Open Access
ARTICLE
Pre-breeding in Rice Development: Phenotypic-Genotypic Evaluation Associated with High Yield and Early Harvesting Traits
1 Department of Agronomy, Faculty of Agriculture, Universitas Gadjah Mada, Yogyakarta, 55281, Indonesia
2 National Research and Innovation Agency, Jakarta, 10340, Indonesia
* Corresponding Author: Erlina Ambarwati. Email:
(This article belongs to the Special Issue: Recent Research Trends in Genetics, Genomics, and Physiology of Crop Plants)
Phyton-International Journal of Experimental Botany 2024, 93(11), 3073-3089. https://doi.org/10.32604/phyton.2024.058098
Received 04 September 2024; Accepted 29 October 2024; Issue published 30 November 2024
Abstract
The breeding process involves developing techniques to create cultivars that thrive in the ever-changing global climate, allowing for early harvesting and high yield. This study aimed to screen rice genotypes with early harvesting and high yield to develop new-generation cultivars. The study was conducted in a field experiment at the Department of Agronomy, Faculty of Agriculture, Universitas Gadjah Mada, Special Region of Yogyakarta, Indonesia, from December 2022 to April 2023. Ten genotypes were laid out using an Augmented Randomized Complete Block Design (ARCBD) with three rows and six columns. The observations were macro and micro-climate, soil quality, and morphological traits of rice. Data were analyzed using a Linear Mixed Model (LMM), correlation matrix, heatmap cluster, Principal Component Analysis (PCA), PCA-Biplot, Multiple Factor Analysis (MFA), and circular cluster. The results provided information that the rice genotypes with the early harvesting genotypes were Inbrida M70D (95.01 days), Trisakti (100.98 days), and SR Super (104.76 days). Furthermore, the rice genotypes with the highest yields were Gamagora 2 (49.82 g clump−1), Gamagora 4 (46.61 g clump−1), Gamagora 7 (37.26 g clump−1), and Gamagora 10 (29.84 g clump−1). The genetic clustering based on Simple Sequence Repeat markers linked to early flowering genes indicates that SR Super and Inbrida M70D show the most distinct characteristics compared to other genotypes. Molecular findings corroborate the morphological analysis, indicating that Inbrida M70D and SR Super are early harvesting genotypes, therefore they can be used this group of genotypes as cross-breeding material to obtain genotypes with early harvesting and high-yielding traits.Keywords
Asia, Africa, and Latin America consume large amounts of rice [1]. Nearly half the world’s population depends on rice as its primary food supply. Global rice demand is projected to reach approximately 584 million tons by 2050, representing an 11.88% increase from total world rice consumption in 2023/2024 [2]. Rice production must keep pace to meet this growing demand [3]. Asia accounts for 90.6% of global rice production, and Indonesia ranks fourth globally in rice production with a growth rate of 6.9% [4]. In 2023, rice production in Indonesia decreased by 2.05% compared to 2022, which poses significant challenges ahead [5].
The primary reason for the decline in rice production in Indonesia is the decrease in the land available for growing rice. The harvesting area in 2023 decreased by 2.45% compared to 2022 [5]. The annual conversion of paddy fields to non-paddy fields is significantly higher than the expansion of paddy fields. Projections for 2045 indicate a further reduction, leaving only 6 million hectares of rice fields [6]. The situation is further worsened by the severe impact of global climate change [7–9]. Global climate change will cause a decrease in rice production by 6% to 12% by the year 2050 [10]. A further forecast suggests that rice production and cultivation will decrease by almost 51% during the twenty-first century [11].
Recent research developments have sparked increased interest in adaptable agricultural strategies to counter the potential decrease in rice production due to global climate change [12]. Cultivars offer a promising approach to enhancing rice production [13]. Productivity improvements significantly impact rice production growth, contributing 56% of the increase, compared to only 26.3% from expanding the harvested area. The rice breeding program in Indonesia spanned from the 1920s to 2015 [14,15]. Until 2015, the Ministry of Agriculture Republic Indonesia has introduced 550 rice varieties, each with specific advantages such as a short duration period, high yield, tolerance to pests and diseases, and suitability to different ecological conditions [16]. Currently, no cultivar possesses both early harvesting (less than 90 to 104 days) and high yield (greater than 10 tons ha−1). These characteristics must be stable and adaptable in lowland and upland rice agroecosystems [17–20].
The breeding process involves developing techniques to create cultivars that thrive in the ever-changing global climate, allowing for earlier harvesting and high yields on marginal land. In rice ecosystems that rely on rainfall, specific cultivars with a growth duration of 95 to 105 days can tolerate drought, even after the end of the wet season [21]. Furthermore, early harvesting rice cultivars offer distinct advantages over those farmers usually grow. This benefit involves reducing the risks associated with El Nino and La Nina events and the harmful effects caused by pests and diseases. To mitigate the impact of climate change and as a substitute for intensive farming, it is imperative to cultivate superior cultivars that are early harvesting and high yields [22–24]. Plant breeding programs start with pre-breeding activities to select genotypes of parent materials based on targeted traits [25]. Pre-breeding can be conducted using morphological analysis of target traits [26]. Additionally, molecular markers can guide pre-breeding programs in exploring natural genetic variation for complete genotypic identification [27]. The Simple Sequence Repeat (SSR) marker has proven to be a valuable method for assisting breeders in selecting parent lines with markers linked to the target traits [28].
In pre-breeding programs, selecting parent materials with the desired target traits is crucial for successful cultivar development. Universitas Gadjah Mada has Gamagora rice genotypes with high yield potential, but their harvesting time is more than 110 days. We plan to cross the Gamagora with early harvesting rice varieties to develop rice with high yield and early harvesting traits. Several Gamagora genotypes show significant yield potential, which this study aims to confirm. Meanwhile, scientific validation of early harvesting traits in the local varieties used in this study is lacking, as current knowledge is primarily based on tradition without statistical support. This study will utilize statistical analysis and phenotypic-genotypic methods to confirm that the Gamagora genotypes have high yield potential and that the local varieties exhibit early harvesting traits. It is essential to strengthen the foundation for selecting parental lines in future rice breeding programs.
