Open Access
ARTICLE
Integrated Transcriptomics and Metabolomics Analysis for the Mechanism Underlying White-to-Pink Petal Color Transition in Hibiscus mutabilis Flowers
College of Food and Biological Engineering, Chengdu University, Chengdu, 610016, China
* Corresponding Author: Xiaodong Shi. Email:
(This article belongs to the Special Issue: Plant Secondary Metabolism and Functional Biology)
Phyton-International Journal of Experimental Botany 2024, 93(10), 2571-2581. https://doi.org/10.32604/phyton.2024.056606
Received 26 July 2024; Accepted 20 September 2024; Issue published 30 October 2024
Abstract
Cotton rose (Hibiscus mutabilis) is a well-known ornamental plant that produces large flowers of vibrant colors. However, metabolites in H. mutabilis flowers with vibrant color have not been fully understood. By performing a combined analysis of metabolomics and transcriptomics data, we here explored mechanisms for the production of primary active compounds in this plant. Multivariate statistics unveiled differences in flavonoid metabolism between white and pink flowers, with pink flowers exhibiting a greater flavonoid abundance. The white-to-pink transition of cotton rose flowers may be attributed to pelargonidin-3-O-glucoside formation. On examining the expression of genes related to the structure of flavonoids, pink flowers were found to have a higher number of upregulated genes than white flowers, which resulted in higher flavonoid accumulation in the pink flowers. These results underscore the potential applications and development value of cotton rose flowers. Our study provides relevant insights into the regulation of key active components and the theoretical basis for the efficient utilization of cotton rose flowers.Graphic Abstract
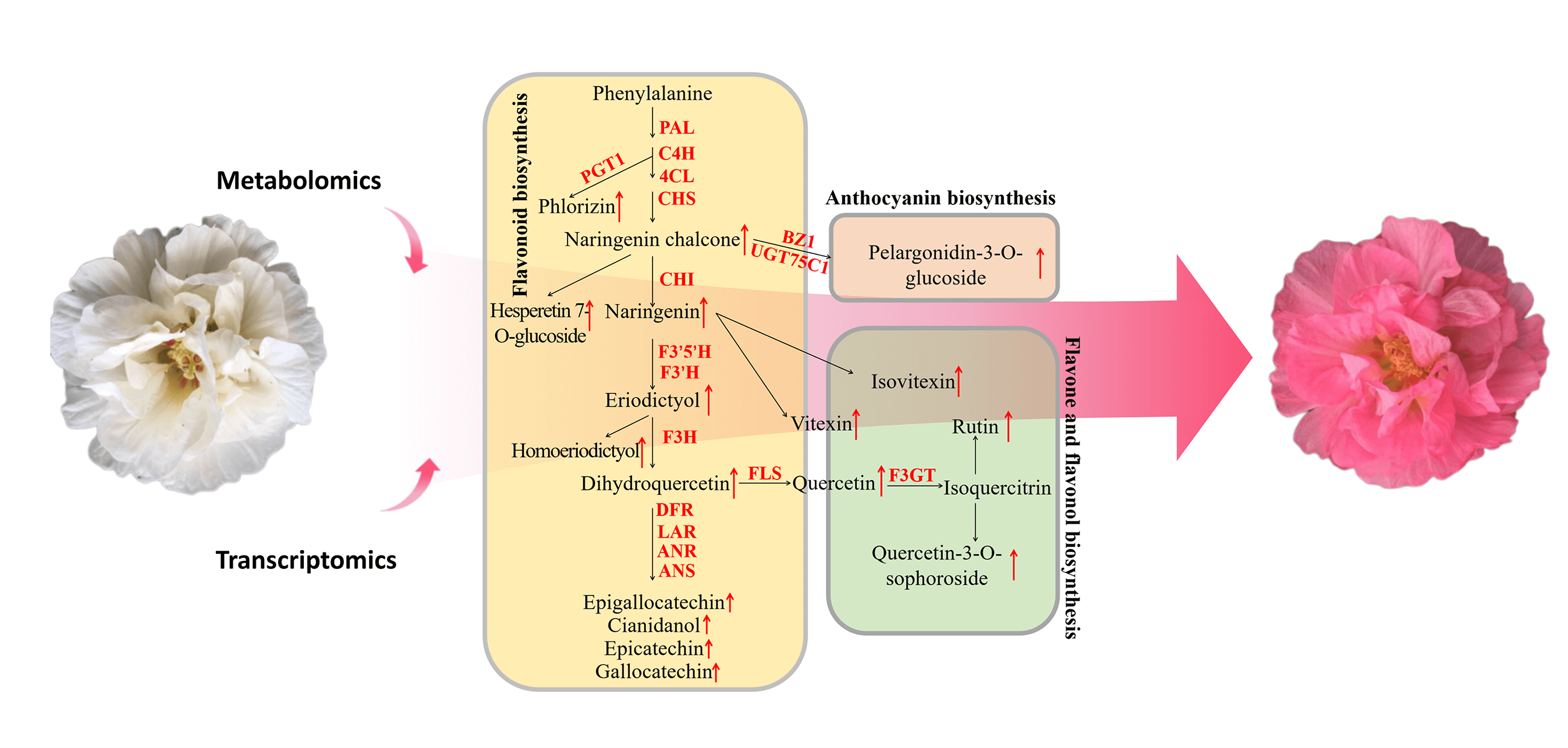
Keywords
Nomenclature
UHPLC-MS | Ultra high-performance chromatography-mass spectrometer |
DEG | Differentially expressed genes |
PCA | Principal component analysis |
OPLS-DA | Orthogonal partial least squares-discriminant analysis |
Hibiscus mutabilis (family Malvaceae), also known as cotton rose, is a deciduous shrub or small tree species with a long cultivation history in China, Japan, and Southeast Asia [1,2]. According to Chinese Materia Medica, cotton rose is beneficial in lowering body temperatures (e.g., fevers), detoxifying blood, and decreasing swelling. It is used to treat coughs, carbuncles, boils, and burns in clinics [3]. Cotton rose contains abundant bioactive substances, including flavonoids, organic acids, coumarins, and lignans. Among them, flavonoids (e.g., kaempferol, quercetin, isorhamnetin, and their flavonol glycosides) are the key effective compounds [4–6]. These compounds exhibit diverse pharmacological activities including anti-inflammatory, bacteriostatic, antioxidative, hypoglycemic, and immunomodulatory [7,8]. These therapeutic properties make cotton rose a highly valuable plant species in the medicine industry.
