in PC 2 (Fig. 3b). These characters played vital roles in PC 2 and were recognized as quality contributing characters. The quality scoring of black tea by sensory tasting (QSB), the number of pubescence (NP), and the scoring of fermentation time (SF) are also recognized as quality-related characteristics in many studies [75–77]. In a Principal Component Analysis-biplot (PCA-biplot), the distance of arrows from the center illustrates the intensity of association; a longer distance reflects higher contribution and vice versa [78]. From the PCA-biplot graph (Fig. 3c), it was observed that GLY, PP, PI, SBF, and DR pointed away from the center, thus largely affecting PC 1. Characters like GLY, PP, and SBF were found to affect positively, while DR and PI were negatively associated with PC 1. Both dormancy period (DR) and plucking interval (PI) adversely affect tea yield, which is in line with many findings [79–81]. On the other hand, QSB, NP, and SF possessed longer distances and were isolated as the most associated characters for PC 2. PCA-biplot (Fig. 3c) also indicates the possibility of having yield potential genotypes which resided under Cluster-1 and Cluster-2. Again, Cluster-1, Cluster-3, and Cluster-4 possessed the genotypes which could be more quality potential (Fig. 3c).
A correlation matrix was developed to measure the pattern, type, and intensity of the variables to each other. The yield potential of the genotypes (GLY) is positively related to PP and SBF while negatively associated with PI and DR. On the other hand, the quality of black tea (QSB) relied on NP and SF (Fig. 3a–c and Fig. 4). From both PCA-biplot and correlation matrix it can be decided that, yield and quality potentials of tea germplasms strongly associated with GLY, PP, PI, SBF, DR and QSB, NP, SF characters, respectively, which is a sort of agreement with various studies [72,82–84].
Before conducting any crop improvement program through a plant breeding strategy, it is a prime need to exploit genetic variations of characters over the existing population. Genetic diversity analysis is a proper guideline to point out the variations and effectiveness of selected traits [85]. Any trait is a cumulative expression of genetic and environmental effects. Diversity analysis is also used to calculate the extent of environmental effects and genetic effects on the characters. So, genetic variability and diversity analysis of characters are preconditions of a successful breeding program. In this corresponding experiment, the PCV of all the characters was higher than GCV and the differentiations were very low, implying that traits were less influenced by the environment (Table 3). Higher PCV values were also observed in many crops, like rice [86], wheat [87], maize [88], etc., with different characters. All the characters were except RP were highly to moderate heritable which found in line with Fig. 1 (uniformly distributed). High heritability with high GAM was found in SBF, DR, PI, LB, PP, NF, SF, GLY, and NP which indicates that selection breeding with these characters will be fruitful for further research. So, it can be concluded that for conducting a crop improvement program, selection based on GLY, PP, PI, SBF, DR, QSB, NP, and SF could have an immense effect on better yield and quality. A similar sort of interpretation was also made from PCA analysis (Fig. 3a–c). Medium GCV and PCV were observed in the number of shoots per bush while high heritability was found in several shoots (99%), bush diameter (97%), recovery days from pruning (88%), and green leaf yield (84%) in some tea genotypes of Southwestern Ethiopia [89].
Cluster analysis with a dendrogram is generally used to classify the variables within numerous clusters based on their relationship and normalized value [90]. Here, 13 measured traits were categorized into two groups while 200 genotypes were divided into six clusters. Six clusters of genotypes indicated that a large number of variations were presented among the genotypes. Cluster-1 and Cluster-2 possessed the genotypes related to high yield potentials characters (GLY, PP, PI, SBF, and DR) while Cluster-3, Cluster-4, and Cluster-1 carried the genotypes with high black tea quality traits like QSB, NP and SF (Table 1 and Fig. 2). This result was found to be similar with the interpretation of PCA-biplot graph (Fig. 3c). Several numbers of clustering also found in different studies [91–94].