A total of 10 different rice genotypes, including three promising rice lines and one national variety from the Faculty of Agriculture, Universitas Gadjah Mada, Indonesia, and six local cultivars (Table 1), were utilized as research materials.
2.2 Experimental Layout and Agronomic Practices
The study was conducted in a field experiment at the Department of Agronomy, Faculty of Agriculture, Universitas Gadjah Mada, Special Region of Yogyakarta, Indonesia, from December 2022 to April 2023. The geographical location was at the coordinates 7°46′14.5812′′ S and 110°22′39.8064′′ E. Three promising rice lines and one national variety from the Faculty of Agriculture, Universitas Gadjah Mada, Indonesia, were used as check entries, each appearing once in every block. Local cultivars used as test entries were not replicated. The experimental design used an Augmented Randomized Complete Block Design (ARCBD) with three rows and six columns.
The pot size used in this study was 25 cm × 16 cm × 16 cm. Pots were placed at a distance of 0.5 m. Each treatment consisted of five pots as experimental units. The planting medium was soil with a weight per pot of ±12.50 kg. Rice seeds were soaked for 12 h before seeding. Transplanting rice seedlings into pots was done at 21 days. The fertilization used Urea, Zwavelzure Ammoniac (ZA), SP-36, and KCl, respectively. The doses of urea, ZA, SP-36, and KCl were 300, 100, 150, and 150 kg ha−1, respectively, or the equivalent of 1.88, 0.63, 0.94, and 0.94 g pot−1. Urea was applied thrice at 14, 28, and 42 days after transplanting (DAT). ZA, SP-36, and KCl were applied once at 14 DAT. Irrigation was carried out every day up to field capacity. Control of brown planthopper (Nilaparvata lugens), blast (Pyricularia oryzae), and bacterial leaf blight (Xanthomonas oryzae pv. Oryzae) used insecticides, fungicides, and bactericides with active ingredients of pimetrozin, mancozeb, and copper hydroxide, respectively.
Macro and micro-climate observations during the study included total rainfall (mm), average air temperature (°C), and average relative humidity (%). Macro and micro-climate were observed during the study.
Soil quality observations include the soil’s physical, chemical, and biological properties. The physical properties observed include soil texture (% clay, % sand, % silt), bulk density, soil moisture (pF 0, pF 2.54, pF 4.2), permeability, and porosity [29]. The soil chemical properties observed include pH H2O, organic carbon, cation exchange capacity, electrical conductivity, total nitrogen, soil nutrient availability (phosphorus, potassium, sodium, magnesium, manganese), and exchangeable soil nutrient (iron, aluminum). Soil biological characteristics include total bacteria and total fungi [30,31]. The observations were conducted at the study site and the Laboratory of General Soil and Microbiology, Faculty of Agriculture, Universitas Gadjah Mada, Yogyakarta, Indonesia.
2.3.3 Rice Morphological Traits
The observations of morphological traits were carried out based on Standard Evaluation Systems IRRI [32] including a number of productive tillers (panicle clump−1), plant height (cm), flowering time (days), harvesting time (days), number of grains per panicle (grains), percentage of empty grains per panicle (%), 1000-grains weight (g), yield with a moisture content of 12% (g clump−1). The number of productive tillers and plant height were observed during the maximum vegetative phase. The observations of flowering times (50%) and harvesting time (80%) were carried out in the generative phase. In contrast, the number of grains per panicle, percentage of empty grains per panicle, 1000-grains weight, and yield were carried out at harvest.
2.3.4 DNA Isolation and Amplification
Genomic DNA was extracted from 0.1 g leaf tissue using Geneaid GP100 Genomic DNA Mini Kit (Plant). Its concentration and purity were measured using Nanodrop. Genomic DNA was diluted to 25 ng μL−1 in ddH2O to be used as a DNA template. Reagen composition for PCR consists of 5 μL GoTaq®® Green Master Mix, 0.5 μL forward primer, 0.5 μL reverse primer [33–35], 1.5 μL Nuclease Free Water, and 2.5 μL DNA template. The PCR program was carried out using a pre-denaturation temperature at 94°C for 5 min, followed by 35 cycles of denaturation at 94°C for 1 min, annealing for 2 min, and extension at 72°C for 2 min, and the final extension at 72°C for 10 min. The PCR products were separated by electrophoresis on a 1.5% agarose gel and visualized with a UV transilluminator [36]. SSR markers linked to early flowering genes used in this study are shown in Table 2.
The data on morphological traits were analyzed using a Linear Mixed Model (LMM) [37]. The LMM results were then continued with Tukey-Kramer grouping for genotype Least Squares Means (LS-Means) (p < 0.05) and visualized with a box plot with error bars. The relationship between two morphological traits was analyzed using a correlation matrix, and the grouping of rice genotypes based on their related morphological traits was visualized using a heatmap cluster [38,39].
A PCA-Biplot analysis is performed on the rice genotype and morphological traits data matrix to create a two-dimensional graph representing the relationship between rice genotypes and morphological traits [40]. It is achieved by systematically assigning values to the elements of the data matrix.
Screening rice genotypes associated with early harvesting and high yields was performed using Multiple Factor Analysis (MFA) and grouped with circular clusters. The rotation used in MFA was varimax [41,42]. Screening for morphological traits and rice genotypes will be used to determine criteria for early harvest and high yields and significantly contributed that had a communality value of >0.5 [43].
The Linear Mixed Model (LMM), Least Squares Means (LS-Means), and Tukey-Kramer grouping were analyzed with SAS®® Studio software using proc glimmix [44]. The visualized of macro and micro-climate, randomization of pot trial in the field, correlation matrix, heatmap cluster, PCA, PCA-Biplot, MFA, and circular cluster were visualized with Rstudio software using Plotly, shiny, FielDHub, corrplot, FactoMineR, factoextra, GGally, ggplot2, magrittr, pheatmap, and scatterplot3d packages [45–47]. The visualized DNA bands were analyzed by scoring the presence or absence of bands. Score 1 indicated presence, while 0 indicated absence. The binary data will be processed for genetic clustering analysis with Rstudio based on Jaccard’s coefficient and UPGMA clustering method [48].