In addition to possessing high medicinal value, cotton rose serves as a crucial ornamental plant as it produces intensely colored flowers with a long blooming period [9]. For example, flowers of certain cotton rose varieties are pink-white in the morning and pink at noon. The flowers of many ornamental plants also exhibit medicinal properties and offer health benefits because of their bioactive constituents. For centuries, chrysanthemum tea, a flower-based infusion, has been extensively used to treat various medical conditions, including chest pain, high blood pressure, diabetes, and headaches [10]. Flowers of Osmanthus fragrans and Chrysanthemum morifolium are often employed as folk medicine and tea and beverage additives in China [11]. Flowers are rich sources of novel drugs, from which natural medicines are derived. Although cotton rose extracts are known to have numerous biological functions, information regarding its flower metabolites and their effects remains limited. Moreover, the material basis for the effects of cotton rose flowers and high diversity in flower colors remains unclear.
Metabolomics technology facilitates a holistic analysis and quantification of metabolites in integrated tissue systems, and, therefore, is instrumental to systems biology-based research [12]. High-throughput technologies have facilitated detailed investigations into the complexity of plant secondary metabolism. For example, through a metabolomic analysis of anthocyanin accumulation in white and pink tea (Camellia sinensis) flowers, Zhou et al. revealed the exclusive accumulation of three specific anthocyanins in pink tea flowers [13]. This finding is relevant for breeding tea cultivars with anthocyanin-rich pink flowers. Furthermore, a combination of metabolomics and other omics-based technologies is widely used to identify functional genes and characterize complex secondary metabolic bioprocesses in plants. Based on transcriptome and metabolome data, Mao et al. identified terpenoid and flavonoid biosynthesis-related genes in Pinus taeda [14]. An integrated analysis of the Magnolia champaca metabolome and transcriptome clarified the biosynthesis pathways of floral volatile organic compounds [15]. The study added to the existing transcriptome data for M. champaca, potentially enhancing the commercial value of this plant species in the flavor and fragrance industry. Analyzing the metabolome and transcriptome in combination is crucial for identifying and analyzing the mechanism underlying active compound synthesis in plants. By applying metabolomics techniques, we identified cotton rose metabolites. Finally, the diversity in the identified metabolites of differentially colored cotton rose flowers as well as the associated regulatory mechanism were investigated. We also identified bioactive compounds in the flowers and preliminarily characterized their formation mechanisms. The data presented herein will aid in the future application of cotton rose flowers for medicinal purposes.
