Open Access
REVIEW
Clinical implications of single cell sequencing for bladder cancer
1 UroScience, School of Medical Sciences, University of Campinas, UNICAMP, Campinas, Sao Paulo, 13083-872, Brazil
2 ImmunOncology, Pontifical Catholic University of Campinas, PUC-Campinas, Campinas, Sao Paulo, 13087-571, Brazil
* Corresponding Author: LEONARDO OLIVEIRA REIS. Email:
# These authors contributed equally
Oncology Research 2024, 32(4), 597-605. https://doi.org/10.32604/or.2024.045442
Received 27 August 2023; Accepted 08 January 2024; Issue published 20 March 2024
Abstract
Bladder cancer (BC) is the 10th most common cancer worldwide, with about 0.5 million reported new cases and about 0.2 million deaths per year. In this scoping review, we summarize the current evidence regarding the clinical implications of single-cell sequencing for bladder cancer based on PRISMA guidelines. We searched PubMed, CENTRAL, Embase, and supplemented with manual searches through the Scopus, and Web of Science for published studies until February 2023. We included original studies that used at least one single-cell technology to study bladder cancer. Forty-one publications were included in the review. Twenty-nine studies showed that this technology can identify cell subtypes in the tumor microenvironment that may predict prognosis or response to immune checkpoint inhibition therapy. Two studies were able to diagnose BC by identifying neoplastic cells through single-cell sequencing urine samples. The remaining studies were mainly a preclinical exploration of tumor microenvironment at single cell level. Single-cell sequencing technology can discriminate heterogeneity in bladder tumor cells and determine the key molecular properties that can lead to the discovery of novel perspectives on cancer management. This nascent tool can advance the early diagnosis, prognosis judgment, and targeted therapy of bladder cancer.Graphical Abstract
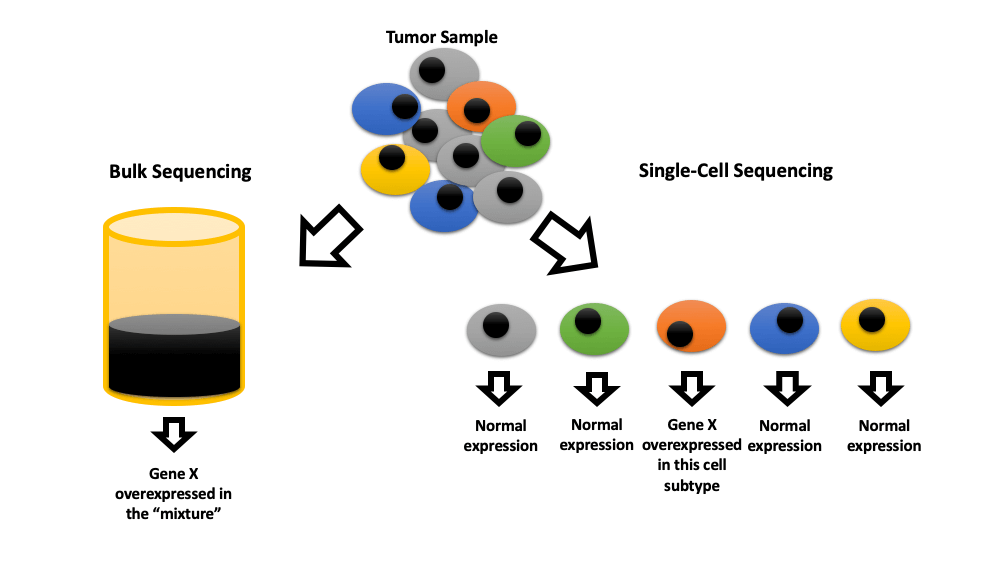
Keywords
Supplementary Material
Supplementary Material FileAbbreviations
BC | Bladder cancer |
CAF | Cancer associated fibroblast |
ICI | Immune checkpoint inhibitor |
scRNA seq | Single cell RNA sequencing |
snRNA seq | Single nucleus rna sequencing |
TAM | Tumor associated macrophages |
TME | Tumor microenvironment |
Single-cell sequencing technologies were pioneered in the early 90s and gained widespread attention in 2013 when they were named “Nature Methods”. These technologies are superior to traditional bulk sequencing methods due to their ability to map heterogenicity among individual cells of the same tissue. In short, these technologies isolate cells before administrating highly sensitive next-generation sequencing (NGS), which can sequence the very small amount of material available at the single-cell level. There are numerous techniques for isolating cells. The simplest method is separating cells one by one by micropipette under a microscope. The limiting dilution technique involves separating and suspending the cells in liquid before distributing the suspension in multi-well plates at the concentration of 0.5 cells per well. Microfluidic separation is a more advanced high throughput technique with relatively low cost. Single-nucleus separating is sometimes used instead of whole-cell separating to increase the durability of samples [1,2].