The study site’s altitude was 140 m above sea level. The total rainfall during the study was 1240.40 mm. The highest monthly rainfall was in February, at 375.30 mm, while the lowest was in April, at 60.40 mm. The average relative air temperature during the study ranged between 24°C–28°C, while the average relative humidity was 81%–97% (Fig. 1).
Figure 1: Macro and micro-climate in the study site. RR: Total rainfall; Tair: Average air temperature; RH: Average relative humidity
The soil quality analysis indicates that the soil texture is sandy loam, with a permeability of 18.00 cm ha−1 and a porosity of 45.37%, classified as fast. The bulk density of the soil was 1.12 g cm−3, and water availability varied between pF 2.54 and pF 4.2 by 23.85%. The value of pH H2O, soil organic carbon, cation exchange capacity, and electrical conductivity were 6.3, 1.92%, 19.19 cmol (+) kg−1, and 1.548 dS m−1, respectively. These traits were categorized as slightly acid, low, moderate, and low (Table 3).
The total nitrogen concentration was categorized as low, whereas the availability of phosphorus and potassium was medium. The total nitrogen, phosphorus, and potassium concentration were 0.18%, 15.14 mgL−1, and 0.44 cmol(+) kg−1, respectively. Manganese availability was measured at 1.2 mgL−1, while sodium, calcium, and magnesium values were 0.54, 9.16, and 1.51 cmol(+) kg−1, respectively. Manganese had a low availability, while sodium, calcium, and magnesium had a medium availability in the soil. Exchangeable iron and aluminum were classified as a low category. The values for each of them are 3.3 and 3.2 ppm, respectively. In the soil biology, the total bacteria count was 1.43 × 105 cfu g−1 dry soil, while the total fungi count was 1.28 × 103 cfu g−1 dry soil. Based on climate and soil quality evaluations, the study site was marginally suitable for rice cultivation (Table 3).
3.2 Morphological Traits of Rice Genotypes
A comprehensive analysis of the morphological traits of rice genotypes highlighted significant disparities among the different genotypes. Based on the number of productive tillers, Gamagora 7 was significantly higher than the Gamagora 4 genotype. Specifically, the Gamagora 7 had 22.00 panicle clump−1, while the Gamagora 4 had 21.30 panicle clump−1. In contrast, the genotypes SR Super, Genjah Blora, and Inbrida M70D showed the lowest panicle clump−1, with 13.40, 12.73, and 10.50 panicle clump−1, respectively (Fig. 2a). The assessment of productive tillers provided insight into the growth characteristics, which were further complemented by observations of plant height. Genjah Blora, Cakrabuana 04, and Gamagora 10 had the highest plant height, measuring 108.07, 106.63, and 103.91 cm, respectively. At the same time, the shortest genotype was SR Super, with a notable height of only 78.25 cm (Fig. 2b). In contrast to the number of productive tillers and plant height, Inbrida M70D shows notable performance in flowering and harvesting time, with the shortest duration among all genotypes, with significant differences of 66.33 and 95.01 days compared to others. In contrast, Gamagora 2 exhibited the most prolonged flowering and harvesting durations, at 103.33 and 133.11 days, respectively (Fig. 2c,d).
Following a detailed exploration of growth components, the analysis of yield components associated with the rice genotypes revealed the potential yield of each genotype. Gamagora 2 exhibited the highest number of grains per panicle, 180.52 grains. In contrast, the MSP Beras Merah and Trisakti had the lowest values, around 59.43 and 49.66 grains, respectively (Fig. 3a). Gamagora 7 had the lowest percentage of empty grain per panicle, with a value of 18.49%. At the same time, the other genotypes showed no significant variations (Fig. 3b). Gamagora 4 had the highest 1000-grain weight of 33.44 g, whereas the Inbrida M70D and SR Super displayed the lowest of 17.94 and 17.71 g, respectively (Fig. 3c). The genotype that achieved the highest yield was Gamagora 2, which yielded 49.82 g clump−1. Gamagora 4 and Gamagora 7 achieved second and third place, respectively, with 46.61 and 37.26 g clump−1. SR Super and Inbrida M70D exhibited the lowest yields, producing 14.53 and 12.08 g clump−1, respectively (Fig. 3d).
Figure 2: Boxplot of growth component of rice genotypes. (a) Number of productive tillers, (b) plant height, (c) flowering time, (d) harvesting time
Figure 3: Boxplot of yield component and yield of rice genotypes. (a) Number of grains per panicle, (b) Percentage of empty grain per panicle, (c) 1000-grains weight, (d) yield
3.3 Relationship between Morphological Traits
The results of the correlation matrix provide information that there was a positive correlation with yield, namely flowering time (r = 0.416*), harvesting time (r = 0.375*), and number of grains per panicle (r = 0.663***). Conversely, a negative correlation was observed between the percentage of empty grains per panicle (r = −0.348*) (Fig. 4a). Higher yields correlate with more prolonged flowering and harvesting times, while a higher percentage of empty grains can reduce yield. The genotypes tested were divided into two big clusters that generally have differences in flowering time, harvesting time, and yield component (Fig. 4b). The first cluster consists of MSP 17 Beras Merah, Genjah Blora, Cakrabuana, SR Super, Inbrida M70D, and Trisakti. The second cluster comprises Gamagora 2, Gamagora 4, Gamagora 7, and Gamagora 10. The heatmap colors indicate the data values. The first cluster tends to lower harvesting time, flowering time, and yield component values than the second cluster. The PCA is employed to streamline the variables that were previously chosen. The PCA simplifies data analysis by lowering the number of variables while retaining the relevant information. The PCA results provide information about the contribution of PC1 and PC2, which were 51.00% and 16.90%, respectively. The genotype contributions to PC1 and PC2 from highest to lowest are Inbrida M70D, Gamagora 2, Gamagora 4, Gamagora 7, Genjah Blora, Cakrabuana 04, MSP 17 Beras Merah, SR Super, Gamagora 10, and Trisakti, respectively (Fig. 4c).
