Open Access
ARTICLE
Pan-cancer analysis of RNA 5-methylcytosine reader (ALYREF)
1 Samuel Oschin Comprehensive Cancer Institute, Department of Medicine, Department of Biomedical Sciences, Cedars-Sinai Medical Center, Los Angeles, CA, 90048, USA
2 Department of Urology, The Second Affiliated Hospital of Anhui Medical University, Hefei, 230601, China
3 Department of Urology, Institute of Urology, West China Hospital, Sichuan University, Chengdu, 610041, China
4 Department of Pathology, Ningbo Diagnostic Pathology Center, Ningbo, 315021, China
5 Department of Public Research Platform, Taizhou Hospital of Zhejiang Province Affiliated to Wenzhou Medical University, Linhai, 317000, China
6 Department of Rehabilitation, West Kyushu University, Kanzaki-shi, 842-8585, Japan
7 Department of Urology, Kyung Hee University, Seoul, 446 701, South Korea
8 Department of Rehabilitation, The Affiliated Hospital of Southwest Medical University, Luzhou, 646000, China
* Corresponding Authors: DENGXIONG LI. Email: ; DECHAO FENG. Email:
,
# These authors contributed equally to this work
Oncology Research 2024, 32(3), 503-515. https://doi.org/10.32604/or.2024.045050
Received 16 August 2023; Accepted 01 December 2023; Issue published 06 February 2024
Abstract
The increasing interest in RNA modifications has significantly advanced epigenomic and epitranscriptomic technologies. This study focuses on the immuno-oncological impact of ALYREF in human cancer through a pan-cancer analysis, enhancing understanding of this gene’s role in cancer. We observed differential ALYREF expression between tumor and normal samples, correlating strongly with prognosis in various cancers, particularly kidney renal papillary cell carcinoma (KIRP) and liver hepatocellular carcinoma (LIHC). ALYREF showed a negative correlation with most tumor-infiltrating cells in lung squamous cell carcinoma (LUSC) and lymphoid neoplasm diffuse large B-cell lymphoma (DLBC), while positive correlations were noted in LIHC, kidney chromophobe (KICH), mesothelioma (MESO), KIRP, pheochromocytoma and paraganglioma (PARD), and glioma (GBMLGG). Additionally, ALYREF expression was closely associated with tumor heterogeneity, stemness indices, and a high mutation rate in TP53 across these cancers. In conclusion, ALYREF may serve as an oncogenic biomarker in numerous cancers, meriting further research attention.Keywords
The core tenet of the central dogma in molecular biology, a landmark achievement of the 20th century, posits that genetic information can be exchanged between nucleic acids and proteins, two distinct classes of biological macromolecules [1]. This concept encompasses transcription and translation processes, where genetic information transfers from DNA to RNA and from RNA to proteins, and includes DNA replication. Additionally, RNA self-replication in some viruses (e.g., Tobacco Mosaic Virus) and reverse transcription of RNA into DNA in others (like certain oncogenic viruses) complement this rule [1–3]. In these processes, epigenomics plays a pivotal role in various cellular physiology processes in eukaryotes. It involves a biodiverse assembly of covalent modifications to histone proteins and nucleic acids, changing nucleosomes’ spatiotemporal arrangement, regulating chromatin’s three-dimensional conformation and nuclear topology, RNA splicing mechanisms, RNA binding protein location and activity, and transcribed elements of the non-protein coding genome. These elements work in concert to dynamically regulate chromatin structure and fine-tune gene expression, influencing biological properties [4]. Consequently, epigenome disruption can lead to the onset and progression of cancer through disordered transcriptional programs [5,6].
The growing understanding of DNA and RNA modifications’ biological functions has spurred advancements in epigenomic and epitranscriptomic technologies, revealing more than 17 and 160 different types of chemical modifications in DNA and RNA, respectively [7]. Epigenetic modifications involve the attachment, removal, and recognition of several chemical groups through specialized enzymes known as epigenetic “writers”, “erasers”, and “readers” [4,8,9]. While modifications in histones and DNA have been extensively investigated, covalent RNA modifications have primarily focused on the 5’ cap modification and the poly (A) tail, even though over one hundred types of chemical modifications have been identified in cellular RNAs since 1960s [10], notably following the sequencing of the first biological RNA in 1965 [11]. In recent decades, internal RNA modifications have gained attention for their versatile roles in cell fate, given the increasing awareness of RNA’s direct functional impact on gene expression through various classes of non-coding RNAs, such as microRNA and long ncRNA [12]. Among these, N6-methyladenosine is notable for its significant effects on normal life activities and diseases [13], serving as a promising biomarker and therapeutic targets [14–16]. Its functions include accelerate pre-mRNA processing, mRNA stability, splicing, nuclear transport, and translational ability [17–19]. Other RNA modifications include 5-methoxycarbonylmethyluridine, N6-methyladenosine, 5-methylcytosine (m5C), 7-methylguanosine, 5-methoxycarbonylmethyl-2-thiouridine, pseudouridine, N1-methyladenosine, and others [12].
The roles of m5C readers (YTHDF2, ALYREF, and YBX1) and writers (DNMTs and NSUNs), implicated in cellular metabolism and motility, are thought to regulate gene expression at the post-transcriptional stage [20]. This study aims to elucidate the immuno-oncological effect of ALYREF in human cancer through a comprehensive pan-cancer analysis, thereby deepening our understanding of this gene’s role in cancer.
Differential and prognostic analysis
Consistent with our previous studies [21,22], we extracted ALYREF (ENSG00000183684) expression data from a standardized TCGA pan-cancer dataset obtained from the UCSC database [23]. We also evaluated metastatic samples from primary tumor, TCGA-Skin Cutaneous Melanoma, and cancer-derived peripheral blood from primary blood (TCGA-Acute Myeloid Leukemia). A high-quality TCGA prognostic dataset was acquired from prior investigations [24]. After excluding samples with expression levels of 0 and those with follow-up periods shorter than 30 days, we logarithmically transformed each expression value log2 (x+1). Consequently, we obtained expression data for 38 malignancies and corresponding survival information (overall, disease-specific, disease-free, and progression-free survival), excluding cancers with fewer than 10 samples. ALYREF’s clinical associations with pancreatic cancer were also evaluated. Using the Cox proportional hazards regression model and the log-rank test, we analyzed ALYREF’s prognostic significance. We compared differential expression between tumor and normal samples by screening samples from solid tissue normal, cancer-peripheral blood, and primary tumors, and eliminating those with fewer than three samples. Differential significance was determined using signed rank tests and unpaired Wilcoxon rank sum tests.