Cotton rose plants with different flower colors (i.e., pink and white) were selected at Chengdu University (Chengdu City, Sichuan Province, China) and grown under natural conditions at the university campus. Fully bloomed white flowers were collected in the morning, whereas pink flowers were collected in the afternoon. All study materials were frozen in liquid nitrogen and stored at −80°C until use for the subsequent transcriptome sequencing analysis and metabolite extraction. These analyses were completed using three independent biological replicates to achieve reproducible results.
2.2 Extraction and Determination of Metabolites
Freeze-dried samples were ground using a mixer mill (60 Hz for 30 s) and precisely weighed. Then, 50 mg samples were transferred to separate Eppendorf tubes, to which 700 µL extraction solution (methanol/water = 3:1; precooled at −40°C) was added. The samples were vortexed for 30 s, homogenized at 35 Hz for 4 min, and sonicated for 5 min in an ice-water bath. The homogenization and sonication steps were repeated three times. The samples were incubated overnight at 4°C on a shaker and centrifuged at 12,000 rpm for 15 min at 4°C. The supernatant was filtered through a 0.22-µm microporous membrane, and the collected filtrate was diluted (1:10) in the methanol/water solution (3:1) containing the internal standard. After the diluted filtrate was vortexed for 30 s, the solution was transferred to 2-mL glass vials. Then, 50 µL aliquots of each sample were pooled from the vials to produce quality control samples. These samples were stored at −80°C and subjected to ultra high-performance chromatography–mass spectrometry (UHPLC–MS). The UHPLC separation was performed using an EXIONLC System (Sciex Technologies). A QTrap 6500+ system (Sciex Technologies) was used with the following typical ion source parameters: ion spray voltage: +5500/−4500 V; curtain gas: 35 psi; temperature: 400°C; ion source gas 1: 60 psi; ion source gas 2: 60 psi; and DP: ±100 V.
2.3 Transcriptome Data Assembly and Functional Annotation
After extracting total RNA from the frozen flowers by using the RNAprep Pure Plant Kit (Tiangen Biotech, Beijing, China), we generated sequencing libraries by using NEBNext® Ultra™ RNA Library Prep Kit for Illumina® (NEB, USA). Employing the Trinity program, the reads were fragmented (i.e., K-mers), extended into contigs, and then combined according to the overlapping sequences. Unigene sequences served as queries for BLAST searches of NR, Swiss-Prot, GO, COG, KOG, and eggNOG databases. Among the unigenes obtained from the assembly, the enriched KEGG pathways were identified using KOBAS 2.0. The unigene-encoded amino acid sequences were predicted and annotated based on a BLAST search of the Pfam database, which was conducted using HMMER. To obtain high-quality clean reads, raw reads in the fastq format were processed using in-house Perl scripts. This step eliminated the adapters, poly-N sequences, and low-quality sequences among the raw reads. Then, downstream analyses were performed using the high-quality clean reads obtained. The genes differentially expressed between groups were analyzed using DESeq2.
Differentially abundant metabolites were screened on the basis of the following criteria: the VIP > 1 in the orthogonal partial least squares-discriminant analysis (OPLS-DA) model, and p value of <0.05 in Student’s t-test. Using the Benjamini-Hochberg method, the p value obtained from the original hypothesis test was corrected for analyzing the differentially expressed genes (DEGs). The corrected p value was used for identifying significant DEGs according to fold-change ≥ 2 and FDR < 0.01.
