Open Access
ARTICLE
Deep learning identification of novel autophagic protein-protein interactions and experimental validation of Beclin 2-Ubiquilin 1 axis in triple-negative breast cancer
1 State Key Laboratory of Southwestern Chinese Medicine Resources, School of Pharmacy and College of Medical Technology, Chengdu University of Traditional Chinese Medicine, Chengdu, 611137, China
2 Department of Biotherapy, Cancer Center and State Key Laboratory of Biotherapy, West China Hospital, Sichuan University, Chengdu, 610041, China
* Corresponding Authors: WEI HUANG. Email: ; BO LIU. Email:
# These two authors contributed equally to this work
Oncology Research 2025, 33(1), 67-81. https://doi.org/10.32604/or.2024.055921
Received 10 July 2024; Accepted 23 October 2024; Issue published 20 December 2024
Abstract
Background: Triple-negative breast cancer (TNBC), characterized by its lack of traditional hormone receptors and HER2, presents a significant challenge in oncology due to its poor response to conventional therapies. Autophagy is an important process for maintaining cellular homeostasis, and there are currently autophagy biomarkers that play an effective role in the clinical treatment of tumors. In contrast to targeting protein activity, intervention with protein-protein interaction (PPI) can avoid unrelated crosstalk and regulate the autophagy process with minimal interference pathways. Methods: Here, we employed Naive Bayes, Decision Tree, and k-Nearest Neighbors to elucidate the complex PPI network associated with autophagy in TNBC, aiming to uncover novel therapeutic targets. Meanwhile, the candidate proteins interacting with Beclin 2 were initially screened in MDA-MB-231 cells using Beclin 2 as bait protein by immunoprecipitation-mass spectrometry assay, and the interaction relationship was verified by molecular docking and CO-IP experiments after intersection. Colony formation, cellular immunofluorescence, cell scratch and 3-(4,5-Dimethylthiazol-2-yl)-2,5-diphenyltetrazolium bromide (MTT) tests were used to predict the clinical therapeutic effects of manipulating candidate PPI. Results: By developing three PPI classification models and analyzing over 13,000 datasets, we identified 3733 previously unknown autophagy-related PPIs. Our network analysis revealed the central role of Beclin 2 in autophagy regulation, uncovering its interactions with 39 newly identified proteins. Notably, the CO-IP studies identified the substantial interaction between Beclin 2 and Ubiquilin 1, which was anticipated by our model and discovered in immunoprecipitation-mass spectrometry assay results. Subsequently, in vitro investigations showed that overexpressing Beclin 2 increased Ubiquilin 1, promoted autophagy-dependent cell death, and inhibited proliferation and metastasis in MDA-MB-231 cells. Conclusions: This study not only enhances our understanding of autophagy regulation in TNBC but also identifies the Beclin 2-Ubiquilin 1 axis as a promising target for precision therapy. These findings open new avenues for drug discovery and offer inspiration for more effective treatments for this aggressive cancer subtype.Keywords
Supplementary Material
Supplementary Material FileCite This Article
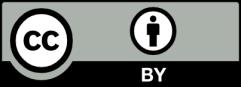
This work is licensed under a Creative Commons Attribution 4.0 International License , which permits unrestricted use, distribution, and reproduction in any medium, provided the original work is properly cited.