Tumor stemness, heterogeneity, and mutation landscape
We investigated the correlation between ALYREF expression and tumor stemness using the Spearman analysis method with two stemness indexes: epigenetically regulated RNA expression-based (EREG-EXPss) and RNA expression-based (RNAss) [25]. In addition, neoantigen (NEO) [26], tumor purity [26], and tumor mutation burden (TMB) obtained from the Genomic Data Commons (https://portal.gdc.cancer.gov/) and proceeded by MuTect2 software and R package “maftools” [27], and microsatellite instability (MSI) [28] were used to assess the relationship between tumor heterogeneity and ALYREF expression. We combined the information on mutations and gene expression, then sorted the samples of synonymous mutations. The frequency of gene mutations in high and low ALYREF expression groups was compared using the chi-square test, based on ALYREF’s median expression in each analyzed malignancy.
Physical interactions and tumor immune microenvironment (TME)
We employed GeneMANIA to investigate potential physical interaction genes with ALYREF [29]. Additionally, we analyzed the associations of ALYREF mRNA expression with 24 inhibitory, 36 stimulatory checkpoints [26], and 150 immunoregulatory genes (chemokine, receptor, MHC, immunoinhibitory, immunostimulatory). The TME was assessed [30] using the Timer algorithm and the R package “IOBR” [31].
Given ALYREF’s notable association with urinary tumors, we examined its biological function in renal and prostate cancer cell lines. The CAKI-2 cell line, sourced from the National Collection of Authenticated Cell Cultures, was cultivated in matched complete medium. Our previous studies detail the cell culture procedures, real-time quantitative polymerase chain reaction (RT-qPCR), and cell counting kit-8 (CCK8) tests [32–34]. The primer sequences used were as follows, with glyceraldehyde-3-phosphate dehydrogenase (GAPDH) serving as an internal control: GAPDH: 5′-CTGGGCTACACTGAGCACC-3′ (forward) and 5′-TCCAAGTGGTCGTTGAGGGCAATG-3′ (reverse); ALYREF: 5′-TATGATCGCTCTGGTCGCAG-3′ (forward) and 5′-AGAGGGACGCCGTTGTACT-3′ (reverse). Additionally, the sequences of small interfering RNA (siRNA) of ALYREF were as follows: ALYREF si-1 sense: 5′-CGUGGAGACAGGUGGGAAAdTdT-3′; ALYREF si-1 antisense: 5′-UUUCCCACCUGUCUCCACGdTdT-3′. ALYREF si-2 sense: 5′-GGAGUCUCAGACGCCGAUAUUdTdT-3′; ALYREF si-2 antisense: 5′-AAUAUCGGCGUCUGAGACUCCdTdT-3′; ALYREF si-3 sense: 5′-GAACUCUUUGCUGAAUUUGGAdTdT-3′; ALYREF si-3 antisense: 5′-UCCAAAUUCAGCAAAGAGUUCdTdT-3′. Control sense: 5′-UUCUCCGAACGUGUCACGUdTdT-3′; Control antisense: 5′-ACGUGACACGUUCGGAGAAdTdT-3′.
All analyses were conducted using software R (version 3.6.3) and its relevant packages. Unpaired Wilcoxon rank sum and signed rank tests assessed pairwise differences, while the Kruskal test was used for multiple sample sets. Spearman analysis evaluated correlations among continuous variables that failed the Shapiro‒Wilk normality test. Statistical significance was defined as a two-sided p < 0.05, with significance levels indicated as follows: *p < 0.05; **p < 0.01; ***p < 0.001.
Differential expression and prognosis analysis
ALYREF exhibited significant differential expression in various human cancers compared to normal samples, with heightened expression in nineteen cancers and reduced expression in two (Fig. 1a). This gene was also closely associated with key prognostic indices including overall survival (Fig. 1b), disease-specific survival (Fig. 1c), disease-free survival (Fig. 1d), and progression-free survival (Fig. 1e) in numerous cancers. These included a majority of urinary tumors such as kidney chromophobe (KICH), pan-kidney cohort (KIPAN), kidney renal papillary cell carcinoma (KIRP), bladder urothelial carcinoma (BLCA), prostate adenocarcinoma (PRAD) and adrenocortical carcinoma (ACC)), glioma (GBMLGG), brain lower grade glioma (LGG), esophageal carcinoma (ESCA), lung squamous cell carcinoma (LUSC), cholangiocarcinoma (CHOL), mesothelioma (MESO), lymphoid neoplasm diffuse large B-cell lymphoma (DLBC), lung adenocarcinoma (LUAD), and liver hepatocellular carcinoma (LIHC). Notably, ALYREF’s relationship with KIRP and LIHC was statistically significant across all these prognostic indices (Fig. 2a). Furthermore, ALYREF mRNA expression showed significant age associations in six of these cancers (Fig. 2b), with both positive and negative correlations (Suppl. Fig. S1).
Figure 1: Differential expression and prognosis analyses at pan-cancer level. (a) ALYREF mRNA expression differences between tumor and normal samples with statistic significance at pan-cancer level; (b) forest plot showing the prognostic value of ALYREF for overall survival; (c) forest plot showing the prognostic value of ALYREF for disease-specific survival; (d) forest plot showing the prognostic value of ALYREF for disease-free survival; (e) forest plot showing the prognostic value of ALYREF for progression-free survival. **p < 0.01; ***p < 0.001; ****p < 0.0001.
Figure 2: Clinical correlation and immune analyses. (a) Venn diagram showing the results of differential expression and various prognostic indexes; (b) the lollipop graph showing the correlation between ALYREF expression and age at pan-cancer level; (c) the physically interacted genes with ALYREF; (d) heat map showing the correlations between immunomodulatory genes and ALYREF at pan-cancer level; (e) heat map showing the correlations between immune checkpoints and ALYREF at pan-cancer level; (f) heat map showing the correlations between tumor-infiltrating cells and ALYREF at pan-cancer level. *p < 0.05; **p < 0.01; ***p < 0.001; ****p < 0.0001.
Physical interactions and immune analysis
Twenty genes reported to interact physically with ALYREF were identified (Fig. 2c). ALYREF was positively correlated with most immunomodulatory genes in patients with urinary tumors (KICH, KIRP, KIPAN, PRAD, BLCA, and ACC), GBMLGG, LGG, MESO, and LIHC, showing particularly strong correlations with KICH and ACC (Fig. 2d). Similarly, ALYREF was positively associated with numerous immune checkpoints in these cancers, except for DLBC, LUSC, and ESCA (Fig. 2e). Notably, ALYREF demonstrated a significant correlation with CD276, an immunoglobulin superfamily member involved in regulating T-cell-mediated immune responses, in patients with urinary tumors (KICH, KIRP, KIPAN, PRAD, BLCA, and ACC), LIHC, GBMLGG, and LGG, especially in ACC, KIPAN, KIRP, PRAD, LIHC, GBMLGG, and LGG (r ≥ 0.5) (Fig. 2e). Consistent with these findings, ALYREF was negatively related to most tumor-infiltrating cells in LUSC and DLBC, while showing opposite correlations in LIHC, KICH, MESO, KIRP, PARD, and GBMLGG (Fig. 2f). Remarkably, KICH displayed notable correlations with B cells, CD8+ T cells, macrophages, and dendritic cells, and MESO exhibited a high correlation with dendritic cells (Fig. 2f).