3.1 Identification of Metabolites
The cotton rose flowers are large and showy and exhibit color transitions, turning white in the morning, and pink in the afternoon (Fig. 1). In the current study, a metabolomics analysis of the flowers yielded 872 metabolites, including flavonoids (136), alkaloids (112), coumarins (88), phenols (80), amino acids and derivatives (53), and other metabolites in the white and pink flower groups. The principal component analysis (PCA) reflected substantial diversity between the groups, with all samples within the 95% confidence interval (Hotelling’s T-squared ellipse). The three replicates for the two groups were located on either side of the PCA score scatter plot, whereas the quality control samples clustered in the center (Fig. 2A). The PCA indicated that the data were reproducible for the samples within the same group, and the metabolite contents differed between the two groups. Using the SIMCA software, we performed the OPLS-DA of the metabolites. The original model’s R2Y and Q2 were close to 1, which suggested that the established model was consistent with the sample data and thus can appropriately explain differences between the two groups. Moreover, the permuted model had a smaller Q2 value than the original model, indicating that the original model was robust and that no overfitting issue was present. In total, 124 differentially abundant metabolites were identified and ranked according to their log2 (fold-change) value. Of them, 93 and 31 metabolites were more and less abundant, respectively, in the pink flower samples than in the white flower samples (Fig. 2B). Adenosine, theaflavin, epigallocatechin, and epicatechin, the most variable differentially abundant metabolites, were more abundant in the pink flowers than in the white flowers. Following classification, the predominant differentially abundant metabolites were found to be flavonoids (31), amino acids (13), and alkaloids (10) (Fig. 2C). Furthermore, these metabolites were mapped to pathways in Arabidopsis, including 35 metabolic pathways. The pathways involving the most differentially abundant metabolites were related to flavonoid biosynthesis, amino acid biosynthesis, ABC transporters, aminoacyl-tRNA biosynthesis, and oxocarboxylic acid. The enriched pathways were closely related to the following biosynthesis processes: aminoacyl-tRNA biosynthesis, flavonoid biosynthesis, flavone and flavonol biosynthesis, and amino acid biosynthesis and metabolism (Fig. 3A). In total, 31 differentially abundant flavonoid metabolites were screened, namely 12 flavones, 7 catechin derivatives, 5 flavonols, 3 chalcones, 2 proanthocyanidins, 1 isoflavone, and 1 flavanone (Fig. 2C). Only Kuwanon H was less abundant in the pink flowers compared with the white flowers, whereas the other 30 flavonoid metabolites were more abundant. Hence, flavonoids are the key differentially abundant metabolites produced during pink cotton rose flower development. More specifically, the contents of these metabolites tended to increase as the flowers transitioned from white to pink.
Figure 1: The different floral color stages of H. mutabilis. From left to right, the flower color gradually turned pink
Figure 2: (A) PCA score plot for metabolite profiling. (B) Volcano plot of the differential metabolites between the pink and white flowers; up-regulated and down-regulated metabolites are shown in pink and blue colors, respectively. (C) Cluster heatmap of all differentially metabolites between the pink and white flowers; FP: pink flowers; FW: white flowers; QC: quality control samples
Figure 3: Enrichment analysis of KEGG pathways for the differential metabolites (A) and differentially expressed genes (B)
After eliminating low-quality data through the quality control procedure, 37.18 Gb clean data were obtained, with at least 5.93 Gb per sample. More than 93.23% of the bases had a quality score of Q30. The 129,028 unigenes obtained had an N50 of 1159 bp. These results confirmed that the transcriptome sequencing data were of high quality and thus appropriate for the subsequent analysis. Of the 19,121 DEGs identified, 8912 were upregulated and 10,209 were downregulated. Moreover, of the 16,792 annotated unigenes, 13,002 and 11,281 were annotated according to the GO and KEGG databases, respectively. According to the GO functional classification, the DEGs were enriched in 57 GO terms, with 20, 15, and 22 GO terms belonging to the cellular component, molecular function, and biological process categories, respectively. Among the DEGs, the top 10 GO terms were catalytic activity, cellular process, metabolic process, binding, cell, cell part, organelle, single organism process, membrane, and biological regulation. Furthermore, response to stimulus, oxidation-reduction process, and response to stress were found to be the three most enriched biological process subcategories, whereas the membrane, cell periphery, and obsolete cytoplasmic part were the three most enriched cellular component subcategories. Catalytic activity, transferase activity, and transporter activity were the three most significantly enriched molecular function subcategories. The DEGs identified in the flowers in different developmental stages were majorly related to cellular structures, enzyme activities, and processes mediating responses to external conditions. According to the KEGG pathway functional annotation and enrichment analysis, 11,281 DEGs were identified, and they were associated with 134 pathways. The key enriched KEGG pathways among the significant DEGs were carbon metabolism, plant hormone signal transduction, MAPK signaling pathway, plant-pathogen interaction, and ribosome (Fig. 3B). These enriched pathways were predominantly involved in the biosynthesis and metabolism of various compounds (e.g., phenylpropanoid, flavonoid, ubiquinone, and other terpenoid-quinone biosynthesis as well as starch, sucrose, linoleic acid, glycerolipid, alpha-linolenic acid, and amino acid metabolism). These KEGG results indicate that the genes related to the biosynthesis of certain compounds and metabolic regulation are crucial for the metabolite-based changes in the color of the cotton rose flowers from white to pink. Moreover, plant hormone signal transduction and brassinosteroid biosynthesis exert critical regulatory effects on the development of these flowers and the formation of their specific metabolites.