Waterlogging has become one of the major abiotic stressors limiting yield and quality in many shrub and tree species, such as peach, coconut, cotton, coffee, tea, etc. [95–99]. When plants are subjected to waterlogging conditions, the oxygen supply in their roots is reduced and the aerial influx of CO2 is prevented, generating the reactive oxygen species (ROS) and also directly causing stomatal closure hindering respiration and photosynthesis which ultimately affects growth and yield [100,101]. To minimize yield losses, it is crucial to develop waterlogging-tolerant crop varieties with sustainable and feasible breeding strategies to combat waterlogging conditions [102]. To conduct any long-term breeding program, the selection and collection of germplasms of desired traits among a wide range of populations is a prerequisite step. In the present experiment, 200 genotypes were collected from naturally waterlogged areas of different tea estates under 13 parameters. Out of 200 genotypes, the best-performed (top ten) yield and quality potential genotypes were identified through variance analysis with multiple comparisons (Tables 4 and 5). P/LAL/08/23 (G1), P/AFN/11/35 (G2), P/OTI/31 (G3), P/AFN/11/46 (G4), P/LAL/08/62 (G5), P/LAL/09/116 (G6), P/AFN/13/90 (G7), P/CHM/18/74 (G8), P/MPR/12/58 (G9) and P/AFN/13/97 (G10) were the genotypes with higher GLY values. These ten genotypes were performed with satisfaction in other yield contributing characters like PP, PI, SBF, and DR. All these genotypes were from Cluster-1 and Cluster-2 which was a match to the results of cluster heatmap with dendrogram and PCA-biplot (Figs. 2 and 3c) and these ten genotypes were identified for future breeding interest. In the case of black tea quality, the top ten genotypes were also sorted out by the performance of quality contributing characters like QSB, NP, and SF. But amongst 200 genotypes, only one genotype P/AFN/11/31 (G28) was given excellent quality liquor (quality score of 34.66 out of 50) (Tables 5 and A3). QSB, NP, and SF were high heritable characters (Table 3) that denote the consistency of tea quality generation after generation. So, P/AFN/11/31 (G28) is the only genotype with higher quality potential from Cluster-1 (Figs. 2 and 3c) that can be selected for further research programs. So, finally it can be concluded that total eleven (11) genotypes like P/LAL/08/23, P/AFN/11/35, P/OTI/31, P/AFN/11/46, P/LAL/08/62, P/LAL/09/116, P/AFN/13/90, P/CHM/18/74, P/MPR/12/58, P/AFN/13/97 and P/AFN/11/31 were found promising with higher yield and quality potential which can be used for further research and breeding program for quantify their waterlogging tolerance ability.
Phenotypic selection breeding of tea is a conventional technique used to improve desired traits like yield, quality, or inducing tolerance. Among the 13 measured attributes of the current experiment, traits like GLY, DR, PI, SBF, PP, NF, NP, LB, SF, and QSB were highly heritable characters suggesting that these traits should be taken into consideration as selection criteria for breeding programs of tea. The yield of tea (GLY) was positively related to PP, and SBF but negatively to PI and DR, whereas quality of tea (QSB) highly relied on NP and SF. Finally, eleven genotypes (P/LAL/08/23, P/AFN/11/35, P/OTI/31, P/AFN/11/46, P/LAL/08/62, P/LAL/09/116, P/AFN/13/90, P/CHM/18/74, P/MPR/12/58, P/AFN/13/97 and P/AFN/11/31) were identified considering yield and quality potentials which might be taken for further investigation facilitating future crop improvement program for waterlogging tolerance.
Acknowledgement: The authors extend their appreciation to Director of Bangladesh Tea Research Institute for overall cooperation and Bangladesh Agricultural University Research System authority for partial funding (article processing fee) of this publication.
Funding Statement: Author Md. Riyadh Arefin received the grant of National Science and Technology from the Ministry of Science and Technology, Government of the People’s Republic of Bangladesh (https://most.gov.bd/) (accessed on 27 November 2024) for conducting this experiment.
Author Contributions: The authors confirm contribution to the paper as follows: study conception and design: Md. Riyadh Arefin and A. K. M. Golam Sarwar; data collection: Md. Riyadh Arefin; analysis and interpretation of results: Md. Sabibul Haque, Md. Riyadh Arefin and A. K. M. Golam Sarwar; draft manuscript preparation: Md. Riyadh Arefin. All authors reviewed the results and approved the final version of the manuscript.
Availability of Data and Materials: The authors confirm that the data supporting the findings of this study are available within the article.
Ethics Approval: Not applicable.
Conflicts of Interest: The authors declare no conflicts of interest to report regarding the present study.