Tumor heterogeneity and stemness
We discovered significant correlations between ALYREF expression and various aspects of tumor heterogeneity and stemness. For TMB, ALYREF was significantly associated with GBMLGG (r = 0.41), LGG (r = 0.29), LUAD (r = 0.41), KIPAN (r = 0.18), BLCA (r = 0.16), ACC (r = 0.42) and ESCA (r = −0.17) (Fig. 3a). In terms of MSI, notable correlations were found with LUSC (r = 0.20), LIHC (r = 0.10), and GBMLGG (r = −0.27) (Fig. 3b). For NEO, ALYREF showed a significant relationship with LUAD (r = 0.26) (Fig. 3c). Regarding tumor purity, ALYREF was markedly associated with GBMLGG (r = 0.44), LGG (r = 0.42), ESCA (r = 0.36), LUSC (r = 0.41) and BLCA (r = −0.17) (Fig. 3d). In the context of tumor stemness, ALYREF was significantly correlated with LGG (r = 0.15), LUAD (r = 0.49), ESCA (r = 0.48), PRAD (r = 0.10), LUSC (r = 0.56), LIHC (r = 0.37), MESO (r = 0.31), BLCA (r = 0.37), ACC (r = 0.24), and DLBC (r = 0.57) for RNAss (Fig. 3e). For EREG.EXPss, significant correlations were noted with GBMLGG (r = 0.18), LGG (r = 0.17), ESCA (r = 0.22), PRAD (r = 0.19), LUSC (r = 0.26), BLCA (r = 0.17), ACC (r = 0.45), DLBC (r = 0.57), KIRP (r = −0.14), and KIPAN (r = −0.12) (Fig. 3f).
Figure 3: Tumor heterogeneity and stemness analyses. (a) The lollipop graph showing the correlation between ALYREF expression and TMB at pan-cancer level; (b) the lollipop graph showing the correlation between ALYREF expression and MSI at pan-cancer level; (c) the lollipop graph showing the correlation between ALYREF expression and NEO at pan-cancer level; (d) the lollipop graph showing the correlation between ALYREF expression and tumor purity at pan-cancer level; (e) the lollipop graph showing the correlation between ALYREF expression and RNAss at pan-cancer level; (f) the lollipop graph showing the correlation between ALYREF expression and EREG.EXPss at pan-cancer level.
Given ALYREF’s prominent role in urinary tumors and LIHC, we analyzed their mutation landscapes (Fig. 4). TP53 emerged as the most mutated gene in these cancers (Figs. 4a and 4b, 4d–4f). Notable mutations included CTNNB and TTN in ACC (Fig. 4a), MET and RERE in KIRP (Fig. 4c), TP53, RB1, FGFR3, FAT3, and AHNAK in BLCA (Fig. 4d), TP53, FAT4, and CUBN in PRAD (Fig. 4e), TP53, CSMD3, RB1, CNGA3 and RTPRB in LIHC (Fig. 4f), showing significant variation between high- and low-expression ALYREF groups. These findings underscore the importance of TP53 in these cancers.
Figure 4: Mutation analysis. (a) Waterfall plot showing the gene mutation frequency differences between high-and low-ALYREF expression groups in ACC; (b) waterfall plot showing the gene mutation frequency differences between high-and low-ALYREF expression groups in KICH; (c) waterfall plot showing the gene mutation frequency differences between high- and low-ALYREF expression groups in KIRP; (d) waterfall plot showing the gene mutation frequency differences between high- and low-ALYREF expression groups in BLCA; (e) waterfall plot showing the gene mutation frequency differences between high-and low-ALYREF expression groups in PRAD; (f) waterfall plot showing the gene mutation frequency differences between high-and low-ALYREF expression groups in LIHC. Note: group was determined by the median value of ALYREF expression.
Using the RT-qPCR assay, we observed that ALYREF siRNA1, siRNA2, and siRNA3 effectively reduced ALYREF mRNA expression in PC3 cells (Fig. 5a). Based on these results, siRNA1 and siRNA2 were selected for subsequent experiments. We found that these siRNAs significantly inhibited the proliferation of 786-O, ACHN, CAKI-2, PC3 and DU145 cells (Figs. 5b–5f).
Figure 5: Effect of ALYREF on the biological behaviors of renal cancer and prostate cancer cell lines. (a) RT‒qPCR results of ALYREF siRNAs; (b) Effect of ALYREF siRNAs on 786-O cells using CCK8 assay; (c) Effect of ALYREF siRNAs on ACHN cells using CCK8 assay; (d) Effect of ALYREF siRNAs on CAKI-2 cells using CCK8 assay; (e) Effect of ALYREF siRNAs on PC3 cells using CCK8 assay; (f) Effect of ALYREF siRNAs on DU145 cells using CCK8 assay. *p < 0.05; **p < 0.01; ***p < 0.001; ****p < 0.0001.
The epigenome plays a crucial role in a wide array of cellular processes and is vital for the survival of living organisms. Recent studies have highlighted the use of epigenetic markers, like histone acetylation and methylation marks on cell-free nucleosomes, as proxies for RNA-based transcriptional profiling [35]. The development, progression, and metastasis of tumors are closely linked to aberrant epigenomes, which encompass DNA methylation, histone modification, nucleosome remodeling, and RNA level changes [36]. Over recent decades, significant progress has been made in understanding the functional importance of RNA modifications in regulating the processing and function of both coding and non-coding RNAs, thereby shaping diverse gene expression programs.
The identification of DNA m5C dates back to the 1950s, and it was later recognized that m5C modification, a common alteration in various RNA species, occurs when a methyl group from the donor molecule, typically S-adenosyl-methionine, attaches to thefifth carbon position of the cytosine base in RNA [37,38]. In recent years, it has become evident that m5C RNA modification plays a critical role in controlling how both coding and non-coding RNAs regulate RNA metabolism and function. There is increasing evidence that m5C influences RNA stability, translation, nuclear export, and cleavage, thereby affecting cell division, differentiation, apoptosis, stress responses, and other biological processes [6,39]. While m5C is found in a broad range of RNAs, it is most common in eukaryotic tRNAs and rRNAs and is typically detected using RNA bisulfite sequencing, which yields efficient and abundant results [40–43]. Other detection methods include RNA methyltransferases crosslinked to RNA targets and m5C antibody-based immunoprecipitation techniques [40]. However, methods for identifying m5C sites in RNAs other than tRNA and rRNA are less reliable, underscoring the urgent need for new computational methods and improved bisulfite sequencing techniques [44,45].