Figure 4: Relationship between morphological traits and rice genotypes. (a) Correlation matrix. (b) Heatmap cluster. (c) Individuals—PCA, and (d) PCA—Biplot. 1: Gamagora 2; 2: Gamagora 4; 3: Gamagora 7; 4: Gamagora 10; 5: Cakrabuana 04; 6: Genjah Blora; 7: Inbrida M70D; 8: MSP 17 Beras Merah; 9: SR Super; 10: Trisakti; PEGP: Percentage of Empty Grains per Panicle; PH: Plant Height; 1000-Grains Weight; NPT: Number of Productive Tillers; Y: Yield; HT: Harvesting Time; FT: Flowering Time; NGP: Number of Grains per Panicle
The PCA-Biplot (Fig. 4d) indicated that the first group, quadrant I, consisted of three genotypes. The genotypes mentioned were Cakrabuana 04, SR Super, and Trisakti distinguished by specific morphological traits, namely plant height and the percentage of empty grains per panicle. The following traits were ranked in descending order of their contribution. Gamagora 2 constituted the second group, commonly referred to as quadrant II. The most significant morphological traits for Gamagora 2, ranked in descending order of importance, were the flowering time, rice yield, and harvesting time. The third group, quadrant III, consisted of Genjah Blora, Inbrida M70D, and MSP 17 Beras Merah. Quadrant III, the third group, did not exhibit any specific morphological traits linked to the genotypes. In quadrant IV, the fourth group consisted of Gamagora 4, Gamagora 7, and Gamagora 10. These genotypes exhibited distinct morphological traits, such as the number of productive tillers, 1000-grain weight, and the number of grains per panicle. The traits were ranked according to their contribution, from highest to lowest. In descending order, the morphological traits that contributed most to the formation of these clusters were the number of productive tillers, flowering time, rice yield, harvesting time, 1000-grains weight, plant height, number of grains per panicle, and percentage of empty grains per panicle.
3.4 Screening of Rice Genotypes Associated with Early Harvesting and High-Yielding
The screening obtained through multiple factor analysis (MFA) revealed that the critical morphological traits that significantly contributed (a communality value greater than 0.5) were the number of productive tillers, flowering time, rice yield, harvesting time, and 1000-grains weight. The plant height, number of grains per panicle, and the percentage of empty grains per panicle were negligible (Fig. 5a). The prominent morphological traits were employed to establish a circular cluster for identifying the rice genotype with the early harvesting and highest yields. Based on the genotype contributions and circular cluster analysis, it was determined that the rice genotypes with the early harvesting genotypes were Inbrida M70D (95.01 days), Trisakti (100.98 days), and SR Super (104.76 days) (Fig. 5b,d). Furthermore, the highest yields were Gamagora 2 (49.82 g clump−1), Gamagora 4 (46.61 g clump−1), Gamagora 7 (37.26 g clump−1), and Gamagora 10 (29.84 g clump−1) (Fig. 5c,d). Using this group of genetic types as a resource for cross-breeding can produce genetic types with the desirable traits of early harvesting and high yield.
Figure 5: Screening of rice genotypes associated with early harvesting and high yield. (a) Contributions of morphological traits for rice genotypes, (b) Screening of rice genotypes for early harvesting, (c) Screening of rice genotypes for high yield, (d) Circular cluster for screening of rice genotypes associated with early harvesting and high yields. 1: Gamagora 2; 2: Gamagora 4; 3: Gamagora 7; 4: Gamagora 10; 5: Cakrabuana 04; 6: Genjah Blora; 7: Inbrida M70D; 8: MSP 17 Beras Merah; 9: SR Super; 10: Trisakti
3.5 Genetic Clustering of Ten Genotypes Based on SSR Linked to Early Flowering Genes
Six SSR markers linked to early-flowering genes were used to construct the genetic clustering to get information about similarities from clustering based on the similarity coefficient. Two clusters formed at a similarity coefficient of 0.52 (Fig. 6a). The first clusters were MSP 17 Beras Merah, Gamagora 10, Genjah Blora, Gamagora 7, Gamagora 2, Cakrabuana 04, Trisakti, Inbrida M70D, and SR Super. The second group contains only Gamagora 4. The two big clusters show that Gamagora 4 had the furthest genetic distance from the other nine genotypes. At 0.52, the first group was divided into two groups, where Inbrida M70D and SR Super were in one group and separated from the others. Genjah Blora and Gamagora 7 were in the same group at a coefficient of 0.75, which means that the two genotypes had the most similar traits compared to the others.
Figure 6: Genetic diversity of ten genotypes based on six SSR markers linked to early-flowering genes. (a) Genetic clustering based on Jaccard’s similarity coefficient and UPGMA method, (b) The coefficient of genetic dissimilarity based on Jaccard’s coefficient
Based on the coefficient dissimilarity, the highest coefficient (0.71) was between Gamagora 4 and Inbrida M70D, indicating that these two genotypes were the least similar compared to the others (Fig. 6b). The other pairs of Gamagora and early-harvesting genotypes with coefficient values greater than 0.5 were Gamagora 2 with SR Super (0.53), Gamagora 2 with Inbrida M70D (0.58), Gamagora 4 with SR Super (0.61), Gamagora 4 with Cakrabuana 04 (0.58), Gamagora 4 with Trisakti (0.55), Gamagora 10 with Trisakti (0.53), Gamagora 10 with Inbrida M70D (0.52), Gamagora 4 with Genjah Blora (0.55).
Early-harvesting rice cultivars also have advantages over long-duration cultivars. These advantages include reduced risks during El Nino and La Nina, and damage from pests and diseases [49]. Developing superior cultivars with early harvesting and high yield will provide an alternative solution to climate change problems and the need for intensive cultivation. The initial step of the breeding program is pre-breeding, which involves all activities associated with identifying desirable traits or genes [26]. This study analyzed morphological traits associated with yield components and harvesting time.