RNA is the most commonly sequenced molecule in these technologies (RNAseq), allowing researchers to map gene activity at the time of biopsy in each cell (transcriptional variability). In the field of oncology, this allows for the assessment of the tumor microenvironment (TME) at the single-cell level. Whole-exome sequencing (WES) maps the functional DNA and whole-genome sequencing (WGS) maps the entire DNA. Targeted NGS (tNGS) maps a specific panel of related genes that may be altered in single cells. T cell receptor sequencing (TCR-seq) maps the TCR-related RNA activity of single T cells [2–4]. Recently, NGS has been combined with the detection of other molecules such as proteins to concomitantly map them on individual cells (multi-omics). Chromatin immunoprecipitation (CHIP-seq), REAP-seq (RNA and surface protein), CITE-seq, and QuRIE-seq (RNA and epitopes) are among these methods [5–8].
Bladder cancer (BC) accounts for 3% of all new cancer diagnoses with 0.5 million new cases and 0.2 million deaths per year [9]. It is the 13th leading cause of cancer-related death in the world [10]. The 5-year overall survival rate for BC can be as high as 77% in non-metastatic disease and only 6% in metastatic BC. This cancer is four times more common in men (9.6 per 100,000) than in women (2.4 per 100,000). Tobacco smoking, positive family history, and exposure to chemicals are the main risk factors for this malignancy [11]. The frequency of BC rises with age; more than 90% of diagnoses occur in patients over 55 years of age [11]. Bladder cancer is classified into muscle-invasive bladder cancer (MIBC) and non-muscle-invasive bladder cancer (NMIBC) [9,12]. In addition to surgery, patients may receive radiotherapy, chemotherapy with mitomycin C, epirubicin, thiotepa, gemcitabine, platinum-based agents, doxorubicin, Bacillus Calmette Guerin (BCG) immunotherapy, and Immune Checkpoint Inhibitor (ICI) THERAPY [13]. Despite advances in available therapeutic methods, the clinical outcome of patients with bladder cancer remains unsatisfactory.
In this review, we summarize the clinical implications of single-cell sequencing technologies in bladder cancer.
The design and implementation of this scoping review were guided by Arksey et al.’s [14] methodological framework, described by the Joanna Briggs Institute [15]. To organize the information, the PRISMA model [16] was used and we followed the PRISMA-ScR recommendations [17].
Inclusion and exclusion criteria
This review focuses on the clinically significant application of single-cell sequencing technologies in bladder cancer. Inclusion criteria are in vitro or in vivo studies involving single-cell sequencing in bladder cancer published in any language. Exclusion criteria are letters, reviews, conference abstracts, editorials, unrelated articles, replies, unpublished articles, and articles without full-text access.
A comprehensive search of the literature published until February 2023 was conducted using Emtree language and medical subject headings (MeSh), in Pubmed, Embase, and the Cochrane Central Register of Controlled Trials (CENTRAL) databases. A broad search strategy was performed using a combination of the following search terms: ((urothelial cancer OR bladder cancer) AND single-cell sequencing). We supplemented this search with manual searches of the relevant articles identified through the Scopus, and Web of Science. Details of the search strategy are available in Appendix 1.
We used the Rayyan platform to screen articles and extract data. The software automatically removed duplicates; the remaining exclusions were manually performed by two researchers. Titles and abstracts were separately screened by the first and third authors with a portion of articles double screened for application of inclusion and exclusion criteria. The second author separately screened all articles. Data were extracted by the first three authors.
Our initial search resulted in 247 citations (187 citations from electronic databases and 60 citations from other sources). After removing the duplicates, the first two authors independently reviewed the remaining 180 titles and extracted those that clearly did not meet the inclusion criteria. The first three authors independently reviewed the abstracts of 135 potentially eligible studies. The full text of the remaining 66 studies was obtained and evaluated in accordance with the inclusion criteria. Any disagreement over inclusion was resolved by discussion. Finally, 41 studies were included in the review (Fig. 1).
Figure 1: Process of identification and inclusion of studies—PRISMA flow diagram.