In vitro and in vivo studies have identifiedthe RNA methyltransferase NSUN2 as primarily enzyme catalyzing m5C production in mRNAs, with m5C specifically recognized by the mRNA export adaptor ALYREF [46]. Recent research has linked ALYREF to the development of various tumors, including bladder cancer [47,48], breast cancer [49], neuroblastoma [50], hepatocellular carcinoma [51,52], glioblastoma [53], and non-small cell lung cancer [54]. These findings underscore the gene’s critical role in human cancer. Beyond these experimentally validated cancers, our study observed differential ALYREF expression in most cancers and its prognostic potential in KICH, KIRP, PRAD, ACC, ESCA, CHOL, MESO, and DLBC, particularly in urinary tumors. Additionally, ALYREF inhibition significantly impaired renal and prostate cancer cell proliferation in vitro. Recent studies indicate that aging is accompanied by progressive epigenetic alterations in both dividing and nondividing cells [55], with epigenetic changes proposed as a hallmark of aging by 2023 [56]. Aging is a risk factor for various age-related diseases, including cancers [57–60] and degenerative diseases [61–64]. Our study found that ALYREF expression was significantly related to aging, confirming the link between epigenomics, aging, and cancer. We also identified several ALYREF interaction genes for future cancer research.
In this study, correlation analyses between ALYREF expression and immunomodulatory genes, immune checkpoints, and tumor-infiltrating cells showed consistent results. We observed a significant positive correlation between ALYREF and most tumor-infiltrating cells in urinary tumors, LIHC, GBMLGG and LGG. Notably, ALYREF also correlated positively with CD276. During immune response, T cell activation depends on two signals: the interaction between the T cell receptor and the peptide-major histocompatibility complex on antigen-presenting cells, and the engagement of CD28 receptor family members on T cells with B7 ligand family members on antigen-presenting cells [65–68]. This costimulatory signaling is crucial for both helper and cytotoxic T cells, enhancing their activation, proliferation, and differentiation, and leading to cytokine reception [65–67]. CD276, also known as B7-H3, is a type I transmembrane protein that belongs to the second signal pathway in immune responses [69–71]. Although broadly expressed in various tissues (such as liver and prostate) and cell types at the mRNA level, its protein expression is limited due to post-transcriptional regulation [66]. CD276 is believed to be involved in regulating the T-cell-mediated immune response and may play a protective role in tumor cells by inhibiting natural-killer mediated cell lysis [72]. Research indicates that despite the widespread mRNA expression of this gene in both normal tissues and solid tumors, the protein predominantly occurs in tumor tissues. This expression correlates with negative prognosis and poor clinical outcomes in patients [66,72]. Consequently, we hypothesize that ALYREF may increase CD276 protein levels in antigen-presenting cells, thereby restricting the function of CD8+ T cells. However, ALYREF mRNA expression shows a negative correlation with tumor-infiltrating cells in LUSC. Given that this gene is upregulated in most cancers, including LUSC, we suggest that ALYREF could contribute to the formation of immunodeficiency niches by reducing the number of tumor-infiltrating cells, thus exacerbating LUSC progression. Estimating tumor purity using high-throughput genomic and epigenomic data presents an alternative to cell sorting technologies, such as Fluorescent-Activated Cell Sorting or Magnetic-Activated Cell Sorting. This approach is advantageous for assessing the proportion of cancer cells in solid tumor samples due to the time-consuming and costly nature of experimental validation [73–75]. In addition to fewer immune cells, LUSC patients with high ALYREF expression tend to exhibit greater tumor purity, which suggests a propensity for worse outcomes. Although no significant correlation was found between ALRREF expression and tumor-infiltrating cells in patients with GBMLGG, LGG, and ESCA, patients with high expression of this gene often have higher tumor purity and are likely to experience poorer prognoses. NEO, produced by cancer cell mutations, possesses strong immunogenicity and is exclusively expressed in tumor cells, making it an attractive therapeutic target [76].
Moreover, TMB defined by the number of somatic mutations, including synonymous and intron mutations, per 38 Mb of genome area for targeted sequencing, has shown that higher TMB correlates with improved survival in bladder cancer patients receiving immune checkpoint inhibitor treatments [77–80]. LUAD, representing almost 40% of lung cancer cases, is a prevalent subtype and is noted for its high morbidity and mortality rates globally [37,81]. In China, lung cancer has been the most rapidly increasing malignancy over the past 30 years, predominantly comprising histological subtypes such as adenocarcinoma and squamous cell carcinoma, which make up 80%–85% of cases, with the remainder being small cell lung cancer (atezolizumab plus etoposide/platinum) [58,82]. In our study, a positive association was observed between ALYREF and both NEO and TMB in LUAD, suggesting that patients with high ALYREF expression might respond better to immunotherapy. Additionally, GBMLGGs, highly aggressive cancers originating in the neuroepithelial layer, and ACC, despite its low incidence, often lead to immediate metastases, precluding surgical interventions [83–85]. Patients with overexpressed ALYREF in these cancers might also benefit from immunotherapy. As cancer progresses, a differentiated phenotype is lost, and cancer cells acquire progenitor- and stem-cell-like characteristics, indicating that undifferentiated primary tumors are more prone to metastasis and associated with worse prognosis [25]. In Hodgkin’s lymphoma series, including DLBC, the incidence ranges from 30% to 58%, and the recommended radiotherapy dose post-chemotherapy is 30 to 46 Gy; yet, over 30% of DLBC patients relapse [86,87]. A clear positive correlation was found between ALYREF expression and both RNAss and EREG.EXPss in DLBC patients, hinting at a potentially poorer prognosis for those with higher ALYREF expression.
TP53, the most frequently mutated gene in human cancers, involves multiple pathways for tumor growth inhibition [88–91]. However, TP53 mutations often lead to p53 inactivation, enabling tumor cells to evade death and grow rapidly [89,92,93]. Originally identified as a key regulator in acute DNA damage response, recent studies have uncovered additional TP53 downstream targets and pathways [92–95], including those related to stemness, metabolism, redox biology, genomic integrity, and tumor suppression through non-cell autonomous signaling [88,90,92,95]. Consistently, our study found TP53 to be the most mutated gene in urinary tumors and LIHC, with a higher mutation frequency in patients expressing higher ALYREF, underscoring the significance of TP53 in cancer. Given the prevalence of TP53 mutations in cancers, efforts are ongoing to restore mutant p53 functionality, aiming to induce tumor cell death and eradication [89]. In this study, we observed that our research suggests that ALYREF has a broad potential role in various cancers, particularly in urinary tumors and LIHC.
Our findings suggest that ALYREF could serve as an oncogenic biomarker in many cancer patients and should be given more attention by researchers.
Acknowledgement: We appreciated the Figdraw (www.figdraw.com) and Chengdu Basebiotech Co., Ltd., for their assistance in drawing and data process.