The genotypes that showed high yields were Gamagora 2, Gamagora 4, and Gamagora 7, with 49.82, 46.61, and 37.26 g clump−1, but they have long harvesting periods of 133.11, 124.89, and 122 days, respectively. Gamagora 2 has the highest yield despite having lesser weights of 1000 grains and productive tillers than Gamagora 4, with a similar percentage of empty grains. That is because Gamagora 2 has the highest number of grains per panicle, which shows a significant positive correlation with yield components (r = 0.663***) (Fig. 4a). Rice grain yield is a complex quantitative trait determined by three major yield components, namely panicle number, grain number per panicle, and grain weight [50–52], with grain number as the critical trait for increasing grain yield in a breeding program [52–55]. Moreover, the genes associated with grain number have pleiotropic effects, influencing panicle number, grain weight, flowering time, and traits related to domestication [52].
The early flowering and harvesting genotypes in this study had low yields. The critical finding was that the Inbrida M70D genotype showed the earliest harvesting time, namely 95.01 days, but had a low yield of 12.08 g clump−1. Short-duration genotypes generally have lower yield potential than medium-to-long duration types because longer growth durations increase their ability to capture resources and crop biomass [56]. One of the early-flowering genes, RFT1, also has pleiotropic effects on several agronomic traits, enhancing plant height and yield potential by extending the vegetative stage [57]. Short-duration varieties have a short vegetative growth period, and the reproductive period is similar for all rice genotypes [56]. Rice harvesting time is critical and relates to the value of yield and yield components and the adaptability of various lowland and upland rice cultivars [58,59].
Morphological traits indicate a positive correlation with rice yield, including flowering time, harvesting time, and number of grains per panicle (Fig. 4a). Extended flowering and harvesting periods typically result in higher yields. The genotypes tested were divided into two big clusters that generally have differences in flowering time, harvesting time, and yield component (Fig. 4b). That is visualized in a heatmap divided into two clusters, each cluster generally exhibits a shorter period with lower yield and a more extended period with higher yield. The clustering of genotypes in the heatmap is similar to the grouping in PCA. Principal component analysis in this study provides information on the relationship between several morphological traits contributing significantly to rice genotypes. Rice genotypes with high yield potential, such as Gamagora 2, Gamagora 4, Gamagora 7, and Gamagora 10, were in different quadrants, along with genotypes with early flowering and harvesting potential, such as Cakrabuana 04, Genjah Blora, Inbrida M70D, MSP 17 Beras Merah, SR Super, and Trisakti (Fig. 4d). Reducing the growth duration of rice genotypes will change the yield and yield components during sink formation so that early flowering and harvesting genotypes have low yields. Whether rice yield limitations are caused by source capacity, sink capacity, or a combination of both for short-lived cultivars is still being determined. Rice cultivars with higher yields have a longer period to absorb nutrients, water, and sunlight, thereby increasing leaf area, yield components, and rice yield [60].
Multiple factor analysis (MFA) and circular cluster were used to screen rice genotypes related to early harvest and the highest yield. The initial screening aimed to identify morphological traits with significant contributions characterized by a communality value >0.5. Several studies use MFA to screen soil quality traits that significantly affect the yield of several commodities, such as rice [61] and soybean [20,62]. Three variables related to this study, flowering time, harvesting time, and rice yield, were used to form circular clusters. Based on genotype contributions and circular cluster analysis, the rice genotypes with the highest yields were Gamagora 2 (49.82 g clump−1), Gamagora 4 (46.61 g clump−1), Gamagora 7 (37.26 g clump−1), and Gamagora 10 (29.84 g clump−1). Furthermore, the rice genotypes with early harvesting were Inbrida M70D (95.01 days), Trisakti (100.98 days), and SR Super (104.76 days). The Multiple Factor Analysis (MFA) findings regarding early flowering and harvesting traits are further corroborated by genetic clustering analysis based on Simple Sequence Repeat (SSR) markers, providing additional evidence for the claims concerning early maturity traits.
Genetic clustering derived from similarity coefficients illustrates the similarity among tested genotypes and facilitates their selection as parental lines for breeding programs. SSR markers are also used to select drought-tolerant rice genotypes that will be used for parent material in breeding programs [42]. The result of this study shows that SR Super and Inbrida M70D separated from other genotypes at a coefficient of 0.52. This study uses SSR markers linked to early flowering genes, so the clusters tend to have similarities in flowering traits. All the SSR markers in this study are linked with early-flowering genes. There were three markers on chromosome 6, namely RM 8225 linked with the Hd1 gene, RM 190, and RM 225 linked with Hd3a and RFT1 genes [34]. RM 8006 and RM 3832 are markers on chromosome 7 that are linked to the Ghd7 gene [35]. RM 5620 is the marker in chromosome 10 linked with the Ehd1 gene [36]. The smaller the similarity coefficient value, the greater the genetic distance. The greater the genetic distance of the parental line, the greater the effect of heterosis [63]. Based on the result of genetic clustering showed that Gamagora 4 is the most recommended genotype for crossing with other early-flowering parents. Inbrida M70D and SR Super are the most recommended early flowering genotypes to cross with Gamagora because both genotypes have the smallest similarity coefficient from Gamagora.
These findings indicate that all Gamagora rice genotypes exhibit promising high-yield traits, while Inbrida M70D and SR Super are strong candidates for early harvesting. Crossing these genotypes is expected to produce rice varieties that combine high yield and early harvesting. With shorter life cycles, these genotypes may also reduce water requirements, so it can be a solution in dealing with increasing temperatures due to climate change.
While this study provides valuable insights into high-yield and early-maturing rice genotypes, it is essential to note that the number of genotypes evaluated may not fully represent the genetic diversity needed for the broad applicability of the findings. The limited genetic pool could restrict the identification of potentially more robust genotypes with desirable traits. Therefore, future studies should aim to expand genetic diversity by including a broader range of genotypes, which would enhance the likelihood of discovering more stable and high-performing varieties.