3.3 Association Analysis of Flavonoid Biosynthesis
We first mapped the differentially abundant flavonoid metabolites and DEGs identified in the white and pink cotton rose flowers to KEGG metabolic pathways, including flavonoid biosynthesis (ko00941), anthocyanin biosynthesis (ko00942), flavone and flavonol biosynthesis (ko00944), and phenylpropanoid biosynthesis (ko00940). The relationship between genes and flavonoid metabolites in the cotton rose flowers was examined by combining the results for the metabolites and genes and establishing a network (Fig. 4A). Overall, 78 DEGs were obtained, including those encoding 4-coumarate CoA ligase (4CL) (16), phenylalanine ammonia lyase (PAL) (13), cinnamate 4-hydroxylase (C4H) (7), and CHS (7), as well as 17 differentially accumulated flavonoids (Fig. 4B). According to the heatmap for the genes and metabolites, 65 and 13 of the 78 DEGs were upregulated and downregulated, respectively, in the pink flowers, which increased the flavonoid metabolite contents (Fig. 4C). Compared with the corresponding expression in the white flowers, the expression levels of all DEGs encoding phenylpropanoid biosynthesis-related PAL and C4H increased in the pink flowers, which promoted the formation of downstream secondary metabolites. Furthermore, the expression levels of seven CHS-encoding DEGs increased in the pink flowers. These changes in gene expression promoted the formation of the basic carbon structure of flavonoids and augmented the formation of flavonoids of different classes. Additionally, the increased expression of genes encoding F3’5’H, F3’H, F3H, and F3GT induced dihydroquercetin, quercetin, and rutin syntheses in the pink flowers. Two anthocyanin biosynthesis genes, BZ1 and UGT75C1, which were upregulated in the pink flowers, were associated with pelargonidin-3-O-glucoside formation.
Figure 4: Combined analysis of gene expression and metabolite changes in the flavonoid pathway. (A) Mechanisms underlying flavonoid biosynthesis in H. mutabilis flowers with different colors; this pathway was constructed based on the KEGG pathways. Heatmap of differential metabolites (B) and differentially expressed genes (C) in the white and pink flowers. The degree of change is marked with different colors: red indicates upregulation, and green represents downregulation
Multi-omics approaches involving transcriptome and metabolome analyses are widely used for mining plant metabolites and clarifying mechanisms underlying their formation. However, systematic research on compound formation in ornamental cotton rose flowers is lacking. In this study, we gathered data regarding metabolite contents and gene expression levels in cotton rose flowers by performing an integrated metabolome and transcriptome analysis. The study findings offer researchers reference materials to explore the potential utility of ornamental flowers in medicine.
The use of plants as medicines is influenced by certain factors, such as functional active ingredients, volatile aroma compounds, and essential oils present in their flowers [16]. For example, Lonicerae japonicae flower extracts contained chlorogenic acid, which exhibited potential as a novel therapeutic for preventing and treating heart failure [17]. Buckwheat flower extracts exerted antioxidant effects on brain and liver tissues [18]. In Eutrema japonicum, flavonoid glycosides were more abundant in the flowers than in the roots and leaves [19]. Therefore, E. japonicum floral extracts may possess effective antiaging compounds valuable for the cosmetics industry. Substantial amounts of phenolic compounds were detected in tree peony flowers, which imply that these flowers may be used as the raw material for producing functional foods and as a natural source of antioxidants [20]. In the current study examining active components in cotton rose flowers, flavonoids were identified as the most diverse class of metabolites. In total, 136 flavonoids, including rutin, quercetin, catechin, and naringenin, were identified. Moreover, 35 differentially abundant flavonoids accumulated more in the pink cotton rose flowers than in the white flowers, indicating that flavonoids may be the key active compounds in cotton rose flowers, especially in the pink flowers.