Funding Statement: This program was supported by the Chinese Scholarship Council (Grant No. 202206240086), the National Natural Science Foundation of China (Grant No. 82170432) and programs from Science and Technology Department of Sichuan Province (Grant No. 2020YFSY0024). The funders had no role in the study design, data collection or analysis, preparation of the manuscript, or the decision to publish.
Author Contributions: XY and ZTT proposed the project, conducted data analysis, interpreted the data, and wrote the manuscript; XY, KC, RCW and DCF conducted data analysis, interpreted the data; DXL and DCF supervised the project, and interpreted the data. All authors reviewed and edited the manuscript.
Availability of Data and Materials: The results showed here are in whole or part based upon data generated by the TCGA Research Network: https://www.cancer.gov/tcga.
Ethics Approval: Not applicable.
Conflicts of Interest: The authors have no conflicts of interest to declare.
References
1. Chang, H. Y., Qi, L. S. (2023). Reversing the central dogma: RNA-guided control of DNA in epigenetics and genome editing. Molecular Cell, 83(3), 442–451. [Google Scholar] [PubMed]
2. Song, Y. (2021). Central dogma, redefined. Nature Chemical Biology, 17(8), 839. [Google Scholar] [PubMed]
3. Saw, P. E., Xu, X., Chen, J., Song, E. W. (2021). Non-coding RNAs: The new central dogma of cancer biology. Science China. Life Sciences, 64(1), 22–50. [Google Scholar] [PubMed]
4. Hogg, S. J., Beavis, P. A., Dawson, M. A., Johnstone, R. W. (2020). Targeting the epigenetic regulation of antitumour immunity. Nature Reviews Drug Discovery, 19(11), 776–800. [Google Scholar] [PubMed]
5. Roux, B., Picou, F., Debeissat, C., Koubi, M., Gallay, N. et al. (2022). Aberrant DNA methylation impacts HOX genes expression in bone marrow mesenchymal stromal cells of myelodysplastic syndromes and de novo acute myeloid leukemia. Cancer Gene Therapy, 29(8–9), 1263–1275. [Google Scholar] [PubMed]
6. Yu, W., Zhang, C., Wang, Y., Tian, X., Miao, Y. et al. (2023). YAP 5-methylcytosine modification increases its mRNA stability and promotes the transcription of exosome secretion-related genes in lung adenocarcinoma. Cancer Gene Therapy, 30(1), 149–162. [Google Scholar] [PubMed]
7. Zhao, L. Y., Song, J., Liu, Y., Song, C. X., Yi, C. (2020). Mapping the epigenetic modifications of DNA and RNA. Protein & Cell, 11(11), 792–808. [Google Scholar]
8. Orsolic, I., Carrier, A., Esteller, M. (2023). Genetic and epigenetic defects of the RNA modification machinery in cancer. Trends in Genetics, 39(1), 74–88. [Google Scholar] [PubMed]
9. Ding, Z., Liu, Y., Huang, Q., Cheng, C., Song, L. et al. (2023). m6A-and immune-related lncRNA signature confers robust predictive power for immune efficacy in lung squamous cell carcinoma. View, 3(4), 20220083. [Google Scholar]
10. Cohn, W. E. (1960). Pseudouridine, a carbon-carbon linked ribonucleoside in ribonucleic acids: Isolation, structure, and chemical characteristics. The Journal of Biological Chemistry, 235, 1488–1498. [Google Scholar] [PubMed]
11. Holley, R. W., Everett, G. A., Madison, J. T., Zamir, A. (1965). Nucleotide sequences in the yeast alanine transfer ribonucleic acid. The Journal of Biological Chemistry, 240, 2122–2128. [Google Scholar] [PubMed]
12. Barbieri, I., Kouzarides, T. (2020). Role of RNA modifications in cancer. Nature Reviews. Cancer, 20(6), 303–322. [Google Scholar] [PubMed]
13. Li, X., Ma, B., Zhang, W., Song, Z., Zhang, X. et al. (2023). The essential role of N6-methyladenosine RNA methylation in complex eye diseases. Genes & Diseases, 10(2), 505–520. [Google Scholar]
14. Chen, M., Wei, L., Law, C. T., Tsang, F. H., Shen, J. et al. (2018). RNA N6-methyladenosine methyltransferase-like 3 promotes liver cancer progression through YTHDF2-dependent posttranscriptional silencing of SOCS2. Hepatology, 67(6), 2254–2270. [Google Scholar] [PubMed]
15. Zhou, S., Bai, Z. L., Xia, D., Zhao, Z. J., Zhao, R. et al. (2018). FTO regulates the chemo-radiotherapy resistance of cervical squamous cell carcinoma (CSCC) by targeting beta-catenin through mRNA demethylation. Molecular Carcinogenesis, 57(5), 590–597. [Google Scholar] [PubMed]
16. Lin, X., Wang, F., Chen, J., Liu, J., Lin, Y. B. et al. (2022). N6-methyladenosine modification of CENPK mRNA by ZC3H13 promotes cervical cancer stemness and chemoresistance. Military Medical Research, 9(1), 19. [Google Scholar] [PubMed]
17. Camper, S. A., Albers, R. J., Coward, J. K., Rottman, F. M. (1984). Effect of undermethylation on mRNA cytoplasmic appearance and half-life. Molecular and Cellular Biology, 4(3), 538–543. [Google Scholar] [PubMed]
18. Finkel, D., Groner, Y. (1983). Methylations of adenosine residues (m6A) in pre-mRNA are important for formation of late simian virus 40 mRNAs. Virology, 131(2), 409–425. [Google Scholar] [PubMed]
19. Xu, L., Sun, X., Griffiths, B., Voloboueva, L., Valdes, A. et al. (2023). Sexual dimorphism in brain sirtuin-1 and m6A methylated sirtuin-1 mRNA, and in protection with post-injury anti-miR-200c treatment, after experimental stroke in aged mice. Aging and Disease, 14(3), 892–903. [Google Scholar] [PubMed]
20. Zhang, Q., Liu, F., Chen, W., Miao, H., Liang, H. et al. (2021). The role of RNA m5C modification in cancer metastasis. International Journal of Biological Sciences, 17(13), 3369–3380. [Google Scholar] [PubMed]
21. Feng, D., Shi, X., Zhu, W., Zhang, F., Li, D. et al. (2022). A pan-cancer analysis of the oncogenic role of leucine zipper protein 2 in human cancer. Experimental Hematology & Oncology, 11(1), 55. [Google Scholar]
22. Zhu, W., Feng, D., Shi, X., Li, D., Wei, Q. et al. (2022). A pan-cancer analysis of the oncogenic role of zinc finger protein 419 in human cancer. Frontiers in Oncology, 12, 1042118. [Google Scholar] [PubMed]