Table 1 summarizes the included articles. The first 19 articles performed single-cell sequencing on samples acquired from patient tumors [18–36]. Five studies performed this technique in vitro or on animal BC models [37–41]. The remaining used public database [42–58]. All studies classified single cells and defined cell clusters contributing to the TME of BC. They identified clinically important cell subtypes by analyzing patient outcomes or responses to treatment.
Public databases: 29 articles analyzed public cancer databases as part of their study. Gene Expression Omnibus (GEO) [59] is currently the largest database containing single-cell sequencing data of cancer patients. Other databases such as The Cancer Genome Atlas project (TCGA) [60], ArrayExpress [61], and Genotype Tissue Expression project (GTEx) [62] mainly contain bulk sequencing data. TISCH [63] and The Cancer Single-Cell State Atlas (CancerSEA) [64] are lesser-known public databases of single-cell RNA sequences focusing on TME. The website of CellPress provides a review of highlighted reveals by these pooled public databases [65].
Fourteen studies analyzed these publicly available databases to generate a hypothesis [42–56]. Nine studies validated their hypothesis by in-house experiments [42–50]. Five studies validated their hypothesis by analyzing other databases [51–55].
Bladder cancer tends to be a heterogeneous tumor [66]. Nine studies investigated this heterogeneity by single-cell analysis of BC tumors and normal adjacent tissue (NAT). They classified cells into clusters and showed their ancestral origin [31–36,38,39,56].
The clinical implication of single-cell sequencing is evident in seven studies [18,19,25,32,42,55,56]. These studies show that separating cells of a patient’s tumor and characterizing cell subtypes can improve clinical decision-making by predicting the tumor’s response to different treatments.
Current single-cell sequencing techniques are extremely complex and involve multiple steps. The process of separating cells could damage the cell contents. Two studies addressed this issue by performing single-nucleus separation instead of whole-cell separation; the nucleus is less prone to degradation during the separation procedure [18,20].
Analyzing the high-dimensional data generated by single-cell sequencing is extremely complex and suffers from “the curse of dimensionality” [67], i.e., too much information [2,3,68–70]. Most studies addressed this issue by filtering for genes of interest as the first step of their analysis. For example, Zhou et al. [42] gathered a list of senescence-related genes from the website CellAge (http://genomics.senescence.info/cells) and only investigated related data in the TCGA database to generate their B cell senescence-related gene signature (Table 1). Other studies addressed this complexity through the reduction of dimensions (excluding insignificant genes). The most common dimension reduction techniques were clustering using the R package Seurat and visualizing using t-SNE [71].
Public databases such as TCGA and GEO pool data from various sequencing studies without providing sufficient data on heterogeneity among their techniques and patient factors. This is less important when sequencing DNA since DNA is a stable molecule designed by nature to maintain its integrity throughout an animal’s life. However, RNA sequencing is tricky since RNA is a transient molecule, only expressed when the cell requires it and degraded fairly rapidly. At room temperature, mRNA has a half-life of 16 h [72]. It is assumed that all RNA sequencing data pooled in these databases are acquired from analyzing fresh samples using similar techniques. However, a sentence in the article by Banchereau et al. (Table 1) casts doubt on this assumption: “…a bulk RNAseq analysis was performed on formalin-fixed paraffin-embedded tumor tissue”. Banchereau et al. experiments are a major contributor to IMvigor studies used by many articles included in this review [32].
It is important to keep in mind that transcription in cells is highly dependent on environmental factors. Temperature, oxygen level, stress, and even the circadian rhythm (time of the day) can influence transcription. Many commonly used medications such as steroids directly affect transcription [73–76]. As Yao et al. showed (Table 1), the over-the-counter drug acetaminophen influences the expression level of the gene, they found to be associated with BC tumor grade [52].
Single-cell analysis is a relatively new tool that aids in understanding the complexity of bladder cancer tumor’s microenvironment. Included studies in our scoping review collectively show that identifying certain cell subtypes in these heterogenous tumors may help clinicians in predicting response to expensive or invasive therapies such as immune checkpoint inhibitors or surgery with or without neoadjuvant chemotherapy. However, there are currently no clinical trials showing the benefit of performing this test on the overall well-being of the patients. Future single-cell sequencing studies should include epidemiological data, environmental factors, as well as comprehensive clinical data to improve our understanding of bladder cancer etiology and its heterogenous response to treatment.