The results provided information that the rice genotypes with the early harvesting genotypes were Inbrida M70D (95.01 days), Trisakti (100.98 days), and SR Super (104.76 days). These genotypes had the potential to develop varieties that were agronomically beneficial due to early harvesting traits. Furthermore, the highest yields were Gamagora 2 (49.82 g clump−1), Gamagora 4 (46.61 g clump−1), Gamagora 7 (37.26 g clump−1), and Gamagora 10 (29.84 g clump−1). The genetic clustering analysis based on SSR markers linked to early flowering genes indicates that SR Super and M70D have the most distinct characteristics compared to other genotypes. Molecular findings corroborate the morphological analysis, which indicates that Inbrida M70D and SR Super are early-harvesting genotypes.
Acknowledgement: The authors sincerely thank all members of the Gamagora development team for their assistance in carrying out this research activity.
Funding Statement: The research for this article was fully funded by the National Research and Innovation Agency and the Indonesia Endowment Funds for Education (LPDP), Ministry of Finance through the Program Riset dan Inovasi untuk Indonesia Maju Gelombang 3 grant-in-aid scheme (No. 12/II.7/HK/2023).
Author Contributions: Study conception and design: Taryono, Erlina Ambarwati, Supriyanta, Taufan Alam, Alwa Widi Aisya; data collection: Taufan Alam, Supriyanta, Alwa Widi Aisya; analysis and interpretation of results: Taufan Alam, Alwa Widi Aisya, Erlina Ambarwati, Taryono, Supriyanta; draft manuscript preparation: Alwa Widi Aisya, Taufan Alam, Erlina Ambarwati, Taryono, Rizky Pasthika Kirana, I Gusti Komang Dana Arsana, Vina Eka Aristya, Ardian Elonard Purba. All authors reviewed the results and approved the final version of the manuscript.
Availability of Data and Materials: The data used in this study can be accessed by contacting the corresponding author upon request.
Ethics Approval: Not applicable.
Conflicts of Interest: The authors declare that they have no conflicts of interest to report regarding the present study.
References
1. Zhao M, Lin Y, Chen H. Improving the nutritional quality of rice for human health. Theor Appl Genet. 2020;133(5):1397–413. doi:10.1007/s00122-019-03530-x [Google Scholar] [PubMed] [CrossRef]
2. FAO. Food outlook—Biannual report on global food markets. Rome: Food and Agriculture Organization of the United Nations; 2023. p. 160. [Google Scholar]
3. Samal P, Babu S, Mishra B. The global rice agriculture towards 2050: an inter-continental perspective. Outlook Agric. 2022;51(2):164–72. doi:10.1177/00307270221088338. [Google Scholar] [CrossRef]
4. USDA. Rice sector at a glance. 2023. Available from: https://www.ers.usda.gov/topics/crops/rice/rice-sector-at-a-glance/. [Accessed 2024]. [Google Scholar]
5. Statistic Indonesia. Berita resmi statistik. 2023. Available from: https://www.bps.go.id/id/pressrelease/2024/03/01/2375/pada-2023--luas-panen-padi-mencapai-sekitar-10-21-juta-hektare-dengan-produksi-padi-sebesar-53-98-juta-ton-gabah-kering-giling--gkg-.html. [Accessed 2024]. [Google Scholar]
6. Mulyani A, Nursyamsi D, Syakir M. Strategi pemanfaatan sumberdaya lahan untuk pencapaian swasembada beras berkelanjutan. J Sumberd Lahan. 2017;11(1):11–22. [Google Scholar]
7. FAO. FAO strategy on climate change 2022–2031. Rome: Food and Agriculture Organization of the United Nations; 2022. p. 36. [Google Scholar]
8. Ansari A, Pranesti A, Telaumbanua M, Alam T, Taryono, Wulandari R, et al. Evaluating the effect of climate change on rice production in Indonesia using a multimodelling approach. Heliyon. 2023;9(9):1–15. [Google Scholar]
9. Ansari A, Wuryandani S, Pranesti A, Telaumbanua M, Ngadisih, Hardiansyah M, et al. Corrigendum: optimizing water-energy-food nexus: achieving economic prosperity and environmental sustainability in agriculture. Front Sustain Food Syst. 2023;7:1–7. [Google Scholar]
10. Sun J, Chen L, Ogle S, Cheng K, Xu X, Li Y, et al. Future climate change may pose pressures on greenhouse gas emission reduction in China’s rice production. Geoderma. 2023;440(25):1–10. [Google Scholar]
11. Hussain S, Huang J, Ahmad S, Nanda S, Anwar S, Shakoor A, et al. Rice production under climate change: adaptations and mitigating strategies. In: Fahad S, Hasanuzzaman M, Alam M, Ullah H, Saeed M, Khan I, et al., editors. Environment, climate, plant and vegetation growth. Berlin, Germany: Springer; 2020. p. 659–86. [Google Scholar]
12. Jamal M, Kristiansen P, Kabir M, de Bruyn L. Challenges and adaptations for resilient rice production under changing environments in Bangladesh. Land. 2023;12(6):1217–38. doi:10.3390/land12061217. [Google Scholar] [CrossRef]
13. Piepho H, Nazir M, Qamar M, Rattu A, Din R, Hussain M, et al. Stability analysis for a countrywide series of wheat trials in Pakistan. Crop Sci. 2016;56(5):2465–75. doi:10.2135/cropsci2015.12.0743. [Google Scholar] [CrossRef]
14. Las I. Development of varieties in national rice. In: Proceedings of Food Crop Agriculture and Innovation Seminar, 2004 Aug 5; Bogor, Indonesia. [Google Scholar]