23. Goldman, M. J., Craft, B., Hastie, M., Repecka, K., McDade, F. et al. (2020). Visualizing and interpreting cancer genomics data via the Xena platform. Nature Biotechnology, 38(6), 675–678. [Google Scholar] [PubMed]
24. Liu, J., Lichtenberg, T., Hoadley, K. A., Poisson, L. M., Lazar, A. J. et al. (2018). An integrated TCGA pan-cancer clinical data resource to drive high-quality survival outcome analytics. Cell, 173, 400–416. [Google Scholar] [PubMed]
25. Malta, T. M., Sokolov, A., Gentles, A. J., Burzykowski, T., Poisson, L. et al. (2018). Machine learning identifies stemness features associated with oncogenic dedifferentiation. Cell, 173, 338–354. [Google Scholar] [PubMed]
26. Thorsson, V., Gibbs, D. L., Brown, S. D., Wolf, D., Bortone, D. S. et al. (2018). The immune landscape of cancer. Immunity, 48, 812–830. [Google Scholar] [PubMed]
27. Beroukhim, R., Mermel, C. H., Porter, D., Wei, G., Raychaudhuri, S. et al. (2010). The landscape of somatic copy-number alteration across human cancers. Nature, 463, 899–905. [Google Scholar] [PubMed]
28. Bonneville, R., Krook, M. A., Kautto, E. A., Miya, J., Wing, M. R. et al. (2017). Landscape of microsatellite instability across 39 cancer types. JCO Precision Oncology, 2017, PO.17.00073. [Google Scholar] [PubMed]
29. Warde-Farley, D., Donaldson, S. L., Comes, O., Zuberi, K., Badrawi, R. et al. (2010). The GeneMANIA prediction server: Biological network integration for gene prioritization and predicting gene function. Nucleic Acids Research, 38, W214–220. [Google Scholar] [PubMed]
30. Li, T., Fan, J., Wang, B., Traugh, N., Chen, Q. et al. (2017). TIMER: A web server for comprehensive analysis of tumor-infiltrating immune cells. Cancer Research, 77(21), e108–e110. [Google Scholar] [PubMed]
31. Zeng, D., Ye, Z., Shen, R., Yu, G., Wu, J. et al. (2021). IOBR: Multi-omics immuno-oncology biological research to decode tumor microenvironment and signatures. Frontiers in Immunology, 12, 687975. [Google Scholar] [PubMed]
32. Feng, D., Zhu, W., Shi, X., Wei, W., Han, P. et al. (2022). Leucine zipper protein 2 serves as a prognostic biomarker for prostate cancer correlating with immune infiltration and epigenetic regulation. Heliyon, 8(10), e10750. [Google Scholar] [PubMed]
33. Liu, K., Xia, D., Bian, H., Peng, L., Dai, S. et al. (2023). Regulator of G protein signaling-1 regulates immune infiltration and macrophage polarization in clear cell renal cell carcinoma. International Urology and Nephrology. https://doi.org/10.1007/s11255-023-03794-9 [Google Scholar] [PubMed] [CrossRef]
34. Wang, X., Lu, Y., Tuo, Z., Zhou, H., Zhang, Y. et al. (2021). Role of SIRT1/AMPK signaling in the proliferation, migration and invasion of renal cell carcinoma cells. Oncology Reports, 45(6), 109. [Google Scholar] [PubMed]
35. Adams, E., Sepich-Poore, G. D., Miller-Montgomery, S., Knight, R. (2022). Using all our genomes: Blood-based liquid biopsies for the early detection of cancer. View, 3, 20200118. [Google Scholar] [PubMed]
36. Shi, J., Chen, Y., Peng, C., Kuang, L., Zhang, Z. et al. (2022). Advances in targeted therapy against driver mutations and epigenetic alterations in non-small cell lung cancer. Oncologie, 24, 613–648. [Google Scholar]
37. Song, H., Zhang, J., Liu, B., Xu, J., Cai, B. et al. (2022). Biological roles of RNA m5C modification and its implications in cancer immunotherapy. Biomarker Research, 10(1), 15. [Google Scholar] [PubMed]
38. Cantara, W. A., Crain, P. F., Rozenski, J., McCloskey, J. A., Harris, K. A. et al. (2011). The RNA modification database, RNAMDB: 2011 update. Nucleic Acids Research, 39, D195–201. [Google Scholar] [PubMed]
39. Li, M., Tao, Z., Zhao, Y., Li, L., Zheng, J. et al. (2022). 5-methylcytosine RNA methyltransferases and their potential roles in cancer. Journal of Translational Medicine, 20(1), 214. [Google Scholar] [PubMed]
40. Garcia-Vilchez, R., Sevilla, A., Blanco, S. (2019). Post-transcriptional regulation by cytosine-5 methylation of RNA. Biochimica Et Biophysica Acta. Gene Regulatory Mechanisms, 1862(3), 240–252. [Google Scholar] [PubMed]
41. Janin, M., Ortiz-Barahona, V., de Moura, M. C., Martinez-Cardus, A., Llinas-Arias, P. et al. (2019). Epigenetic loss of RNA-methyltransferase NSUN5 in glioma targets ribosomes to drive a stress adaptive translational program. Acta Neuropathologica, 138(6), 1053–1074. [Google Scholar] [PubMed]
42. Blanco, S., Dietmann, S., Flores, J. V., Hussain, S., Kutter, C. et al. (2014). Aberrant methylation of tRNAs links cellular stress to neuro-developmental disorders. The EMBO Journal, 33(18), 2020–2039. [Google Scholar] [PubMed]
43. Nombela, P., Miguel-Lopez, B., Blanco, S. (2021). The role of m(6)A, m(5)C and Psi RNA modifications in cancer: Novel therapeutic opportunities. Molecular Cancer, 20(1), 18. [Google Scholar] [PubMed]
44. Huang, T., Chen, W., Liu, J., Gu, N., Zhang, R. (2019). Genome-wide identification of mRNA 5-methylcytosine in mammals. Nature Structural & Molecular Biology, 26(5), 380–388. [Google Scholar]
45. Chen, X., Li, A., Sun, B. F., Yang, Y., Han, Y. N. et al. (2019). 5-methylcytosine promotes pathogenesis of bladder cancer through stabilizing mRNAs. Nature Cell Biology, 21(8), 978–990. [Google Scholar] [PubMed]
46. Yang, X., Yang, Y., Sun, B. F., Chen, Y. S., Xu, J. W. et al. (2017). 5-methylcytosine promotes mRNA export-NSUN2 as the methyltransferase and ALYREF as an m5C reader. Cell Research, 27(5), 606–625. [Google Scholar] [PubMed]
47. Wang, N., Chen, R. X., Deng, M. H., Wei, W. S., Zhou, Z. H. et al. (2023). m(5)C-dependent cross-regulation between nuclear reader ALYREF and writer NSUN2 promotes urothelial bladder cancer malignancy through facilitating RABL6/TK1 mRNAs splicing and stabilization. Cell Death & Disease, 14(2), 139. [Google Scholar]