References
1. Khan N, Mukhtar H. Tea and health: studies in humans. Curr Pharm Des. 2013;19(34):6141–7. doi:10.2174/1381612811319340008. [Google Scholar] [PubMed] [CrossRef]
2. Tomita I. Efficacy of tea in human health. UK: CABI; 2017. p. 1–8. doi:10.1079/9781786392398.0000. [Google Scholar] [CrossRef]
3. Zohora KFT, Arefin MR. Tea and tea product diversification: a review. Turkish JAF Sci Tech. 2022;10(12):2334–53. doi:10.24925/turjaf.v10i12.2334-2353.5280. [Google Scholar] [CrossRef]
4. Bag S, Mondal A, Majumder A, Banik A. Tea and its phytochemicals: hidden health benefits & modulation of signaling cascade by phytochemicals. Food Chem. 2021;371:131098. doi:10.1016/j.foodchem.2021.131098. [Google Scholar] [PubMed] [CrossRef]
5. Raza SMS. Prospects and challenges of tea industry in Bangladesh. Cost Manage. 2019;47(2):31–5. [Google Scholar]
6. Arefin MR, Hossain MI. Present status and future prospects of tea production and research on varietal improvement in Bangladesh. Turkish JAF Sci Tech. 2022;10(12):2324–33. doi:10.24925/turjaf.v10i12.2324-2333.5259. [Google Scholar] [CrossRef]
7. Islam MA. Role of Bangladesh tea board on enhancing productivity and quality of Bangladesh tea. Tea J Bangladesh. 2024;50:1–17. doi:10.5281/zenodo.11236745. [Google Scholar] [CrossRef]
8. Islam GMR, Iqbal M, Quddus K, Ali M. Present status and future needs of tea industry in Bangladesh. Proc Pak Acad Sci. 2005;42(4):304–14. [Google Scholar]
9. Ahmad I, Hossain MA. Present status of Bangladesh tea sector in respect of world tea: an overview. J Appl Sci Technol. 2013;9(1):116–28. [Google Scholar]
10. Walne CH, Reddy KR. Developing functional relationships between soil waterlogging and corn shoot and root growth and development. Plants. 2021;10(10):2095. doi:10.3390/plants10102095. [Google Scholar] [PubMed] [CrossRef]
11. Zhou XB, Zhang L, Xiong H, Jiang P, Zhu YC, Guo XY, et al. Effects of submergence stress on growth characteristics and yield formation of mid-season hybrid rice combinations. Chin Rice. 2014;20(3):23. [Google Scholar]
12. Ding J, Liang P, Wu P, Zhu M, Li C, Zhu X, et al. Effects of waterlogging on grain yield and associated traits of historic wheat cultivars in the middle and lower reaches of the Yangtze River, China. Field Crops Res. 2020;246:107695. doi:10.1016/j.fcr.2019.107695. [Google Scholar] [CrossRef]
13. Huang C, Gao Y, Qin A, Liu Z, Zhao B, Ning D, et al. Effects of waterlogging at different stages and durations on maize growth and grain yields. Agric Water Manage. 2022;261:107334. doi:10.1016/j.agwat.2021.107334. [Google Scholar] [CrossRef]
14. Barman T, Baruah U, Deka D, Borah D, Lahon T, Saikia J. Selection and evaluation of waterlogging tolerant tea genotypes for plantation in marginal land. Two Bud. 2011;58:33–8. [Google Scholar]
15. Ali N, Hussain I, Ali S, Khan NU, Hussain I. Multivariate analysis for various quantitative traits in wheat advanced lines. Saudi J Biol Sci. 2021;28(1):347–52. doi:10.1016/j.sjbs.2020.10.011. [Google Scholar] [PubMed] [CrossRef]
16. Naggar A, Shafik MM, Musa M, Younis A, Anany AH. Genetic variability of maize hybrids and populations and interrelationships among grain yield and its related traits under drought and low N using multivariate analysis. Asian J Biochem Genet Mol Biol. 2020:26–44. doi:10.9734/ajbgmb/2020/v4i230102. [Google Scholar] [CrossRef]
17. Baye A, Berihun B, Bantayehu M, Derebe B. Genotypic and phenotypic correlation and path coefficient analysis for yield and yield-related traits in advanced bread wheat (Triticum aestivum L.) lines. Tejada Moral M, editor. Cogent Food Agric. 2020;6(1):1752603. doi:10.1080/23311932.2020.1752603. [Google Scholar] [CrossRef]
18. Aytac Z, Kınacı G. Genetic variability and association studies of some quantitative characters in winter rapeseed (Brassica napus L.). Afr J Biotechnol. 2009;8(15):3547–54. [Google Scholar]
19. BTRI (Bangladesh Tea Research Institute). Annual report 2021. Sreemangal, Bangladesh: BTRI; 2022. Available from: https://btri.portal.gov.bd/site/page/fcf7da36-0745-4d3e-b34c-76c4534175cb/. [Accessed 2024]. [Google Scholar]
