Open Access
ARTICLE
Comprehensive bioinformatics analysis and experimental validation: An anoikis-related gene prognostic model for targeted drug development in head and neck squamous cell carcinoma
1 Central Laboratory, Peking University School and Hospital of Stomatology & National Center for Stomatology & National Clinical Research Center for Oral Diseases & National Engineering Research Center of Oral Biomaterials and Digital Medical Devices, Beijing, 100081, China
2 Department of Dentistry, Xuanwu Hospital Capital Medical University, Beijing, 100053, China
3 National Clinical Research Center for Geriatric Disorders, Beijing, 100101, China
4 School of Medicine, Nankai University, Tianjin, 300071, China
5 Department of Oral and Maxillofacial Surgery, Tianjin Stomatological Hospital, School of Medicine, Nankai University, Tianjin, 300041, China
* Corresponding Authors: XIANPENG GE. Email: ; CUIYING LI. Email:
# Lin Qiu and Anqi Tao have contributed equally to this work
Oncology Research 2023, 31(5), 715-752. https://doi.org/10.32604/or.2023.029443
Received 19 February 2023; Accepted 17 May 2023; Issue published 21 July 2023
Abstract
We analyzed RNA-sequencing (RNA-seq) and clinical data from head and neck squamous cell carcinoma (HNSCC) patients in The Cancer Genome Atlas (TCGA) Genomic Data Commons (GDC) portal to investigate the prognostic value of anoikis-related genes (ARGs) in HNSCC and develop new targeted drugs. Differentially expressed ARGs were screened using bioinformatics methods; subsequently, a prognostic model including three ARGs (CDKN2A, BIRC5, and PLAU) was constructed. Our results showed that the model-based risk score was a good prognostic indicator, and the potential of the three ARGs in HNSCC prognosis was validated by the TISCH database, the model’s accuracy was validated in two independent cohorts of the Gene Expression Omnibus database. Immune correlation analysis and half-maximal inhibitory concentration were also performed to reveal the different landscapes of TIME between risk groups and to predict immuno- and chemo-therapeutic responses. Potential small-molecule drugs for HNSCC were subsequently predicted using the L1000FWD database. Finally, in vitro experiments were used to verify the database findings. The relative ARG mRNA expression levels in HNSCC and surrounding normal tissues remained consistent with the model results. BIRC5 knockdown inhibited anoikis resistance in WSU-HN6 and CAL-27 cells. Molecular docking, real-time PCR, cell counting kit-8 (CCK-8), plate clone, and flow cytometry analyses showed that small-molecule drugs predicted by the database may target the ARGs in the prognostic model, inhibit HNSCC cells survival rate, and promote anoikis in vitro. Therefore, we constructed a new ARG model for HNSCC patients that can predict prognosis and immune activity and identify a potential small-molecule drug for HNSCC, paving the way for clinically targeting anoikis in HNSCC.Keywords
Head and neck cancer (HNC) is the sixth most common malignancy and the leading cause of death and decreased life expectancy worldwide. Oral cavity, oropharynx, and nasopharynx tumors are included in HNC. Over 500,000 fatal HNC cases occurred in 2020, and over one million new cases were diagnosed [1]. Epidemiological studies show that the incidence of HNC is rising annually, with a progressive trend toward a higher incidence in younger people. Squamous cell carcinoma accounts for over 90% of HNC [2]. Although tremendous strides have been made in head and neck squamous cell carcinoma (HNSCC) treatment, the 5-year survival rate remains below 50% since most HNSCC patients have advanced disease at diagnosis [3]. Ninety percent of cancer patients die from metastases [4]; lymph node metastases are the most common in HNSCC and are a key prognostic indicator. The 5-year survival rate is considerably poorer for HNSCC patients who experience lymph node metastases [5,6]. It is essential to develop a predictive model for HNSCC metastasis that occurs before lymph node metastasis to improve patient prognosis.
In general, most cells survive by adhering tightly to the extracellular matrix (ECM) or other cells, and cell death occurs once cells are separated from the ECM. This form of cell death was first named “anoikis” in 1994 [7]. Anoikis is a special type of apoptotic cell death with different cell morphology and biochemical markers from those of ferroptosis, necroptosis, and autophagy [8,9]. Anoikis exhibits some features of apoptosis, mainly depends on the cell-matrix interactions, and plays a critical role in cancer metastasis [7]. Tumor metastasis, by contrast, assumes that cancer cells have evolved resistance to anoikis. When cancer cells are liberated from their cell–ECM and cell–cell adhesion states, they survive, disseminate, and metastasize in the circulatory system by resisting anoikis-induced tumor cell death via autocrine or paracrine pathways [10,11].