48. Wang, J. Z., Zhu, W., Han, J., Yang, X., Zhou, R. et al. (2021). The role of the HIF-1α/ALYREF/PKM2 axis in glycolysis and tumorigenesis of bladder cancer. Cancer Communications, 41(7), 560–575. [Google Scholar] [PubMed]
49. Klec, C., Knutsen, E., Schwarzenbacher, D., Jonas, K., Pasculli, B. et al. (2022). ALYREF, a novel factor involved in breast carcinogenesis, acts through transcriptional and post-transcriptional mechanisms selectively regulating the short NEAT1 isoform. Cellular and Molecular Life Sciences, 79(7), 391. [Google Scholar] [PubMed]
50. Nagy, Z., Seneviratne, J. A., Kanikevich, M., Chang, W., Mayoh, C. et al. (2021). An ALYREF-MYCN coactivator complex drives neuroblastoma tumorigenesis through effects on USP3 and MYCN stability. Nature Communications, 12(1), 1881. [Google Scholar] [PubMed]
51. Xue, C., Gu, X., Zheng, Q., Shi, Q., Yuan, X. et al. (2023). ALYREF mediates RNA m5C modification to promote hepatocellular carcinoma progression. Signal Transduction and Targeted Therapy, 8(1), 130. [Google Scholar] [PubMed]
52. Wang, Z. Z., Meng, T., Yang, M. Y., Wang, W., Zhang, Y. et al. (2022). ALYREF associated with immune infiltration is a prognostic biomarker in hepatocellular carcinoma. Translational Oncology, 21, 101441. [Google Scholar] [PubMed]
53. Wang, J., Li, Y., Xu, B., Dong, J., Zhao, H. et al. (2021). ALYREF drives cancer cell proliferation through an ALYREF-MYC positive feedback loop in glioblastoma. OncoTargets and Therapy, 14, 145–155. [Google Scholar] [PubMed]
54. Yang, Q., Wang, M., Xu, J., Yu, D., Li, Y. et al. (2023). LINC02159 promotes non-small cell lung cancer progression via ALYREF/YAP1 signaling. Molecular Cancer, 22(1), 122. [Google Scholar] [PubMed]
55. Pal, S., Tyler, J. K. (2016). Epigenetics and aging. Science Advances, 2(7), e1600584. [Google Scholar] [PubMed]
56. Lopez-Otin, C., Blasco, M. A., Partridge, L., Serrano, M., Kroemer, G. (2023). Hallmarks of aging: An expanding universe. Cell, 186(2), 243–278. [Google Scholar] [PubMed]
57. Health Commission of the People’s Republic of China (2022). National guidelines for diagnosis and treatment of lung cancer 2022 in China (English version). Chinese Journal of Cancer Research, 34(3), 176–206. [Google Scholar]
58. Health Commission of the People’s Republic of China (2022). National guidelines for diagnosis and treatment of prostate cancer 2022 in China (English version). Chinese Journal of Cancer Research, 34(3), 270–288. [Google Scholar]
59. Shen, W., He, J., Hou, T., Si, J., Chen, S. (2022). Common pathogenetic mechanisms underlying aging and tumor and means of interventions. Aging and Disease, 13(4), 1063–1091. [Google Scholar] [PubMed]
60. Lu, J., Li, H., Zhang, G., Yang, F., Zhang, X. et al. (2023). Age-related alterations in peripheral immune landscape with magnified impact on post-stroke brain. Research, 6, 287. [Google Scholar]
61. Du, J., Xu, M., Kong, F., Zhu, P., Mao, Y. et al. (2022). CB2R attenuates intervertebral disc degeneration by delaying nucleus pulposus cell senescence through AMPK/GSK3β pathway. Aging and Disease, 13(2), 552–567. [Google Scholar] [PubMed]
62. Deng, Y., Qiao, L., Du, M., Qu, C., Wan, L. et al. (2022). Age-related macular degeneration: Epidemiology, genetics, pathophysiology, diagnosis, and targeted therapy. Genes & Diseases, 9(1), 62–79. [Google Scholar]
63. Zhu, R., Ji, X., Wu, X., Chen, J., Li, X. et al. (2022). Melatonin antagonizes ovarian aging via YTHDF2-MAPK-NF-κB pathway. Genes & Diseases, 9(2), 494–509. [Google Scholar]
64. Cheng, G., Xie, A., Yan, Z., Zhu, X., Song, Y. et al. (2023). Nanomedicines for Alzheimer’s disease: Therapies based on pathological mechanisms. Brain-X, 1, e27. [Google Scholar]
65. Chapman, N. M., Boothby, M. R., Chi, H. (2020). Metabolic coordination of T cell quiescence and activation. Nature Reviews. Immunology, 20(1), 55–70. [Google Scholar] [PubMed]
66. Picarda, E., Ohaegbulam, K. C., Zang, X. (2016). Molecular pathways: Targeting B7-H3 (CD276) for human cancer immunotherapy. Clinical Cancer Research, 22(14), 3425–3431. [Google Scholar] [PubMed]
67. Kishton, R. J., Sukumar, M., Restifo, N. P. (2017). Metabolic regulation of T cell longevity and function in tumor immunotherapy. Cell Metabolism, 26(1), 94–109. [Google Scholar] [PubMed]
68. Saibil, S. D., Ohashi, P. S. (2020). Targeting T cell activation in immuno-oncology. Current Oncology, 27(Suppl 2), S98–S105. [Google Scholar] [PubMed]
69. Yang, S., Wei, W., Zhao, Q. (2020). B7-H3, a checkpoint molecule, as a target for cancer immunotherapy. International Journal of Biological Sciences, 16(11), 1767–1773. [Google Scholar] [PubMed]
70. Kontos, F., Michelakos, T., Kurokawa, T., Sadagopan, A., Schwab, J. H. et al. (2021). B7-H3: An attractive target for antibody-based immunotherapy. Clinical Cancer Research, 27(5), 1227–1235. [Google Scholar] [PubMed]
71. Zhou, W. T., Jin, W. L. (2021). B7-H3/CD276: An emerging cancer immunotherapy. Frontiers in Immunology, 12(1), 701006. [Google Scholar] [PubMed]
72. Stelzer, G., Rosen, N., Plaschkes, I., Zimmerman, S., Twik, M. et al. (2016). The geneCards suite: From gene data mining to disease genome sequence analyses. Current Protocols in Bioinformatics, 54. [Google Scholar]
73. Schmitz, B., Radbruch, A., Kummel, T., Wickenhauser, C., Korb, H. et al. (1994). Magnetic activated cell sorting (MACS)–A new immunomagnetic method for megakaryocytic cell isolation: Comparison of different separation techniques. European Journal of Haematology, 52(5), 267–275. [Google Scholar] [PubMed]