In addition to their other functions, flavonoids serve as determining factors mediating changes in plant colors. Fu et al. confirmed that the diversity in Camellia japonica flower colors is due to marked differences in the accumulation of various anthocyanins in their petals, including pelargonidin-3-O-glucoside, which is among the major anthocyanins responsible for dark coloration of petals [21]. According to Härtl et al., the red coloration of strawberry fruits, which is highly preferred by consumers, is caused by the accumulation of anthocyanins, especially pelargonidin-3-O-glucoside, 6’-O-malonated derivative, and cyanidin 3-O-glucoside [22]. In our study, the pelargonidin-3-O-glucoside content was significantly higher in the pink flowers than in the white flowers. This implied that its accumulation causes a change in the color of the cotton rose flowers from white to pink. In the flavonoid biosynthesis pathway, the first step involves phenylalanine and the reactions catalyzed by various enzymes, including PAL, C4H, CHS, and 4CL, which ultimately result in the production of various secondary metabolites [23–25]. CHS catalyzes the first step and produces naringenin chalcone, thus it is considered a gatekeeper in the regulation of flavonoid biosynthesis [23,26,27]. In this study, the upregulated DEGs in the pink flowers were associated with flavonoid accumulation. Additionally, DEGs encoding F3’5’H, F3’H, F3H, and F3GT were upregulated in the pink flowers, suggesting that they are the primary regulatory genes promoting flavonoid formation in the flowers. However, 13 DEGs encoding enzymes in the flavonoid biosynthesis pathway were downregulated in the pink flowers. This downregulation may be related to the functional redundancy induced by multi-copy genes and the effects of other regulators [9]. Although various flavonoids were detected in the cotton rose flowers, the genes encoding key enzymes in the flavonoid biosynthesis pathway were relatively conserved. These findings provide useful insights for future studies investigating the coloration mechanism of these flowers.
We combined metabolome and transcriptome data to decipher the molecular mechanisms underlying flower coloration in H. mutabilis. In total, 872 metabolites were identified, including flavonoids, alkaloids, coumarins, and phenols. Moreover, we screened 31 differentially abundant flavonoid metabolites. Except for one, all the identified metabolites were more abundant in the pink flowers than in the white flowers. Based on the integrated metabolomics and transcriptomics analysis, 78 DEGs were obtained, including those encoding flavonoid synthesis-mediating enzymes such as 4CL, PAL, C4H, and CHS. Our data showed that the accumulation of flavonoids correlated with strong up-regulation of flavonoids biosynthetic structural genes in the pink flower as compared to the white samples. Collectively, this study offers candidate genes for functional characterization and for manipulation of flower color in H. mutabilis.
Acknowledgement: We are grateful to Dr. Zhuang Guoqing in Sichuan Academy of Forestry Sciences.
Funding Statement: This work was financially supported by the college students’ Innovation and Entrepreneurship Training Program of Chengdu University (S202311079115, CDUCX2024599).
Author Contributions: The authors confirm contribution to the paper as follows: study conception, design and data collection: Xiaodong Shi; analysis and interpretation of results: Tingyu Wang; draft manuscript preparation: Sui Ai and Jiasi Li. All authors reviewed the results and approved the final version of the manuscript.
Availability of Data and Materials: These raw data can be found in NCBI with an accession number of PRJNA834628.
Ethics Approval: Not applicable.
Conflicts of Interest: The authors declare that they have no conflicts of interest to report regarding the present study.