20. BTRI (Bangladesh Tea Research Institute). Annual report 2023. Sreemangal, Bangladesh: BTRI; 2024. Available from: https://btri.portal.gov.bd/site/page/fcf7da36-0745-4d3e-b34c-76c4534175cb/-. [Accessed 2024]. [Google Scholar]
21. BTB (Bangladesh Tea Board). Annual review of Bangladesh Tea, 2023–2024. Chattogram, Bangladesh: BTB; 2024. Available from: https://teaboard.gov.bd/sites/default/files/files/teaboard.portal.gov.bd/publications/c94f360c_7d8a_4f41_a7ae_6f19c0dacacc/2024-10-14-09-34-425b28342b7d0d811761d6cfb44138b6.pdf. [Accessed 2024]. [Google Scholar]
22. Alam AFMB. Bangladeshe Khudro Porjaye Cha Chas Nirdeshika. Sreemangal, Bangladesh: Bangladesh Tea Research Institute; 2003 (In Bangla). [Google Scholar]
23. Hossain MI, Ahmed M, Mamun MSA, Sarwar AKMG. Yield and quality performances of four tea test clones. J Bangladesh Agril Univ. 2012;10(1):33–8. doi:10.3329/jbau.v10i1.12036. [Google Scholar] [CrossRef]
24. Handique A, Manivel L. Selection criteria for drought tolerance in tea. Assam Rev Tea. 1990;79:18–21. [Google Scholar]
25. Ahmad F, Hamid FS, Sarwar S, Waheed A, Aslam S, Islam S, et al. Effect of different pruning times on the yield of tea (Camellia sinensis L.) under the climatic conditions of Mansehra-Pakistan. Sarhad J Agric. 2014;30(3):305–9. [Google Scholar]
26. Aaqil M, Peng C, Kamal A, Nawaz T, Zhang F, Gong J. Tea harvesting and processing techniques and its effect on phytochemical profile and final quality of black tea: a review. Foods. 2023;12(24):4467. doi:10.3390/foods12244467. [Google Scholar] [PubMed] [CrossRef]
27. Singh ID. Selection and testing field performance of candidate tea clones in India. Int J Tea Sci. 2006;5(1):1–12. [Google Scholar]
28. Krishnaraj T, Gajjeraman P, Palanisamy S, Chandrabose SRS, Mandal AKA. Identification of differentially expressed genes in dormant (banjhi) bud of tea (Camellia sinensis (L.) O. Kuntze) using subtractive hybridization approach. Plant Physiol Biochem. 2011;49(6):565–71. doi:10.1016/j.plaphy.2011.03.011. [Google Scholar] [PubMed] [CrossRef]
29. Zhu M, Li N, Zhao M, Yu WL, Wu J. Metabolomic profiling delineate taste qualities of tea leaf pubescence. Food Res Int. 2017;94:36–44. doi:10.1016/j.foodres.2017.01.026. [Google Scholar] [PubMed] [CrossRef]
30. Waheed A, Hamid FS, Ahmad N. Criteria used in selection of locally best tea bushes. J Biol Sci. 2000;1(1):21–3. doi:10.3923/jbs.2001.21.23. [Google Scholar] [CrossRef]
31. Hossain MI, Arefin MR. Development of sustainable manufacturing protocol of Golden Tips Tea with different clonal combinations in respect of Bangladesh. Tea J Bangladesh. 2024;50(34–40). doi:10.5281/zenodo.11237330. [Google Scholar] [CrossRef]
32. Khan M, Islam MA, Uddin R, Kalam MA, Baishakh NN, Barua P, et al. Biochemical investigation of Bangladeshi black tea and their correlation to organoleptic quality evaluation. Heliyon. 2023;9(6):16802–2. doi:10.1016/j.heliyon.2023.e16802. [Google Scholar] [PubMed] [CrossRef]
33. Hossain MI, Arefin MR. Developing quality white tea (artisan tea) manufacturing protocol by using different clone with their different combinations in respect of Bangladesh. Eur Aca Res. 2018;6(3):1352–8. [Google Scholar]
34. Sheard J. Quantitative data analysis. In: Williamson K, Johanson G, editors. Research methods: information, systems, and contexts. 2nd ed. Cambridge: Elsevier; 2018. p. 429–52. doi:10.1016/B978-0-08-102220-7.00018-2 [Google Scholar] [CrossRef]
35. Ramachandran KM, Tsokos CP. Mathematical statistics with applications. London: Elsevier Academic Press; 2009. Available from: https://opac.feb.uinjkt.ac.id/repository/b0790fe81391c381d03e15e83778ca4d.pdf. [Accessed 2024]. [Google Scholar]
36. Xin YH, Wu YX, Qiao B, Su L, Xie SQ, Ling P. Evaluation on the phenotypic diversity of Calamansi (Citrus microcarpa) germplasm in Hainan island. Sci Rep. 2022;12:371. doi:10.1038/s41598-021-03775-x. [Google Scholar] [PubMed] [CrossRef]