74. Basu, S., Campbell, H. M., Dittel, B. N., Ray, A. (2010). Purification of specific cell population by fluorescence activated cell sorting (FACS). JoVE-Journal of Visualized Experiments, (41), 1546. [Google Scholar]
75. Zheng, X., Zhang, N., Wu, H. J., Wu, H. (2017). Estimating and accounting for tumor purity in the analysis of DNA methylation data from cancer studies. Genome Biology, 18(1), 17. [Google Scholar] [PubMed]
76. Guan, H., Wu, Y., Li, L. U., Yang, Y., Qiu, S. et al. (2023). Tumor neoantigens: Novel strategies for application of cancer immunotherapy. Oncology Research, 31(4), 437–448. [Google Scholar] [PubMed]
77. Samstein, R. M., Lee, C. H., Shoushtari, A. N., Hellmann, M. D., Shen, R. et al. (2019). Tumor mutational load predicts survival after immunotherapy across multiple cancer types. Nature Genetics, 51(2), 202–206. [Google Scholar] [PubMed]
78. Sholl, L. M., Hirsch, F. R., Hwang, D., Botling, J., Lopez-Rios, F. et al. (2020). The promises and challenges of tumor mutation burden as an immunotherapy biomarker: A perspective from the international association for the study of lung cancer pathology committee. Journal of Thoracic Oncology, 15(9), 1409–1424. [Google Scholar] [PubMed]
79. Jardim, D. L., Goodman, A., de Melo Gagliato, D., Kurzrock, R. (2021). The challenges of tumor mutational burden as an immunotherapy biomarker. Cancer Cell, 39(2), 154–173. [Google Scholar] [PubMed]
80. Chan, T. A., Yarchoan, M., Jaffee, E., Swanton, C., Quezada, S. A. et al. (2019). Development of tumor mutation burden as an immunotherapy biomarker: Utility for the oncology clinic. Annals of Oncology, 30(1), 44–56. [Google Scholar] [PubMed]
81. Zheng, Y., Su, Y., Ruan, L., He, Q., Gong, L. et al. (2023). Screening and biomarker assessment of ferroptosis genes FLT3 and ALOX5 in lung adenocarcinoma. Oncologie, 25, 281–289. [Google Scholar]
82. Chen, H., Ma, X., Liu, J., Yang, Y., Fang, Y. et al. (2022). Clinical outcomes of atezolizumab in combination with etoposide/platinum for treatment of extensive-stage small-cell lung cancer: A real-world, multicenter, retrospective, controlled study in China. Chinese Journal of Cancer Research = Chung-Kuo Yen Cheng Yen Chiu, 34(4), 353–364. [Google Scholar] [PubMed]
83. Yu, S., Ma, J. (2022). Spindle and kinetochore-associated complex is associated with poor prognosis in adrenocortical carcinoma. The Journal of Surgical Research, 277, 50–59. [Google Scholar] [PubMed]
84. Wu, K., Liu, Z., Liang, J., Zhu, Y., Wang, X. et al. (2023). Discovery of a glucocorticoid receptor (GR) activity signature correlates with immune cell infiltration in adrenocortical carcinoma. Journal for Immunotherapy of Cancer, 11, e007528. [Google Scholar] [PubMed]
85. Lin, Y., Wu, Z. (2023). Hypoxia-inducible factor 1α (HIF-1α)-activated Gli1 induces invasion and EMT by H3K4 methylation in glioma cells. Oncologie, 25, 71–79. [Google Scholar]
86. Health Commission of the People’s Republic of China (2022). National guidelines for diagnosis and treatment of malignant lymphoma 2022 in China (English version). Chinese Journal of Cancer Research, 34(5), 425–446. [Google Scholar]
87. Tilly, H., Gomes da Silva, M., Vitolo, U., Jack, A., Meignan, M. et al. (2015). Diffuse large B-cell lymphoma (DLBCLESMO clinical practice guidelines for diagnosis, treatment and follow-up. Annals of Oncology, 26(Suppl 5), v116–125. [Google Scholar] [PubMed]
88. Boutelle, A. M., Attardi, L. D. (2021). p53 and tumor suppression: It takes a network. Trends in Cell Biology, 31(4), 298–310. [Google Scholar] [PubMed]
89. Bykov, V. J. N., Eriksson, S. E., Bianchi, J., Wiman, K. G. (2018). Targeting mutant p53 for efficient cancer therapy. Nature Reviews Cancer, 18(2), 89–102. [Google Scholar] [PubMed]
90. Hernandez Borrero, L. J., El-Deiry, W. S. (2021). Tumor suppressor p53: Biology, signaling pathways, and therapeutic targeting. Biochimica Et Biophysica Acta. Reviews on Cancer, 1876(1), 188556. [Google Scholar] [PubMed]
91. Hu, J., Cao, J., Topatana, W., Juengpanich, S., Li, S. et al. (2021). Targeting mutant p53 for cancer therapy: Direct and indirect strategies. Journal of Hematology & Oncology, 14(1), 157. [Google Scholar]
92. Kastenhuber, E. R., Lowe, S. W. (2017). Putting p53 in context. Cell, 170, 1062–1078. [Google Scholar] [PubMed]
93. Wang, Z., Strasser, A., Kelly, G. L. (2022). Should mutant TP53 be targeted for cancer therapy? Cell Death and Differentiation, 29(5), 911–920. [Google Scholar] [PubMed]
94. Zuckerman, V., Wolyniec, K., Sionov, R. V., Haupt, S., Haupt, Y. (2009). Tumour suppression by p53: The importance of apoptosis and cellular senescence. The Journal of Pathology, 219(1), 3–15. [Google Scholar] [PubMed]
95. Lacroix, M., Riscal, R., Arena, G., Linares, L. K., Le Cam, L. (2020). Metabolic functions of the tumor suppressor p53: Implications in normal physiology, metabolic disorders, and cancer. Molecular Metabolism, 33, 2–22. [Google Scholar] [PubMed]
Supplementary Materials
Figure S1: The correlation between ALYREF expression and clinical features at pan-cancer level. (a) ALYREF expression differences among different T stages; (b) ALYREF expression differences among different N stages; (c) ALYREF expression differences between M0 and M1 groups; (d) ALYREF expression differences among different clinical stages; (e) ALYREF expression differences among different grades; (f)ALYREF expression differences between female and male groups. *p < 0.05; **p < 0.01; ***p < 0.001; ****p < 0.0001.
Cite This Article
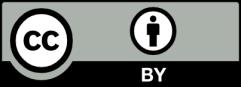
This work is licensed under a Creative Commons Attribution 4.0 International License , which permits unrestricted use, distribution, and reproduction in any medium, provided the original work is properly cited.