Acknowledgement: Not applicable.
Funding Statement: This review did not receive any funding.
Author Contributions: R.Y.: Literature Search, Methodology, Literature Review, Data Extraction and Manuscript Writing; M.J., F.A.V.D., K.B., P.A.F.L., L.S.B.D.C., C.F.G., A.D.A., and N.B.: Literature Review, Data Extraction and Manuscript Writing; L.O.R.: Guidance, Editing, Final Review and Submission. All authors have read and agreed to the published version of the manuscript.
Availability of Data and Materials: Not applicable.
Ethics Approval: Not applicable.
Conflicts of Interest: The authors declare that they have no conflicts of interest to report regarding the present study.
Supplementary Materials: The supplementary material is available online at https://doi.org/10.32604/or.2024.045442.
References
1. Ding, J., Adiconis, X., Simmons, S. K., Kowalczyk, M. S., Hession, C. C. et al. (2020). Systematic comparison of single-cell and single-nucleus RNA-sequencing methods. Nature Biotechnology, 38(6), 737–746. [Google Scholar] [PubMed]
2. Hwang, B., Lee, J. H., Bang, D. (2018). Single-cell RNA sequencing technologies and bioinformatics pipelines. Experimental & Molecular Medicine, 50(8), 1–14. [Google Scholar]
3. Jovic, D., Liang, X., Zeng, H., Lin, L., Xu, F. et al. (2022). Single-cell RNA sequencing technologies and applications: A brief overview. Clinical and Translational Medicine, 12(3), e694. [Google Scholar] [PubMed]
4. Tang, X., Huang, Y., Lei, J., Luo, H., Zhu, X. (2019). The single-cell sequencing: New developments and medical applications. Cell & Bioscience, 9(1), 53. [Google Scholar]
5. Kyrochristos, I. D., Ziogas, D. E., Goussia, A., Glantzounis, G. K., Roukos, D. H. (2019). Bulk and single-cell next-generation sequencing: Individualizing treatment for colorectal cancer. Cancers, 11(11), 1809. [Google Scholar] [PubMed]
6. Rivello, F., van Buijtenen, E., Matuła, K., van Buggenum, J. A. G. L., Vink, P. et al. (2021). Single-cell intracellular epitope and transcript detection reveals signal transduction dynamics. Cell Reports Methods, 1(5), 100070. [Google Scholar] [PubMed]
7. Peterson, V. M., Zhang, K. X., Kumar, N., Wong, J., Li, L. et al. (2017). Multiplexed quantification of proteins and transcripts in single cells. Nature Biotechnology, 35(10), 936–939. [Google Scholar] [PubMed]
8. Yuan, M., Chen, L., Deng, M. (2022). Clustering CITE-seq data with a canonical correlation-based deep learning method. Frontiers in Genetics, 13, 977968. [Google Scholar] [PubMed]
9. Chen, C. W. (2022). Comment on “Long noncoding RNA UCA1 promotes glutamine-driven anaplerosis of bladder cancer by interacting with hnRNP I/L to upregulate GPT2 expression” by Chen et al. Translational Oncology, 18, 101372. [Google Scholar] [PubMed]
10. Patelli, G., Zeppellini, A., Spina, F., Righetti, E., Stabile, S. et al. (2022). The evolving panorama of HER2-targeted treatments in metastatic urothelial cancer: A systematic review and future perspectives. Cancer Treatment Reviews, 104, 102351. [Google Scholar] [PubMed]
11. Maiorano, B. A., de Giorgi, U., Ciardiello, D., Schinzari, G., Cisternino, A. et al. (2022). Immune-checkpoint inhibitors in advanced bladder cancer: Seize the day. Biomedicines, 10(2), 411. [Google Scholar] [PubMed]
12. Tran, L., Xiao, J. F., Agarwal, N., Duex, J. E., Theodorescu, D. (2021). Advances in bladder cancer biology and therapy. Nature Reviews. Cancer, 21(2), 104–121. [Google Scholar] [PubMed]
13. Yadollahvandmiandoab, R., Jalalizadeh, M., Buosi, K., Garcia-Perdomo, H. A., Reis, L. O. (2022). Immunogenic cell death role in urothelial cancer therapy. Current Oncology, 29(9), 6700–6713. [Google Scholar] [PubMed]