37. Dobrinas S, Soceanu A, Popescu V, Popovici IC, Jitariu D. Relationship between total phenolic content, antioxidant capacity, Fe and Cu content from tea plant samples at different brewing times. Processes. 2021;9(8):1311. doi:10.3390/pr9081311. [Google Scholar] [CrossRef]
38. Braun S. Correlation functions. In: Ewins DJ, Braun SG, Rao SS, editors. Encyclopedia of vibration. London: Elsevier Academic Press; 2001. p. 294–302. [Google Scholar]
39. Hauke J, Kossowski T. Comparison of values of Pearson’s and Spearman’s correlation coefficients on the same sets of data. Quaest Geogr. 2011;30(2):87–93. doi:10.2478/v10117-011-0021-1. [Google Scholar] [CrossRef]
40. Huqe MAS, Haque MS, Sagar A, Uddin MN, Hossain MA, Hossain AZ, et al. Characterization of maize hybrids (Zea mays L.) for detecting salt tolerance based on morpho-physiological characteristics, ion accumulation and genetic variability at early vegetative stage. Plants. 2021;10(11):2549. doi:10.3390/plants10112549. [Google Scholar] [PubMed] [CrossRef]
41. Zaki HEM, Radwan KSA. Estimates of genotypic and phenotypic variance, heritability, and genetic advance of horticultural traits in developed crosses of cowpea (Vigna unguiculata [L.] Walp.). Front Plant Sci. 2022;13:987985. doi:10.3389/fpls.2022.987985. [Google Scholar] [PubMed] [CrossRef]
42. Holland JB, Nyquist WE, Cervantes-Martínez CT. Estimating and interpreting heritability for plant breeding: an update. In: Jules J, editor. Plant Breed Rev. New York: John Wiley & Sons Inc.; 2003. p. 9–112. [Google Scholar]
43. Chanda SC, Haque MS, Sarwar AKMG, Nuruzzaman M, Hossain MA. Genetic dissection in morphological traits of Sesbania genotypes. Proc Nat Acad Sci India Sect B: Biol Sci. 2020;90(5):1153–64. doi:10.1007/s40011-020-01185-z. [Google Scholar] [CrossRef]
44. Demeke B, Dejene T, Abebe D. Genetic variability, heritability, and genetic advance of morphological, yield related and quality traits in upland rice (Oryza sativa L.) genotypes at Pawe, northwestern Ethiopia. Cogent Food Agric. 2022;9(1):2157099. doi:10.1080/23311932.2022.2157099. [Google Scholar] [CrossRef]
45. Shivaramegowda KD, Krishnan A, Jayaramu YK, Kumar V, Yashoda, Koh HJ. Genotypic variation among okra (Abelmoschus esculentus (L.) Moench) germplasms in South India. Plant Breed Biotech. 2016;4(2):234–41. doi:10.9787/PBB.2016.4.2.234. [Google Scholar] [CrossRef]
46. Tuwei G, Corley RHV. Pollination methods and inbreeding of tea. Tea. 2017;38(1):3–8. [Google Scholar]
47. Yao MZ, Chen L. Tea germplasm and breeding in China. In: Chen L, Apostolides Z, Chen ZM, editors. Global tea breeding: achievements, challenges and perspectives. Germany: Zhejiang University Press and Springer-Verlag GmbH; 2012. p. 13–68. [Google Scholar]
48. Duncan JMA, Saikia SD, Gupta N, Biggs EM. Observing climate impacts on tea yield in Assam, India. Appl Geogr. 2016;77:64–71. doi:10.1016/j.apgeog.2016.10.004. [Google Scholar] [CrossRef]
49. Dutta R, Smaling EMA, Bhagat RM, Tolpekin VA, Stein A. Analysis of factors that determine tea productivity in Northeastern India: a combined statistical and modelling approach. Exp Agric. 2011;48(1):64–84. doi:10.1017/S0014479711000834. [Google Scholar] [CrossRef]
50. Wijeratne MA, Anandacoomaraswamy A, Amarathunga MKSLD, Ratnasiri J, Basnayake BRSB, Kalra N. Assessment of impact of climate change on productivity of tea (Camellia sinensis L.) plantations in Sri Lanka. J Natl Sci Found Sri. 2007;35(2):119. doi:10.4038/jnsfsr.v35i2.3676. [Google Scholar] [CrossRef]
51. Lou W, Sun K, Zhao Y, Deng S, Zhou Z. Impact of climate change on inter-annual variation in tea plant output in Zhejiang, China. Int J Climatol. 2020;41(S1):E479–90. doi:10.1002/joc.6700. [Google Scholar] [CrossRef]
52. Cooksey RW. Descriptive statistics for summarising data. In: Illustrating statistical procedures: finding meaning in quantitative data. Singapore: Springer; 2020. p. 61–139. doi:10.1007/978-981-15-2537-7_5. [Google Scholar] [CrossRef]
53. Lee J. Statistics, descriptive. In: Kitchin R, Thrift N, editors. International encyclopedia of human geography. Amsterdam: Elsevier Science; 2009. p. 422–8. doi:10.1016/B978-008044910-4.00534-4. [Google Scholar] [CrossRef]
