Open Access
ARTICLE
An Improved YOLOv8-Based Method for Real-Time Detection of Harmful Tea Leaves in Complex Backgrounds
College of Computer and Control Engineering, Northeast Forestry University, Harbin, 150040, China
* Corresponding Author: Jianping Huang. Email:
# Xin Leng and Jiakai Chen are listed as co-first authors
Phyton-International Journal of Experimental Botany 2024, 93(11), 2963-2981. https://doi.org/10.32604/phyton.2024.057166
Received 09 August 2024; Accepted 21 October 2024; Issue published 30 November 2024
Abstract
Tea, a globally cultivated crop renowned for its unique flavor profile and health-promoting properties, ranks among the most favored functional beverages worldwide. However, diseases severely jeopardize the production and quality of tea leaves, leading to significant economic losses. While early and accurate identification coupled with the removal of infected leaves can mitigate widespread infection, manual leaves removal remains time-consuming and expensive. Utilizing robots for pruning can significantly enhance efficiency and reduce costs. However, the accuracy of object detection directly impacts the overall efficiency of pruning robots. In complex tea plantation environments, complex image backgrounds, the overlapping and occlusion of leaves, as well as small and densely harmful leaves can all introduce interference factors. Existing algorithms perform poorly in detecting small and densely packed targets. To address these challenges, this paper collected a dataset of 1108 images of harmful tea leaves and proposed the YOLO-DBD model. The model excels in efficiently identifying harmful tea leaves with various poses in complex backgrounds, providing crucial guidance for the posture and obstacle avoidance of a robotic arm during the pruning process. The improvements proposed in this study encompass the Cross Stage Partial with Deformable Convolutional Networks v2 (C2f-DCN) module, Bi-Level Routing Attention (BRA), Dynamic Head (DyHead), and Focal Complete Intersection over Union (Focal-CIoU) Loss function, enhancing the model’s feature extraction, computation allocation, and perception capabilities. Compared to the baseline model YOLOv8s, mean Average Precision at IoU 0.5 (mAP0.5) increased by 6%, and Floating Point Operations Per second (FLOPs) decreased by 3.3 G.Keywords
Cite This Article
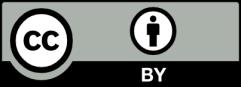
This work is licensed under a Creative Commons Attribution 4.0 International License , which permits unrestricted use, distribution, and reproduction in any medium, provided the original work is properly cited.