References
1. Shi X, Li F, Liu X, Zhu Z, Zeng X, Li M, et al. Study on adaptability of Hibiscus mutabilis germplasm resources in Chengdu region. J Sichuan Forest Sci Technol. 2021;4:90–3 (In Chinese). [Google Scholar]
2. Xie JE, Shi LX, Zhu XY, Wang P, Zhao Y, Su W. Mechanochemical-assisted efficient extraction of rutin from Hibiscus mutabilis L. Innov Food Sci Emerg. 2011;12(2):146–52. doi:10.1016/j.ifset.2010.12.009. [Google Scholar] [CrossRef]
3. Liu D, Mei Q, Wan X, Que H, Li L, Wan D. Determination of rutin and isoquercetin contents in Hibisci mutabilis Folium in different collection periods by HPLC. J Chromatogr Sci. 2015;53(10):1680–4. doi:10.1093/chromsci/bmv071. [Google Scholar] [PubMed] [CrossRef]
4. Wang Y, Feng L, Huang L, Hu P, Yan X, Wang L, et al. Rapid identification on chemical constituents of Hibiscus mutabilis flowers by UPLC-Q-Orbitrap HRMS. Nat Prod Res. 2021;33(12):2042–52 (In Chinese). [Google Scholar]
5. Wang Y, Ye L, Hu P, Xia H. Research progress on chemical constituents, pharmacological effects and extraction technology of total flavonoids from Hibiscus Mutabilis. Shandong Chem Ind. 2022;12:80–6 (In Chinese). [Google Scholar]
6. Xu Y, Yue XW, Wang YJ, Yang HL, Di KX, Bao HW. Simultaneous determination of content and antioxidant activity of five components in Hibiscus mutabilis L by HPLC-QAMS. J Chem. 2022;2022:8845760. [Google Scholar]
7. Hostetler GL, Ralston RA, Schwartz SJ. Flavones: food sources, bioavailability, metabolism, and bioactivity. Adv Nutr. 2017;8(3):423–35. doi:10.3945/an.116.012948. [Google Scholar] [PubMed] [CrossRef]
8. Song F, Tang M, Wang H, Zhang Y, Zhu K, Chen X, et al. UHPLC-MS/MS identification, quantification of flavonoid compounds from Areca catechu L. extracts and in vitro evaluation of antioxidant and key enzyme inhibition properties involved in hyperglycemia and hypertension. Ind Crop Prod. 2022;189:115787. doi:10.1016/j.indcrop.2022.115787. [Google Scholar] [CrossRef]
9. Yang YZ, Liu XD, Shi XQ, Ma J, Zeng XM, Zhu ZS, et al. A high-quality, chromosome-level genome provides insights into determinate flowering time and color of cotton rose (Hibiscus mutabilis). Front Plant Sci. 2022;13:818206. doi:10.3389/fpls.2022.818206. [Google Scholar] [PubMed] [CrossRef]
10. Wang YX, Xu ZZ, Wu Y, Li M, Pang SC, Liang Z, et al. Effect of weakly basic conditions on the separation and purification of flavonoids and glycosides from Chrysanthemum morifolium Tea. Molecules. 2019;24(2):297. doi:10.3390/molecules24020297. [Google Scholar] [PubMed] [CrossRef]
11. Tsai P, Chang M, Hsin C, Chuang C, Chuang L, Wu W. Antilipotoxicity activity of Osmanthus fragrans and Chrysanthemum morifolium flower extracts in hepatocytes and renal glomerular mesangial cells. Mediat Inflamm. 2017;2017(1):4856095. doi:10.1155/2017/4856095. [Google Scholar] [PubMed] [CrossRef]
12. Viant MR, Ebbels MD, Beger RD, Ekman DR, Epps JT, Kamp H, et al. Use cases, best practice and reporting standards for metabolomics in regulatory toxicology. Nat Commun. 2019;10:3041. doi:10.1038/s41467-019-10900-y. [Google Scholar] [PubMed] [CrossRef]
13. Zhou CB, Mei X, Rothenberg DO, Yang ZB, Zhang WT, Wan SH, et al. Metabolome and transcriptome analysis reveals putative genes involved in anthocyanin accumulation and coloration in white and pink tea (Camellia sinensis) flower. Molecules. 2020;25(1):190. doi:10.3390/molecules25010190. [Google Scholar] [PubMed] [CrossRef]
14. Mao J, Huang L, Chen M, Zeng W, Feng Z, Huang S, et al. Integrated analysis of the transcriptome and metabolome reveals genes involved in terpenoid and flavonoid biosynthesis in the loblolly pine (Pinus taeda L.). Front Plant Sci. 2021;12:729161. doi:10.3389/fpls.2021.729161. [Google Scholar] [PubMed] [CrossRef]