54. Kaur P, Stoltzfus J, Yellapu V. Descriptive statistics. Int J Acad Med. 2018;4(1):60–3. doi:10.4103/ijam.ijam_7_18. [Google Scholar] [CrossRef]
55. Łukasik S, Kowalski PA. Clustering with nature-inspired metaheuristics. In: Yang XS, editor. Nature-inspired computation and swarm intelligence: algorithms, theory and applications. Cambridge: Academic Press; 2020. p. 165–78. doi:10.1016/b978-0-12-819714-1.00021-x. [Google Scholar] [CrossRef]
56. Chimoita EL, Maina GD, Olila DO, Onyango JPG. The role of farmer field schools approach in improving tea production among smallholding in Kenya. Univers J Agric. 2015;3(1):4–10. doi:10.13189/ujar.2015.030102. [Google Scholar] [CrossRef]
57. Benti T, Debela A, Bekele Y, Suleman S. Effect of seasonal variation on yield and leaf quality of tea clone (Camellia sinensis (L.) O. Kuntze) in South West Ethiopia. Heliyon. 2023;9(3):e14051. doi:10.1016/j.heliyon.2023.e14051. [Google Scholar] [PubMed] [CrossRef]
58. Hossain MI. Characterization of germplasm and screening of drought tolerant variety of tea (Ph.D. Thesis). Bangladesh Agricultural University: Mymensingh, Bangladesh; 2019. [Google Scholar]
59. Amiri ME, Asil MH. Determination of optimum harvestable length of shoots in tea (Camellia sinensis L.) based on the current shoot growth, rather than interval plucking. J Agric Food Environ. 2007;5(2):122–4. [Google Scholar]
60. Yan L, Wu KH, Lin J, Xu X, Zhang J, Zhao X, et al. Identification and picking point positioning of tender tea shoots based on MR3P-TS model. Front Plant Sci. 2022;13:1–12. doi:10.3389/fpls.2022.962391. [Google Scholar] [PubMed] [CrossRef]
61. Okal AW, Owuor PO, Kamau DM, Onyango L. Effects of production locations and plucking intervals on clonal tea fatty acids levels in the Kenya Highlands. Food Sci Technol Res. 2012;18(3):351–6. [Google Scholar]
62. Sylvestre H, Jacqueline N, Alphonsine M, Olive T. Impact of plucking interval on tea productivity and green leaf quality. Int J Soil Crop Sci. 2014;2(10):68–072. [Google Scholar]
63. Barman T. Tea bush health determination and yield estimation (Master’s Thesis). San Jose State University: San Jose, CA, USA; 2008. [Google Scholar]
64. Hossain MI, Rahman MS. Long term performance of different clones for yield and quality traits in tea. J Sci Technol. 2013;11:138–43. [Google Scholar]
65. Adnan M, Ahmad A, Ahmed A, Khalid N, Hayat I, Ahmed I. Chemical composition and sensory evaluation of tea (Camellia sinensis) commercialized in Pakistan. Pak J Bot. 2013;45(3):901–7. [Google Scholar]
66. Wada KC, Takeno K. Stress-induced flowering. Plant Signal Behav. 2010;5(8):944–7. doi:10.4161/psb.5.8.11826. [Google Scholar] [PubMed] [CrossRef]
67. Qiu D, Shavrukov Y, Chen J, Chen LY. Editorial: stress-induced flowering in plants. Front Plant Sci. 2023;14:1338150. doi:10.3389/fpls.2023.1338150. [Google Scholar] [PubMed] [CrossRef]
68. Teshome M, Endale T, Tesfaye S, Melaku A. Effects of various rooting media on survival and growth of tea (Camellia sinensis L.) stem cuttings at Jimma. J Biol Agric Healthc. 2016;6(3):149–56. [Google Scholar]
69. Awan WA, Badshah N, Waheed A. Performance of various sizes of nodal tea cuttings of Camellia sinensis L. as affected by different timings of planting. Pak J Biol Sci. 2000;3(6):1008–10. [Google Scholar]
70. Roessner U, Nahid A, Chapman B, Hunter A, Bellgard M. Metabolomics—the combination of analytical biochemistry, biology, and informatics. In: Moo-Young M, editor. Comprehensive biotechnology. 2nd ed. Amsterdam: Elsevier Inc.; 2011. p. 447–59. doi:10.1016/B978-0-08-088504-9.00052-0. [Google Scholar] [CrossRef]
71. Nievergelt CM, Maihofer AX, Shekhtman T, Libiger O, Wang X, Kidd KK, et al. Inference of human continental origin and admixture proportions using a highly discriminative ancestry informative 41-SNP panel. Investig Genet. 2013;4(1):13. doi:10.1186/2041-2223-4-13. [Google Scholar] [PubMed] [CrossRef]
72. Neranjana TBKH, Ranatunga MAB, Kottawa-Arachchi JD, Ranaweera KK. Correlation and path coefficient analysis of yield and yield related traits in tea: implications on cultivar selection. Sri Lankan J Tea Sci. 2014;79(1/2):1–11. [Google Scholar]