14. Arksey, H., O’Malley, L. (2005). Scoping studies: Towards a methodological framework. International Journal of Social Research Methodology, 8(1), 19–32. [Google Scholar]
15. Chapter 11: Scoping reviews (2020). JBI manual for evidence synthesis. JBI. https://jbi-global-wiki.refined.site/space/MANUAL/4687342/Chapter+11%3A+Scoping+reviews (accessed on 02/01/2024). [Google Scholar]
16. Moher, D., Liberati, A., Tetzlaff, J., Altman, D. G., PRISMA Group (2009). Preferred reporting items for systematic reviews and meta-analyses: The PRISMA statement. PLoS Medicine, 6(7), e1000097. [Google Scholar] [PubMed]
17. Tricco, A. C., Lillie, E., Zarin, W., O’Brien, K. K., Colquhoun, H. et al. (2018). Prisma extension for scoping reviews (Prisma-scrChecklist and explanation. Annals of Internal Medicine, 169(7), 467–473. [Google Scholar] [PubMed]
18. Gouin, K. H., Ing, N., Plummer, J. T., Rosser, C. J., Ben Cheikh, B. et al. (2021). An N-Cadherin 2 expressing epithelial cell subpopulation predicts response to surgery, chemotherapy and immunotherapy in bladder cancer. Nature Communications, 12(1), 4906. [Google Scholar] [PubMed]
19. Ma, Z., Li, X., Mao, Y., Wei, C., Huang, Z. et al. (2022). Interferon-dependent SLC14A1+ cancer-associated fibroblasts promote cancer stemness via WNT5A in bladder cancer. Cancer Cell, 40(12), 1550–1565.E7. [Google Scholar] [PubMed]
20. Lindskrog, S. V., Schmøkel, S. S., Nordentoft, I., Lamy, P., Knudsen, M. et al. (2023). Single-nucleus and spatially resolved intratumor subtype heterogeneity in bladder cancer. European Urology Open Science, 51, 78–88. [Google Scholar] [PubMed]
21. Fehlings, M., Kim, L., Guan, X., Yuen, K., Tafazzol, A. et al. (2022). Single-cell analysis reveals clonally expanded tumor-associated CD57+ CD8 T cells are enriched in the periphery of patients with metastatic urothelial cancer responding to PD-L1 blockade. Journal for Immunotherapy of Cancer, 10(8), e004759. [Google Scholar] [PubMed]
22. Wu, K., Zeng, J., Shi, X., Xie, J., Li, Y. et al. (2021). Targeting tigit inhibits bladder cancer metastasis through suppressing IL-32. Frontiers in Pharmacology, 12, 801493. [Google Scholar] [PubMed]
23. Xu, Y., Zeng, H., Jin, K., Liu, Z., Zhu, Y. et al. (2022). Immunosuppressive tumor-associated macrophages expressing interlukin-10 conferred poor prognosis and therapeutic vulnerability in patients with muscle-invasive bladder cancer. Journal for Immunotherapy of Cancer, 10(3), e003416. [Google Scholar] [PubMed]
24. Chen, Z., Zhou, L., Liu, L., Hou, Y., Xiong, M. et al. (2020). Single-cell RNA sequencing highlights the role of inflammatory cancer-associated fibroblasts in bladder urothelial carcinoma. Nature Communications, 11(1), 5077. [Google Scholar] [PubMed]
25. Oh, D. Y., Kwek, S. S., Raju, S. S., Li, T., McCarthy, E. et al. (2020). Intratumoral CD4+ T cells mediate anti-tumor cytotoxicity in human bladder cancer. Cell, 181(7), 1612–1625.E13. [Google Scholar] [PubMed]
26. Liang, Y., Tan, Y., Guan, B., Guo, B., Xia, M. et al. (2022). Single-cell atlases link macrophages and CD8+ T-cell subpopulations to disease progression and immunotherapy response in urothelial carcinoma. Theranostics, 12(18), 7745–7759. [Google Scholar] [PubMed]
27. Tao, Y., Li, X., Zhang, Y., He, L., Lu, Q. et al. (2022). TP53-related signature for predicting prognosis and tumor microenvironment characteristics in bladder cancer: A multi-omics study. Frontiers in Genetics, 13, 1057302. [Google Scholar] [PubMed]
28. You, C., Piao, X. M., Kang, K., Kim, Y. J., Kang, K. (2021). Integrative transcriptome profiling reveals ska3 as a novel prognostic marker in non-muscle invasive bladder cancer. Cancers, 13(18), 4673. [Google Scholar] [PubMed]