15. Sutardi, Apriyana Y, Rejekiningrum P, Alifia A, Ramadhani F, Darwis V, et al. The transformation of rice crop technology in Indonesia: innovation and sustainable food security. Agronomy. 2023;13(1):1–14. [Google Scholar]
16. Jamil A, Satoto, Sasmita P, Baliadi Y, Guswara A, Suharna. Description of new superior varieties of rice. Jakarta, Indonesia: Indonesian Agency for Agricultural Research and Development; 2015. p. 83. [Google Scholar]
17. Eberhat S, Russell W. Stability parameters for comparing varieties. Crop Sci. 1966;6(1):36–40. doi:10.2135/cropsci1966.0011183X000600010011x. [Google Scholar] [CrossRef]
18. Yan W, Kang M, Ma B, Woods S, Cornelius P. GGE-biplot vs. AMMI analysis of genotype-by-environment data. Crop Sci. 2007;47(2):643–55. doi:10.2135/cropsci2006.06.0374. [Google Scholar] [CrossRef]
19. Alam T, Suryanto P, Supriyanta, Basunanda P, Wulandari R, Kastono D, et al. Rice cultivar selection in an agroforestry system through GGE-biplot and EBLUP. Biodiversitas. 2021;22(11):4750–7. [Google Scholar]
20. Taryono, Suryanto P, Supriyanta, Wulandari R, Putra E, Widyawan M, et al. Morpho-physiological and biochemical fingerprints of the soybean agroforestry system in different crop rotation models. Indian J Agric Res. 2023;57(4):481–6. [Google Scholar]
21. Onho H, Banayo N, Bueno C, Kashiwagi J, Nakashima T, Iwama K, et al. On-farm assessment of a new early-maturing drought-tolerant rice cultivar for dry direct seeding in rainfed lowlands. Field Crop Res. 2018;219:222–8. doi:10.1016/j.fcr.2018.02.005. [Google Scholar] [CrossRef]
22. IRRI. Growing rice, cultivating partnerships: 40 years of Indonesia-IRRI collaboration. Los Baños, Philippines: International Rice Research Institute; 2015. p. 11–2. [Google Scholar]
23. Killenga S, Chuwa C, Myukiye N, Zakayo J, Pau L, Kimaro D. Rice production manual. Ibadan, Nigeria: International Institute of Tropical Agriculture; 2020. p. 24. [Google Scholar]
24. Bassi F, Sanchez-Garcia M, Ortiz R. What plant breeding may (and may not) look like in 2050? Plant Genome. 2023;17(1):1–14. [Google Scholar]
25. Sukumaran S, Rebetzke G, Mackay I, Bentley A, Reynolds M. Pre-breeding strategies. In: Reynolds M, Braun H, editors. Wheat improvement. Cham: Springer; 2022. p. 451–9. [Google Scholar]
26. Singh G, Gudi S, Amandeep, Upadhyay P, Sekhawat P, Nayak G. Unlocking the hidden variation from wild repository for accelerating genetic gain in legumes. Front Plant Sci. 2022;13:1–24. doi:10.3389/fpls.2022.1035878 [Google Scholar] [PubMed] [CrossRef]
27. Cortés A, Du H. Molecular genetics enhances plant breeding. Mol Genet Enhanc Plant Breed. 2023;24(12):9977–86. [Google Scholar]
28. Rezk A, Mohamed A, El-Betagi S. Genetic variability and diversity analysis in Oryza sativa L. genotypes using quantitative traits and SSR markers. Saudi J Biol Sci. 2024;31(3):1–10. doi:10.1016/j.sjbs.2024.103944 [Google Scholar] [PubMed] [CrossRef]
29. Bandyopahyay K, Aggarwal P, Chakraborty D, Pradhan S, Garg R, Singh R. Practical manual on measurement of soil physical properties; division of agricultural physics. New Delhi: Indian Agricultural Research Institute; 2012. p. 7–10. [Google Scholar]
30. Peters J. Wisconsin procedures for soil testing, plant analysis and feed & forage analysis. Madison, Wisconsin: University of Wisconsin; 2013. p. 1–32. [Google Scholar]
31. David A, Davidson C. Estimation method for serial dilution experiments. J Microbiol Met. 2014;107:214–21. doi:10.1016/j.mimet.2014.08.023 [Google Scholar] [PubMed] [CrossRef]
32. IRRI. Standard evaluation system for rice. Los Baños, Philippines: International Rice Research Institute; 2013. p. 56. [Google Scholar]
33. Subudhi P, Leon T, Tapia R, Chai C, Karan R, Ontoy J, et al. Genetic interaction involving photoperiod-responsive hd1 promotes early flowering under long-day conditions in rice. Sci Rep. 2018;8(1):1–11. doi:10.1038/s41598-018-20324-1 [Google Scholar] [PubMed] [CrossRef]
34. Nonoue Y, Hori K, Ono N, Shibaya T, Tanaka E, Mizobuchi R, et al. Detection of heading date QTLs in advanced-backcross populations of an elite indica rice cultivar, IR64. Breed Sci. 2019;69(2):352–8. doi:10.1270/jsbbs.18172 [Google Scholar] [PubMed] [CrossRef]
35. Hori K, Nonoue Y, Ono N, Shibaya T, Ebana K, Matsubara K, et al. Genetic architecture of variation in heading date among Asian rice accessions. BMC Plant Biol. 2015;15(1):115–32. doi:10.1186/s12870-015-0501-x [Google Scholar] [PubMed] [CrossRef]
36. Kouakou J-L, Gonedelé-Bi S, Assamoi J-B, N’Guetta S-P. Optimization of the Cetyltrimethylammonium bromide (CTAB) DNA extraction protocol using forest elephant dung samples. MethodsX. 2022;9(1):1–12. [Google Scholar]
37. Welham S, Grezan S, Clark S, Mead A. Statistical methods in biology: design and analysis of experiments and regression. Boca Raton, FL, USA: CRC Press; 2015. p. 427. [Google Scholar]
38. Wilkinson L, Friendly M. The history of the cluster heatmap. Am Stat. 2009;63(2):179–84. doi:10.1198/tas.2009.0033. [Google Scholar] [CrossRef]
39. Widyawan M, Hasanah A, Taryono, Alam T, Sayekti R, Pramana A, et al. Multivariate analysis unravels genetic diversity and the relationship between agronomic traits, protein, and dietary fiber in yardlong bean (Vigna unguiculata subsp. sesquipedalis Verdc.). Biodiversitas. 2020;21(12):5662–71. [Google Scholar]