73. Kottawa-Arachchi J, Gunasekare MTK, Ranatunga MAB. Variations in yield related traits of tea (Camellia sinensis L.) germplasm accessions and its utilization in tea breeding programme. Sri Lankan J Tea Sci. 2017;80(1/2):55–66. [Google Scholar]
74. Long L, Shi Y, Ma L, Ruan J. Characterization of young shoot population, yield, and nitrogen demands of tea (Camellia sinensis L.) harvested under different standards. Horticulturae. 2022;8(4):275–5. doi:10.3390/horticulturae8040275. [Google Scholar] [CrossRef]
75. Thuvarakia B, Ranatunga MAB, Kottawa-Arachchi JD, Sumanasinghe VA. Characterization of new tea (Camellia sinensis L.) hybrid progenies based on morphological traits. Int J Tea Sci. 2017;13(1&2):1–9. [Google Scholar]
76. Wang L, Fan S, Wang X, Wang X, Yan X, Shan D, et al. Physicochemical aspects and sensory profiles as various potential factors for comprehensive quality assessment of Nü-Er-Cha produced from Rhamnus heterophylla Oliv. Molecules. 2019;24(18):3211. doi:10.3390/molecules24183211. [Google Scholar] [PubMed] [CrossRef]
77. Muthumani T, Kumar RSS. Influence of fermentation time on the development of compounds responsible for quality in black tea. Food Chem. 2007;101(1):98–102. doi:10.1016/j.foodchem.2006.01.008. [Google Scholar] [CrossRef]
78. Sarwar AKMG, Haque MS, Haque ME, Hossain MA, Azam MG, Uddin MN, et al. Agro-morphological characterization and genetic dissection of linseed (Linum usitatissimum L.) genotypes. Phyton. 2022;91(7):1–23. doi:10.32604/phyton.2022.021069. [Google Scholar] [CrossRef]
79. Vyas D, Kumar S, Ahuja PS. Tea (Camellia sinensis) clones with shorter periods of winter dormancy exhibit lower accumulation of reactive oxygen species. Tree Physiol. 2007;27(9):1253–9. doi:10.1093/treephys/27.9.1253. [Google Scholar] [PubMed] [CrossRef]
80. Vyas D, Kumar S. Tea (Camellia sinensis (L.) O. Kuntze) clone with lower period of winter dormancy exhibits lesser cellular damage in response to low temperature. Plant Physiol Biochem. 2005;43(4):383–8. doi:10.1016/j.plaphy.2005.02.016. [Google Scholar] [PubMed] [CrossRef]
81. Sud RK, Singh CM, Jain NK. Economics of different plucking intervals in China hybrid tea (Camellia sinensis) in Himachal Pradesh. J Plant Crop. 1996;24(1):29–33. [Google Scholar]
82. Kamunya S, Wachira F, Pathak R, Muoki C, Kanyiri W, Ronno W, et al. Quantitative genetic parameters in tea (Camellia sinensis (L.) O. KuntzeI. Combining abilities for yield, drought tolerance and quality traits. Int J Plant Breed Genet. 2020;7(1):1–9. [Google Scholar]
83. Wang X, Han C, Wu W, Xu J, Zhang Q, Chen M, et al. Fundamental understanding of tea growth and modeling of precise tea shoot picking based on 3-D coordinate instrument. Process. 2021;9(6):1059. doi:10.3390/pr9061059. [Google Scholar] [CrossRef]
84. Phong NH, Pongnak W, Soytong K, Poeaim S, Poeaim A. Diversity of tea (Camellia sinensis) grown in Vietnam based on morphological characteristics and inter-Primer Binding Sites (iPBS) Marker. Int J Agric Biol. 2016;18(2):385–92. doi:10.17957/ijab/15.0100. [Google Scholar] [CrossRef]
85. Kumar S, Ram S, Chakraborty M, Ahmad E, Verma N, Lal HC, et al. Role of genetic variability for seed yield and its attributes in linseed (Linum usitatissimum L.) improvement. J Pharmacog Phytother. 2019;8(2S):266–8. [Google Scholar]
86. Chacko A, Jayalekshmy VG, Shahiba AM. Studies on PCV, GCV, heritability, and genetic advance in rice genotypes for yield and yield components. Int J Plant Soil Sci. 2023;35(16):324–30. doi:10.9734/ijpss/2023/v35i163266. [Google Scholar] [CrossRef]
87. Singh MK, Sharma PK, Tyagi BS, Singh G. Genetic analysis for morphological traits and protein content in bread wheat (Triticum aestivum L.) under normal and heat stress environments. Indian J Genet. 2013;73(3):320. doi:10.5958/j.0975-6906.73.3.047. [Google Scholar] [CrossRef]