The significance of anoikis in HNC is becoming clearer as research and genetic testing procedures develop, and anoikis resistance acquisition has been hypothesized to be a critical step in oral cancer metastasis [12]. The signaling pathways associated with anoikis resistance in HNSCC have been the subject of numerous recent investigations [13,14]. For instance, it has been proposed that the epithelial–mesenchymal transition (EMT) plays a significant role in the development of anoikis resistance in cancer cells [13]. Several scientists have investigated the changes in gene expression that occur after HNSCC cells develop anoikis resistance [14]. However, which genes are essential for HNSCC anoikis resistance and how anoikis-related genes (ARGs) affect patient prognosis remains uncertain. In addition, targeting ARGs may help develop effective drugs that can reduce metastasis in HNSCC patients.
We searched for novel prognostic indicators to explore the role of ARGs in prognosis, predicted potential effective drugs for HNSCC, and introduced new methods for the existing relatively mature comprehensive sequence therapy model. We used bioinformatics methods to construct a high-accuracy ARG-based prognostic model. Subsequently, a small-molecule drug was obtained from the L1000FWD database, and its targeting of the prognostic model genes was validated.
Data and clinical sample collection
We obtained RNA sequencing (RNA-seq) and clinical information about HNSCC from The Cancer Genome Atlas (TCGA) Genomic Data Commons (GDC) portal (https://portal.gdc.cancer.gov/) and the Gene Expression Omnibus (GEO) database (datasets: GSE27020 n = 99 and GSE41613 n = 76), then removed data with missing survival status, unknown survival time, or survival < 30 days. The single cell RNA sequencing (scRNA-seq) of HNSCC patients was obtained from the TISCH (http://tisch.comp-genomics.org/). (dataset: HNSCC_GSE103322, cell number = 5902). GeneCards was applied to obtain (https://www.genecards.org/) a total of 419 genes (relevance score with anoikis > 0.4) (Suppl. Table S1). Three pairs of HNSCC samples were obtained for real-time PCR (RT–PCR) and were preserved in liquid nitrogen and frozen at −80°C. Our study protocol was approved by the Biomedical Ethics Committee of Peking University Stomatological Hospital. The code used in this study can be found on GitHub (https://github.com/qiulin961028/anoikis-analysis.git), and the study flow chart is shown in Fig. 1.
Figure 1: Study design flowchart.
RNA-seq data of HNSCC patients were analyzed by log2 transformation. Differentially expressed ARGs were subsequently screened and clustered using the R software (4.1.2) LIMMA package. Univariate and multivariate COX analyses were performed to identify HNSCC prognosis-related ARGs for subsequent prognostic model construction.
Construction and validation of the ARG prognostic model
After the multivariate COX regression analysis, the risk regression coefficients and expressions of each ARG were combined to establish the risk score formula, which led to the prognostic model, calculated as follows:
Coef represents the risk regression coefficient of ARGs, and X represents the ARG expression level. The risk score of each HNSCC patient was measured, and all patients were divided into high- or low-risk groups in accordance with the median risk score. Immediately afterward, the overall survival (OS) outcomes of HNSCC patients were compared between the two risk groups by survival analysis. Receiver operating characteristic (ROC) curves were used to test the accuracy of model predictions. The role of the risk score in predicting prognosis was investigated by univariate and multivariate COX regression analyses.
Evaluation of immune cell infiltration and prediction of drug therapy response
We quantified differences in immune cell infiltration and immune function in the high- and low-risk groups using ssGSEA. We used the Genomics of Cancer Drug Sensitivity in Cancer (https://www.cancerrxgene.org) to forecast the sensitivity to drug therapies as per the half-maximal inhibitory concentration (IC50) in the two groups.
Potential small-molecule drug prediction
Differentially expressed ARGs were classified into up- or down-regulated groups and imported into the L1000FWD website (https://maayanlab.cloud/L1000FWD/) to obtain outcomes. The top three drug structures are shown on the PubChem website (PubChem.ncbi.nlm.nih.gov).