29. Wang, H., Mei, Y., Luo, C., Huang, Q., Wang, Z. et al. (2021). Single-cell analyses reveal mechanisms of cancer stem cell maintenance and epithelial-mesenchymal transition in recurrent bladder cancer. Clinical Cancer Research, 27(22), 6265–6278. [Google Scholar] [PubMed]
30. Lai, H., Cheng, X., Liu, Q., Luo, W., Liu, M. et al. (2021). Single-cell RNA sequencing reveals the epithelial cell heterogeneity and invasive subpopulation in human bladder cancer. International Journal of Cancer, 149(12), 2099–2115. [Google Scholar] [PubMed]
31. Li, Y., Xu, X., Song, L., Hou, Y., Li, Z. et al. (2012). Single-cell sequencing analysis characterizes common and cell-lineage-specific mutations in a muscle-invasive bladder cancer. GigaScience, 1(1), 12. [Google Scholar] [PubMed]
32. Banchereau, R., Chitre, A. S., Scherl, A., Wu, T. D., Patil, N. S. et al. (2021). Intratumoral CD103+ CD8+ T cells predict response to PD-L1 blockade. Journal for Immunotherapy of Cancer, 9(4), e002231. [Google Scholar] [PubMed]
33. Yang, Z., Li, C., Fan, Z., Liu, H., Zhang, X. et al. (2017). Single-cell sequencing reveals variants in ARID1A, GPRC5A and MLL2 driving self-renewal of human bladder cancer stem cells. European Urology, 71(1), 8–12. [Google Scholar] [PubMed]
34. Luo, Y., Tao, T., Tao, R., Huang, G., Wu, S. (2022). Single-cell transcriptome comparison of bladder cancer reveals its ecosystem. Frontiers in Oncology, 12, 818147. [Google Scholar] [PubMed]
35. Warrick, J. I., Hu, W., Yamashita, H., Walter, V., Shuman, L. et al. (2022). Author Correction: FOXA1 repression drives lineage plasticity and immune heterogeneity in bladder cancers with squamous differentiation. Nature Communications, 13(1), 6575. [Google Scholar] [PubMed]
36. Zhang, X., Zhang, M., Hou, Y., Xu, L., Li, W. et al. (2016). Single-cell analyses of transcriptional heterogeneity in squamous cell carcinoma of urinary bladder. Oncotarget, 7(40), 66069–66076. [Google Scholar] [PubMed]
37. Tanaka, N., Katayama, S., Reddy, A., Nishimura, K., Niwa, N. et al. (2018). Single-cell RNA-seq analysis reveals the platinum resistance gene COX7B and the surrogate marker CD63. Cancer Medicine, 7(12), 6193–6204. [Google Scholar] [PubMed]
38. Sfakianos, J. P., Daza, J., Hu, Y., Anastos, H., Bryant, G. et al. (2020). Epithelial plasticity can generate multi-lineage phenotypes in human and murine bladder cancers. Nature Communications, 11(1), 2540. [Google Scholar] [PubMed]
39. Kerzeli, I. K., Lord, M., Doroszko, M., Elgendy, R., Chourlia, A. et al. (2021). Single-cell RNAseq and longitudinal proteomic analysis of a novel semi-spontaneous urothelial cancer model reveals tumor cell heterogeneity and pretumoral urine protein alterations. PLoS One, 16(7), e0253178. [Google Scholar] [PubMed]
40. Esteso, G., Felgueres, M. J., García-Jiménez, Á.F., Reyburn-Valés, C., Benguría, A. et al. (2023). BCG-activation of leukocytes is sufficient for the generation of donor-independent innate anti-tumor NK and γδ T-cells that can be further expanded in vitro. Oncoimmunology, 12(1), 2160094. [Google Scholar] [PubMed]
41. Podojil, J. R., Glaser, A. P., Baker, D., Courtois, E. T., Fantini, D. et al. (2020). Antibody targeting of B7-H4 enhances the immune response in urothelial carcinoma. Oncoimmunology, 9(1), 1744897. [Google Scholar] [PubMed]
42. Zhou, R., Zhou, J., Muhuitijiang, B., Tan, W. (2022). Construction and experimental validation of a B cell senescence-related gene signature to evaluate prognosis and immunotherapeutic sensitivity in bladder cancer. Functional & Integrative Genomics, 23(1), 3. [Google Scholar]
43. Liu, L., Hou, Y., Deng, C., Tao, Z., Chen, Z. et al. (2022). Single cell sequencing reveals that CD39 inhibition mediates changes to the tumor microenvironment. Nature Communications, 13(1), 6740. [Google Scholar] [PubMed]
