Open Access
ARTICLE
Tea Plantation Frost Damage Early Warning Using a Two-Fold Method for Temperature Prediction
1 College of Artificial Intelligence, Hangzhou Dianzi University, Hangzhou, 310018, China
2 School of Information Engineering and Art and Design, Zhejiang University of Water Resources and Electric Power, Hangzhou, 310018, China
* Corresponding Authors: Xianfeng Zhou. Email: ; Dongmei Chen. Email:
(This article belongs to the Special Issue: Plant–Environment Interactions)
Phyton-International Journal of Experimental Botany 2022, 91(10), 2269-2282. https://doi.org/10.32604/phyton.2022.022607
Received 18 March 2022; Accepted 11 April 2022; Issue published 30 May 2022
Abstract
As the source and main producing area of tea in the world, China has formed unique tea culture, and achieved remarkable economic benefits. However, frequent meteorological disasters, particularly low temperature frost damage in late spring has seriously threatened the growth status of tea trees and caused quality and yield reduction of tea industry. Thus, timely and accurate early warning of frost damage occurrence in specific tea garden is very important for tea plantation management and economic values. Aiming at the problems existing in current meteorological disaster forecasting methods, such as difficulty in obtaining massive meteorological data, large amount of calculation for predicted models and incomplete information on frost damage occurrence, this paper proposed a two-fold algorithm for short-term and real-time prediction of temperature using field environmental data, and temperature trend results from a nearest local weather station for accurate frost damage occurrence level determination, so as to achieve a specific tea garden frost damage occurrence prediction in a microclimate. Time-series meteorological data collected from a small weather station was used for testing and parameterization of a two-fold method, and another dataset acquired from Tea Experimental Base of Zhejiang University was further used to validate the capability of a two-fold model for frost damage forecasting. Results showed that compared with the results of autoregressive integrated moving average (ARIMA) and multiple linear regression (MLR), the proposed two-fold method using a second order Furrier fitting model and a K-Nearest Neighbor model (K = 3) with three days historical temperature data exhibited excellent accuracy for frost damage occurrence prediction on consideration of both model accuracy and computation (98.46% forecasted duration of frost damage, and 95.38% for forecasted temperature at the onset time). For field test in a tea garden, the proposed method accurately predicted three times frost damage occurrences, including onset time, duration and occurrence level. These results suggested the newly-proposed two-fold method was suitable for tea plantation frost damage occurrence forecasting.Keywords
Cite This Article
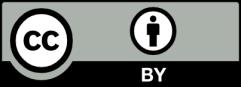