40. Gabriel K. The biplot graphic display of matrices with application to principal component analysis. Biometrika. 1971;58(3):453–67. doi:10.1093/biomet/58.3.453. [Google Scholar] [CrossRef]
41. Kaiser H. The varimax criterion for analytic rotation in factor analysis. Psychometricka. 1958;23:187–200. doi:10.1007/BF02289233. [Google Scholar] [CrossRef]
42. Taryono, Supriyanta, Wulandari R, Nurmansyah, Ambarwati E, Arsana I, et al. Selection of drought-tolerant rice genotypes under cajuput (Melaleuca cajuputi subsp. cajuputi) agroforestry system. Biodiversitas. 2023;24(9):4791–802. [Google Scholar]
43. Greenacre M, Blasius J. Multiple correspondence analysis and related methods. New York: Chapman and Hall/CRC; 2006. [Google Scholar]
44. SAS Institute Inc. SAS®® on demand for academics. North Carolina, USA: SAS Institute Inc.; 2021. [Google Scholar]
45. Kassambara A. Machine learning essentials; Practical guide in R. Statistical Tools for High-throughput Data Analysis (STHDA); 2017. Available from: http://www.sthda.com/english/. [Accessed 2024]. [Google Scholar]
46. R Core Team. R: a language and environment for statistical computing; 2017. Available from: https://www.R-project.org. [Accessed 2024]. [Google Scholar]
47. Sievert C. Interactive web-based data visualization with P, plotly, and shiny. 1st edUK: Routledge and CRC Press; 2020. p. 96–100. [Google Scholar]
48. Serrote C, Reinger L, Silva K, Rabaiolli S, Stefanel C. Determining the polymorphism information content of a molecular marker. Gene. 2020;726(1):144–75. [Google Scholar]
49. Chen J, Cao F, Yin X, Huang M, Zou Y. Yield performance of early-season rice cultivars grown in the late season of double-season crop production under machine-transplanted conditions. PLoS One. 2019;14(3):1–11. [Google Scholar]
50. Xing Y, Zhang Q. Genetic and molecular bases of rice yield. Annu Rev Plant Biol. 2010;61(1):421–42. doi:10.1146/arplant.2010.61.issue-1. [Google Scholar] [CrossRef]
51. Zuo J, Li J. Molecular genetic dissection of quantitative trait loci regulating rice grain size. Annu Rev Genet. 2014;48(1):99–118. doi:10.1146/genet.2014.48.issue-1. [Google Scholar] [CrossRef]
52. Lu Y, Zhuan M, Wang M, Chen R, Tao T, Zhou Y, et al. Genetic molecular factors in determining grain number per panicle of rice. Front Plant Sci. 2022;13:1–14. [Google Scholar]
53. Huo X, Wu S, Zhu Z, Liu F, Fu Y, Cai H, et al. NOG1 increases grain production in rice. Nat Commun. 2017;8(1):1–14. [Google Scholar]
54. Gouda G, Gupta M, Donde R, Mohapatra T, Vadde R, Bahera L. Marker-assisted selection for grain number and yield-related traits of rice (Oryza sativa L.). Physiol Mol Biol Plants. 2020;26(5):885–98. doi:10.1007/s12298-020-00773-7 [Google Scholar] [PubMed] [CrossRef]
55. Wu X, Liang Y, Gao H, Wang J, Zhao Y, Hua L. Enhancing rice grain production by manipulating the naturally evolved cis-regulatory element-containing inverted repeat sequence of OsREM20. Mol Plant. 2021;14(6):997–1011. doi:10.1016/j.molp.2021.03.016 [Google Scholar] [PubMed] [CrossRef]
56. Won P, Liu H, Banayo N. Identification and characterization of high-yielding, short-duration rice genotypes for tropical Asia. Crop Sci. 2020;60(5):2241–50. doi:10.1002/csc2.v60.5. [Google Scholar] [CrossRef]
57. Zhu Y, Fan Y, Wang K, Huang D, Liu W, Ying J, et al. Rice flowering locus T1 plays an important role in heading date influencing yield traits in rice. Sci Rep. 2017;7(1):1–10. [Google Scholar]
58. Mrudhula K, Veni B. Effect of date of harvesting on yield and quality of different rice varieties. Agric Updat. 2020;15(3):188–92. doi:10.15740/HAS/AU. [Google Scholar] [CrossRef]
59. Sujariya S, Jongdee B, Fukai S. Estimation of flowering time and its effect on grain yield of photoperiod sensitive varieties in rainfed lowland rice in Northeast Thailand. F Crop Res. 2022;302:211–25. [Google Scholar]
60. Cheng F, Bin S, Iqbal A, He L, Wei S, Zheng H, et al. High sink capacity improves rice grain yield by promoting nitrogen and dry matter accumulation. Agronomy. 2022;12(7):1–16. [Google Scholar]
61. Suryanto P, Taryono, Supriyanta, Kastono D, Putra E, Widyawan M, et al. Assessment of soil quality parameters and yield of rice cultivars in Melaleuca cajuputi agroforestry system. Biodiversitas. 2020;21(8):3463–70. [Google Scholar]
62. Alam T, Suryanto P, Handayani S, Supriyanta, Wulandari R, Andhari A, et al. Soil quality measurement for sustainable soybean yield agroforestry system under different crop rotation models. Biodiversitas. 2022;23(12):6155–63. doi:10.13057/biodiv/d231209. [Google Scholar] [CrossRef]
63. Tomokowiak A, Bocianowski J, Kwiatek M, Kowalczewski P. Dependence of the heterosis effect on genetic distance, determined using various molecular markers. Open Life Sci. 2020;15(1):1–11. doi:10.1515/biol-2020-0001 [Google Scholar] [PubMed] [CrossRef]
Cite This Article
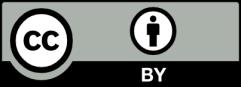
This work is licensed under a Creative Commons Attribution 4.0 International License , which permits unrestricted use, distribution, and reproduction in any medium, provided the original work is properly cited.