44. Chen, H., Yang, W., Xue, X., Li, Y., Jin, Z. et al. (2022). Integrated analysis revealed an inflammatory cancer-associated fibroblast-based subtypes with promising implications in predicting the prognosis and immunotherapeutic response of bladder cancer patients. International Journal of Molecular Sciences, 23(24), 15970. [Google Scholar] [PubMed]
45. Zhao, Y., Zhao, B., Yan, W. H., Xia, Y., Wang, Z. H. et al. (2021). Integrative analysis identified MCT4 as an independent prognostic factor for bladder cancer. Frontiers in Oncology, 11, 704857. [Google Scholar] [PubMed]
46. Du, Y., Miao, W., Jiang, X., Cao, J., Wang, B. et al. (2021). The epithelial to mesenchymal transition related gene calumenin is an adverse prognostic factor of bladder cancer correlated with tumor microenvironment remodeling, gene mutation, and ferroptosis. Frontiers in Oncology, 11, 683951. [Google Scholar] [PubMed]
47. Chen, H., Chen, G. (2022). Dissecting immunosuppressive cell communication patterns reveals junb proto-oncogene (Junb) shaping a non-inflamed tumor microenvironment. Frontiers in Genetics, 13, 883583. [Google Scholar] [PubMed]
48. Xiang, X., Guo, Y., Chen, Z., Zhang, F., Huang, J. et al. (2022). A prognostic risk prediction model based on ferroptosis-related long non-coding RNAs in bladder cancer: A bulk RNA-seq research and scRNA-seq validation. Medicine, 101(51), e32558. [Google Scholar] [PubMed]
49. Wang, L., Sfakianos, J. P., Beaumont, K. G., Akturk, G., Horowitz, A. et al. (2021). Myeloid cell-associated resistance to pd-1/pd-l1 blockade in urothelial cancer revealed through bulk and single-cell rna sequencing. Clinical Cancer Research, 27(15), 4287–4300. [Google Scholar] [PubMed]
50. Li, J. D., Farah, A. A., Huang, Z. G., Zhai, G. Q., Wang, R. G. et al. (2022). Clinical significance and potential regulatory mechanism of overexpression of pituitary tumor-transforming gene transcription factor in bladder cancer. BMC Cancer, 22(1), 713. [Google Scholar] [PubMed]
51. Jiang, Y., Qu, X., Zhang, M., Zhang, L., Yang, T. et al. (2022). Identification of a six-gene prognostic signature for bladder cancer associated macrophage. Frontiers in Immunology, 13, 930352. [Google Scholar] [PubMed]
52. Yao, J., Liu, Y., Yang, J., Li, M., Li, S. et al. (2022). Single-cell sequencing reveals that DBI is the key gene and potential therapeutic target in quiescent bladder cancer stem cells. Frontiers in Genetics, 13, 904536. [Google Scholar] [PubMed]
53. Yu, L., Hu, R., Peng, G., Ding, Q., Tao, T. et al. (2022). Prognostic significance of lineage diversity in bladder cancer revealed by single-cell sequencing. Frontiers in Genetics, 13, 862634. [Google Scholar] [PubMed]
54. Acharjee, A., Agarwal, P., Nash, K., Bano, S., Rahman, T. et al. (2021). Immune infiltration and prognostic and diagnostic use of LGALS4 in colon adenocarcinoma and bladder urothelial carcinoma. American Journal of Translational Research, 13(10), 11353–11363. [Google Scholar] [PubMed]
55. Das, S., Camphausen, K., Shankavaram, U. (2020). Cancer-specific immune prognostic signature in solid tumors and its relation to immune checkpoint therapies. Cancers, 12(9), 2476. [Google Scholar] [PubMed]
56. Hu, R., Tao, T., Yu, L., Ding, Q., Zhu, G. et al. (2021). Multi-omics characterization of tumor microenvironment heterogeneity and immunotherapy resistance through cell states-based subtyping in bladder cancer. Frontiers in Cell and Developmental Biology, 9, 809588. [Google Scholar] [PubMed]
57. Wang, Z., Chen, J., Yang, L., Cao, M., Yu, Y. et al. (2020). Single-cell sequencing-enabled hexokinase 2 assay for noninvasive bladder cancer diagnosis and screening by detecting rare malignant cells in urine. Analytical Chemistry, 92(24), 16284–16292. [Google Scholar] [PubMed]