15. Dhandapani S, Jin J, Sridhar V, Sarojam R, Chua NH, Jang IC. Integrated metabolome and transcriptome analysis of Magnolia champaca identifies biosynthetic pathways for floral volatile organic compounds. BMC Genomics. 2017;18(1):463. doi:10.1186/s12864-017-3846-8. [Google Scholar] [PubMed] [CrossRef]
16. Zhao JL, Jiang L, Tang XH, Peng LX, Li X, Zhao G, et al. Chemical composition, antimicrobial and antioxidant activities of the flower volatile oils of Fagopyrum esculentum, Fagopyrum tataricum and Fagopyrum cymosum. Molecules. 2018;23(1):182. doi:10.3390/molecules23010182. [Google Scholar] [PubMed] [CrossRef]
17. Tian L, Su CP, Wang Q, Wu FJ, Bai R, Zhang HM, et al. Chlorogenic acid: a potent molecule that protects cardiomyocytes from TNF-α-induced injury via inhibiting NF-κB and JNK signals. J Cell Mol Med. 2019;23(7):4666–78. doi:10.1111/jcmm.2019.23.issue-7. [Google Scholar] [CrossRef]
18. Sadauskiene I, Liekis A, Bernotiene R, Sulinskiene J, Kasauskas A, Zekonis G. The effects of buckwheat leaf and flower extracts on antioxidant status in mouse organs. Oxid Med Cell Longev. 2018;2018:6712407. doi:10.1155/omcl.v2018.1. [Google Scholar] [CrossRef]
19. Szewczyk K, Pietrzak W, Klimek K, Miazga-Karska M, Firlej A, Flisinski M, et al. Flavonoid and phenolic acids content and in vitro study of the potential anti-aging properties of Eutrema japonicum (Miq.) Koidz cultivated in Wasabi Farm Poland. Int J Mol Sci. 2021;22(12):6219. doi:10.3390/ijms22126219. [Google Scholar] [PubMed] [CrossRef]
20. Xiang JL, Yang CB, Beta T, Liu SX, Yang RQ. Phenolic profile and antioxidant activity of the edible tree peony flower and underlying mechanisms of preventive effect on H2O2-induced oxidative damage in Caco-2 cells. Foods. 2019;8(10):471. doi:10.3390/foods8100471. [Google Scholar] [PubMed] [CrossRef]
21. Fu M, Yang X, Zheng J, Wang L, Yang X, Tu Y, et al. Unraveling the regulatory mechanism of color diversity in Camellia japonica petals by integrative transcriptome and metabolome analysis. Front Plant Sci. 2021;12:685136. doi:10.3389/fpls.2021.685136. [Google Scholar] [PubMed] [CrossRef]
22. Härtl K, Denton A, Franz-Oberdorf K, Hoffmann T, Spornraft M, Usadel B, et al. Early metabolic and transcriptional variations in fruit of natural white-fruited Fragaria vesca genotypes. Sci Rep. 2017;7:45113. doi:10.1038/srep45113. [Google Scholar] [PubMed] [CrossRef]
23. Alberstein M, Eisenstein M, Abeliovich H. Removing allosteric feedback inhibition of tomato 4-coumarate: coA ligase by directed evolution. Plant J. 2012;69(1):57–69. doi:10.1111/tpj.2011.69.issue-1. [Google Scholar] [CrossRef]
24. Falcone ML, Rius SP, Casati P. Flavonoids: biosynthesis, biological functions, and biotechnological applications. Front Plant Sci. 2012;3:222. doi:10.3389/fpls.2012.00222. [Google Scholar] [PubMed] [CrossRef]
25. Vogt T. Phenylpropanoid biosynthesis. Mol Plant. 2010;3(1):2–20. doi:10.1093/mp/ssp106. [Google Scholar] [PubMed] [CrossRef]
26. Brenda WS. Flavonoid biosynthesis. a colorful model for genetics, biochemistry, cell biology, and biotechnology. Plant Physiol. 2001;126(2):485–93. doi:10.1104/pp.126.2.485. [Google Scholar] [PubMed] [CrossRef]
27. Broun P. Transcriptional control of flavonoid biosynthesis: a complex network of conserved regulators involved in multiple aspects of differentiation in Arabidopsis. Curr Opin Plant Biol. 2005;8(3):272–9. doi:10.1016/j.pbi.2005.03.006. [Google Scholar] [PubMed] [CrossRef]
Cite This Article
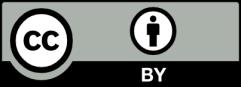
This work is licensed under a Creative Commons Attribution 4.0 International License , which permits unrestricted use, distribution, and reproduction in any medium, provided the original work is properly cited.