Open Access
ARTICLE
Leveraging diverse cell-death patterns to predict the clinical outcome of immune checkpoint therapy in lung adenocarcinoma: Based on muti-omics analysis and vitro assay
1 Department of Radiology, Shengjing Hospital of China Medical University, Shenyang, China
2 Department of Nephrology, The First Affiliated Hospital of China Medical University, Shenyang, China
3 Department of Clinical Medicine, China Medical University, Shenyang, China
4 Department of Medical Oncology, The First Hospital of China Medical University, Shenyang, China
* Corresponding Author: LINGYUN ZHANG. Email:
# These authors contributed equally to this work
(This article belongs to the Special Issue: Multi-Omics Approaches for Precision Medicine)
Oncology Research 2024, 32(2), 393-407. https://doi.org/10.32604/or.2023.031134
Received 16 May 2023; Accepted 06 September 2023; Issue published 28 December 2023
Abstract
Advanced LUAD shows limited response to treatment including immune therapy. With the development of sequencing omics, it is urgent to combine high-throughput multi-omics data to identify new immune checkpoint therapeutic response markers. Using GSE72094 (n = 386) and GSE31210 (n = 226) gene expression profile data in the GEO database, we identified genes associated with lung adenocarcinoma (LUAD) death using tools such as “edgeR” and “maftools” and visualized the characteristics of these genes using the “circlize” R package. We constructed a prognostic model based on death-related genes and optimized the model using LASSO-Cox regression methods. By calculating the cell death index (CDI) of each individual, we divided LUAD patients into high and low CDI groups and examined the relationship between CDI and overall survival time by principal component analysis (PCA) and Kaplan-Meier analysis. We also used the “ConsensusClusterPlus” tool for unsupervised clustering of LUAD subtypes based on model genes. In addition, we collected data on the expression of immunomodulatory genes and model genes for each cohort and performed tumor microenvironment analyses. We also used the TIDE algorithm to predict immunotherapy responses in the CDI cohort. Finally, we studied the effect of PRKCD on the proliferation and migration of LUAD cells through cell culture experiments. The study utilized the TCGA-LUAD cohort (n = 493) and identified 2,901 genes that are differentially expressed in patients with LUAD. Through KEGG and GO enrichment analysis, these genes were found to be involved in a wide range of biological pathways. The study also used univariate Cox regression models and LASSO regression analyses to identify 17 candidate genes that were best associated with mortality prognostic risk scores. By comparing the overall survival (OS) outcomes of patients with different CDI values, it was found that increased CDI levels were significantly associated with lower OS rates. In addition, the study used unsupervised cluster analysis to divide 115 LUAD patients into two distinct clusters with significant differences in OS timing. Finally, a prognostic indicator called CDI was established and its feasibility as an independent prognostic indicator was evaluated by Cox proportional risk regression analysis. The immunotherapy efficacy was more sensitive in the group with high expression of programmed cell death models. Relationship between programmed cell death (PCD) signature models and drug reactivity. After evaluating the median inhibitory concentration (IC50) of various drugs in LUAD samples, statistically significant differences in IC50 values were found in cohorts with high and low CDI status. Specifically, Gefitinib and Lapatinib had higher IC50 values in the high-CDI cohort, while Olaparib, Oxaliplatin, SB216763, and Axitinib had lower values. These results suggest that individuals with high CDI levels are sensitive to tyrosine kinase inhibitors and may be resistant to conventional chemotherapy. Therefore, this study constructed a gene model that can evaluate patient immunotherapy by using programmed cell death-related genes based on muti-omics. The CDI index composed of these programmed cell death-related genes reveals the heterogeneity of lung adenocarcinoma tumors and serves as a prognostic indicator for patients.Keywords
Supplementary Material
Supplementary Material FileCite This Article
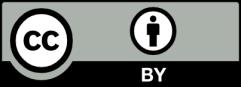
This work is licensed under a Creative Commons Attribution 4.0 International License , which permits unrestricted use, distribution, and reproduction in any medium, provided the original work is properly cited.