58. Chen, A., Fu, G., Xu, Z., Sun, Y., Chen, X. et al. (2018). Detection of urothelial bladder carcinoma via microfluidic immunoassay and single-cell DNA copy-number alteration analysis of captured urinary-exfoliated tumor cells. Cancer Research, 78(14), 4073–4085. [Google Scholar] [PubMed]
59. Edgar, R., Domrachev, M., Lash, A. E. (2002). Gene expression omnibus: NCBI gene expression and hybridization array data repository. Nucleic Acids Research, 30(1), 207–210. [Google Scholar] [PubMed]
60. https://www.cancer.gov/tcga (accessed on 02/01/2024). [Google Scholar]
61. Sarkans, U., Gostev, M., Athar, A., Behrangi, E., Melnichuk, O. et al. (2018). The BioStudies database-one stop shop for all data supporting a life sciences study. Nucleic Acids Research, 46(D1), D1266–D1270. [Google Scholar] [PubMed]
62. www.gtexportal.org (accessed on 02/01/2024). [Google Scholar]
63. Sun, D., Wang, J., Han, Y., Dong, X., Ge, J. et al. (2021). TISCH: A comprehensive web resource enabling interactive single-cell transcriptome visualization of tumor microenvironment. Nucleic Acids Research, 49(D1), D1420–D1430. [Google Scholar] [PubMed]
64. Yuan, H., Yan, M., Zhang, G., Liu, W., Deng, C. et al. (2019). CancerSEA: A cancer single-cell state atlas. Nucleic Acids Research, 47(D1), D900–D908. [Google Scholar] [PubMed]
65. www.cell.com/pb-assets/consortium/pancanceratlas/pancani3/index.html (accessed on 02/01/2024). [Google Scholar]
66. Minoli, M., Kiener, M., Thalmann, G. N., Kruithof-de Julio, M., Seiler, R. (2020). Evolution of urothelial bladder cancer in the context of molecular classifications. International Journal of Molecular Sciences, 21(16), 5670. [Google Scholar] [PubMed]
67. Altman, N., Krzywinski, M. (2018). The curse(s) of dimensionality. Nature Methods, 15(6), 399–400. [Google Scholar] [PubMed]
68. Hong, R., Koga, Y., Bandyadka, S., Leshchyk, A., Wang, Y. et al. (2022). Comprehensive generation, visualization, and reporting of quality control metrics for single-cell RNA sequencing data. Nature Communications, 13(1), 1688. [Google Scholar] [PubMed]
69. Wu, Y., Tamayo, P., Zhang, K. (2018). Visualizing and interpreting single-cell gene expression datasets with similarity weighted nonnegative embedding. Cell Systems, 7(6), 656–666.E4. [Google Scholar] [PubMed]
70. Kharchenko, P. V. (2021). The triumphs and limitations of computational methods for scRNA-seq. Nature Methods, 18(7), 723–732. [Google Scholar] [PubMed]
71. Becht, E., McInnes, L., Healy, J., Dutertre, C. A., Kwok, I. W. H. et al. (2018). Dimensionality reduction for visualizing single-cell data using UMAP. Nature Biotechnology. [Google Scholar]
72. Wang, C., Liu, H. (2022). Factors influencing degradation kinetics of mRNAs and half-lives of microRNAs, circRNAs, lncRNAs in blood in vitro using quantitative PCR. Scientific Reports, 12(1), 7259. [Google Scholar] [PubMed]
73. Pai, A. A., Luca, F. (2019). Environmental influences on RNA processing: Biochemical, molecular and genetic regulators of cellular response. Wiley Interdisciplinary Reviews. RNA, 10(1), e1503. [Google Scholar] [PubMed]
74. Lobo, I. (2008). Environmental influences on gene expression. Nature Education, 1(1), 39. [Google Scholar]
75. Rijo-Ferreira, F., Takahashi, J. S. (2019). Genomics of circadian rhythms in health and disease. Genome Medicine, 11(1), 82. [Google Scholar] [PubMed]
76. Beato, M., Truss, M., Chávez, S. (1996). Control of transcription by steroid hormones. Annals of the New York Academy of Sciences, 784, 93–123. [Google Scholar] [PubMed]
Cite This Article
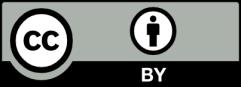