88. Pranay G, Shashibhushan D, Rani KJ, Bhadru D, Kumar CVS. Genotype and phenotype coefficient of variance in maize (Zea mays L.). Int J Environ Clim. 2022;12(12):1119–32. doi:10.9734/ijecc/2022/v12i121550. [Google Scholar] [CrossRef]
89. Zakir M, Addisu M. Estimation of genetic variance on tea (Camellia sinensis (L.) O. Kuntze) clones based on morphological markers in Southwestern Ethiopia. Int J Plant Breed. 2020;7(1):641–7. [Google Scholar]
90. Haque MS, Hossain KS, Baroi A, Alamery S, Attia KA, Hafez YM, et al. Foliar application of abscisic acid and glycine betaine induces tolerance to water scarcity in wheat. Plant Growth Regul. 2024. doi:10.1007/s10725-024-01190-6. [Google Scholar] [CrossRef]
91. Zhang C, Li J, Zhu Z, Zhang Y, Zhao L, Wang C. Cluster analysis on japonica rice (Oryza sativa L.) with good eating quality based on SSR markers and phenotypic traits. Rice Sci. 2010;17(2):111–21. doi:10.1016/S1672-6308(08)60113-4. [Google Scholar] [CrossRef]
92. Mukul MM, Akter N. Morpho-anatomical variability, principal component analysis and euclidean clustering of tossa jute (Corchorus olitorius L.). Heliyon. 2021;7(5):e07042. doi:10.1016/j.heliyon.2021.e07042. [Google Scholar] [PubMed] [CrossRef]
93. Naresh B, Samarendra H, Krishnappa R, Dwipendra T, Rumi N, Debnath S. Comparative stress physiological analysis of Aluminium stress tolerance of indigenous maize (Zea mays L.) cultivars of Eastern Himalaya. Heliyon. 2024;10(11):e31570. doi:10.1016/j.heliyon.2024.e31570. [Google Scholar] [PubMed] [CrossRef]
94. Guo CF, Sun Y, Chen CS, Chen RB, Zhang MQ. Comparison and cluster analysis of photosynthetic characters and water use efficiency in tea (Camellia sinensis) cultivars. Acta Agron Sin. 2008;34(10):1797–804. doi:10.1016/s1875-2780(09)60010-9. [Google Scholar] [CrossRef]
95. Insausti P, Gorjón S. Floods affect physiological and growth variables of peach trees (Prunus persica (L.) Batschas well as the postharvest behavior of fruits. Sci Hortic. 2013;152:56–60. doi:10.1016/j.scienta.2013.01.005. [Google Scholar] [CrossRef]
96. de Medeiros WJF, de Oliveira FÍF, de Lacerda CF, de Sousa CHC, Cavalcante LF, da Silva ARA, et al. Isolated and combined effects of soil salinity and waterlogging in seedlings of Green Dwarf coconut. Semin Cienc Agrar. 2018;39(4):1459. doi:10.5433/1679-0359.2018v39n4p1459. [Google Scholar] [CrossRef]
97. Zhang Y, Liu G, Dong H, Li C. Waterlogging stress in cotton: damage, adaptability, alleviation strategies, and mechanisms. Crop J. 2021;9(2):257–70. doi:10.1016/j.cj.2020.08.005. [Google Scholar] [CrossRef]
98. de Silveira HRO, de Santos MO, Alves JD, de Souza KRD, Andrade CA, Alves RGM. Growth effects of water excess on coffee seedlings (Coffea arabica L.). Acta Sci Agron. 2014;36(2):211–1. doi:10.4025/actasciagron.v36i2.17557. [Google Scholar] [CrossRef]
99. Rao NR, Kapoor M, Sharma N, Venkateswarlu K. Yield prediction and waterlogging assessment for tea plantation land using satellite image-based techniques. Int J Remote Sens. 2007;28(7):1561–76. doi:10.1080/01431160600904980. [Google Scholar] [CrossRef]
100. Azrai M, Efendi R, Muliadi A, Aqil M, Suwarti N, Zainuddin B, et al. Genotype by environment interaction on tropical maize hybrids under normal irrigation and waterlogging conditions. Front Sustain Food Syst. 2022;6:913211. doi:10.3389/fsufs.2022.913211. [Google Scholar] [CrossRef]
101. Zhang Y, Li Y, Liang T, Yuan Y, Li Z, Xu S, et al. Field-grown cotton shows genotypic variation in agronomic and physiological responses to waterlogging. Field Crops Res. 2023;302:109067. doi:10.1016/j.fcr.2023.109067. [Google Scholar] [CrossRef]
102. Hakim M, Hussain A, Alam I, Khoso MA, Ali Q, Liu F. Waterlogging stress in plants: unraveling the mechanisms and impacts on growth, development, and productivity. Environ Exp Bot. 2024:105824–4. doi:10.1016/j.envexpbot.2024.105824. [Google Scholar] [CrossRef]
Appendix A
Cite This Article
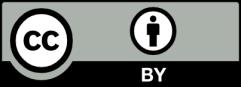
This work is licensed under a Creative Commons Attribution 4.0 International License , which permits unrestricted use, distribution, and reproduction in any medium, provided the original work is properly cited.