Open Access
ARTICLE
Detection and Discrimination of Tea Plant Stresses Based on Hyperspectral Imaging Technique at a Canopy Level
1 College of Artificial Intelligence, Hangzhou Dianzi University, Hangzhou, 310018, China
2 Zhejiang University of Water Resources and Electric Power, Hangzhou, 310018, China
3 Zhejiang Agricultural Technology Extension Center, Hangzhou, 310020, China
* Corresponding Author: Jingcheng Zhang. Email:
Phyton-International Journal of Experimental Botany 2021, 90(2), 621-634. https://doi.org/10.32604/phyton.2021.015511
Received 23 December 2020; Accepted 21 January 2021; Issue published 07 February 2021
Abstract
Tea plant stresses threaten the quality of tea seriously. The technology corresponding to the fast detection and differentiation of stresses is of great significance for plant protection in tea plantation. In recent years, hyperspectral imaging technology has shown great potential in detecting and differentiating plant diseases, pests and some other stresses at the leaf level. However, the lack of studies at canopy level hampers the detection of tea plant stresses at a larger scale. In this study, based on the canopy-level hyperspectral imaging data, the methods for identifying and differentiating the three commonly occurred tea stresses (i.e., the tea leafhopper, anthrax and sun burn) were studied. To account for the complexity of the canopy scenario, a stepwise detecting strategy was proposed that includes the process of background removal, identification of damaged areas and discrimination of stresses. Firstly, combining the successive projection algorithm (SPA) spectral analysis and K-means cluster analysis, the background and overexposed non-plant regions were removed from the image. Then, a rigorous sensitivity analysis and optimization were performed on various forms of spectral features, which yielded optimal features for detecting damaged areas (i.e., YSV, Area, GI, CARI and NBNDVI) and optimal features for stresses discrimination (i.e., MCARI, CI, LCI, RARS, TCI and VOG). Based on this information, the models for identifying damaged areas and those models for discriminating different stresses were established using K-nearest neighbor (KNN), Random Forest (RF) and Fisher discriminant analysis. The identification model achieved an accuracy over 95%, and the discrimination model achieved an accuracy over 93% for all stresses. The results suggested the feasibility of stress detection and differentiation using canopy-level hyperspectral imaging techniques, and indicated the potential for its extension over large areas.Keywords
Cite This Article
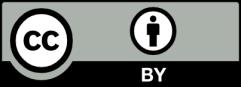
This work is licensed under a Creative Commons Attribution 4.0 International License , which permits unrestricted use, distribution, and reproduction in any medium, provided the original work is properly cited.