Open Access
ARTICLE
Monitoring Thosea sinensis Walker in Tea Plantations Based on UAV Multi-Spectral Image
1 School of Information Engineering, Zhejiang University of Water Resources and Electric Power, Hangzhou, 310018, China
2 College of Artificial Intelligence, Hangzhou Dianzi University, Hangzhou, 310018, China
3 Tea Research Institute, College of Agriculture and Biotechnology, Zhejiang University, Hangzhou, 310058, China
* Corresponding Author: Yao Zhang. Email:
(This article belongs to the Special Issue: Symbiotic Associations for Nutrients Management and Complexes Formation for Better Agricultural Crops Productivity under Biotic and Abiotic Stresses)
Phyton-International Journal of Experimental Botany 2023, 92(3), 747-761. https://doi.org/10.32604/phyton.2023.025502
Received 17 July 2022; Accepted 05 September 2022; Issue published 29 November 2022
Abstract
Thosea sinensis Walker (TSW) rapidly spreads and severely damages the tea plants. Therefore, finding a reliable operational method for identifying the TSW-damaged areas via remote sensing has been a focus of a research community. Such methods also enable us to calculate the precise application of pesticides and prevent the subsequent spread of the pests. In this work, based on the unmanned aerial vehicle (UAV) platform, five band images of multispectral red-edge camera were obtained and used for monitoring the TSW in tea plantations. By combining the minimum redundancy maximum relevance (mRMR) with the selected spectral features, a comprehensive spectral selection strategy was proposed. Then, based on the selected spectral features, three classic machine learning algorithms, including random forest (RF), support vector machine (SVM), and k-nearest neighbors (KNN) were used to construct the pest monitoring model and were evaluated and compared. The results showed that the strategy proposed in this work obtained ideal monitoring accuracy by only using the combination of a few optimized features (2 or 4). In order to differentiate the healthy and TSW-damaged areas (2-class model), the monitoring accuracies of all the three models were computed, which were above 96%. The RF model used the least number of features, including only SAVI and Bandred. In order to further discriminate the pest incidence levels (3-class model), the monitoring accuracies of all the three models were computed, which were above 80%, among which the RF algorithm based on SAVI, Bandred, VARI_green, and Bandred_edge features achieve the highest accuracy (OAA of 87%, and Kappa of 0.79). Considering the computational cost and model accuracy, this work recommends the RF model based on a few optimal feature combinations to monitor and distinguish the severity of TSW in tea plantations. According to the UAV remote sensing mapping results, the TSW infestation exhibited an aggregated distribution pattern. The spatial information of occurrence and severity can offer effective guidance for precise control of the pest. In addition, the relevant methods provide a reference for monitoring other leaf-eating pests, effectively improving the management level of plant protection in tea plantations, and guaranting the yield and quality of tea plantations.Keywords
Cite This Article
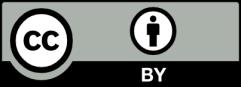
This work is licensed under a Creative Commons Attribution 4.0 International License , which permits unrestricted use, distribution, and reproduction in any medium, provided the original work is properly cited.