The human HNSCC cell lines WSU-HN6 and CAL-27 and the human normal oral keratinocyte epithelial cell line HOK were used in this study. WSU-HN6 and HOK cell lines were obtained from Ninth People’s Hospital, Shanghai Jiao Tong University School of Medicine (Shanghai, China), and the CAL-27 cell line was purchased from American Type Culture Collection (ATCC, Manassas, Virginia, USA). All cells were passaged and preserved at the Central Laboratory of Peking University Hospital of Stomatology and regularly tested to ensure mycoplasma negativity. The methods for detecting mycoplasma in cells were conducted as described previously [15]. All cells were cultured in high-glucose Dulbecco’s Modified Eagle Medium (DMEM) (WSU-HN6 and CAL-27) or Roswell Park Memorial Institute (RPMI) 1640 medium (HOK) (Gibco, Carlsbad, CA, USA) containing 10% fetal bovine serum (Gibco, Carlsbad, CA, USA) and 1% penicillin/streptomycin solution (Solarbio Science & Technology Co., Ltd., Beijing, China) at 37°C and 5% CO2.
Small interfering RNA transfection
The cells were cultured in a 6-well plate at a density of 2.0 × 105 cells/well, and two groups were established: the control group (si-NC) and the interference group (si-BIRC5). According to the recommended protocol, the working concentration of siRNA was 50 nM, and the Lipo8000 (Beyotime Biotechnology Co., Ltd., Shanghai, China) transfection reagent was used. After 24–72 h of culture, the cells were collected for follow-up experiments. The expression of ARG after knockdown was determined by RT–PCR. The siRNAs were synthesized by Tsingke Biotechnology Co., Ltd., China, with the following sequences: si-BIRC5: CCGCATCTCTACATTCAAGAA.
Total RNA was extracted from cells and tissues using TRIzol (Beyotime Biotechnology Co., Ltd., Shanghai, China) and reverse transcribed into cDNA using a Prime Script™ RT Kit (TaKaRa Biotechnology Co., Ltd., Tokyo, Japan). The cDNA template was subsequently amplified by RT–PCR using SYBR Green qPCR Master Mix (Abclonal Technology, Wuhan, China). GAPDH was used as an internal reference, and relative mRNA expression was measured by the 2–ΔΔCT method. All primers were purchased from Sangon Biotech (Shanghai, China). The primer sequences are shown below:
GAPDH:
Forward 5′-GCACCGTCAAGGCTGAGAAC-3′,
Reverse 5′-TGGTGAAGACGCCAGTGGA-3′;
CDKN2A:
Forward 5′-CCGAATAGTTACGGTCGGAGG-3′,
Reverse 5′-CACCAGCGTGTCCAGGAAG-3′;
BIRC5:
Forward 5′-TCTGTCACGTTCTCCACACG-3′,
Reverse 5′-GACCTCCAGAGGTTTCCAGC-3′;
PLAU:
Forward 5′-GAGTGCGCTCTTGCTTTGAC-3′,
Reverse 5′-GTGGATGGAATCCGGAGGAC-3′.
Molecular docking was performed to analyze the binding capacity of radicicol and ARGs. The PubChem database (https://pubchem.ncbi.nlm.nih.gov/) was accessed to download the radicicol structure, and the protein structure was obtained from the Protein Data Bank (PDB; http://www.rcsb.org/). Hydrogen atoms were added, charges were calculated, and charges and bonds on small molecules were adjusted using AutoDockTools (version 1.5.6), and the data were stored in a PDBQT file. Vina was used to calculate the binding energy, and PyMOL (version 4.6.0) was used to visualize the optimal binding model.
Two HNSCC cell lines were inoculated in 96-well plates at 100 μL per well (3000 cells/well) and incubated (37°C and 5% CO2). Cell viability was determined using the cell counting kit-8 (CCK-8) assay (Solarbio Science & Technology Co., Ltd., Beijing, China). Ten microliters of CCK-8 solution was added to each well at the appropriate time point (24, 48, and 72 h), and the plate was incubated for 2 h in the dark. The optical density (OD) of each well was measured at 450 nm using a microplate reader (Tecan, Männedorf, Zürich, Switzerland), and cell viability was calculated as follows: cell viability = [(experimental wells’ OD − blank wells’ OD)/(control wells’ OD − blank wells’ OD)] × 100%)].
Two HNSCC cell lines were inoculated in 6-well plates at 1000 cells/well, and 2 mL of medium was added to each well. The culture medium was changed every two days, and the culture was terminated when cell clones were visible to the eye. The cells were washed with phosphate-buffered saline (PBS), fixed with 4% paraformaldehyde (Beyotime Biotechnology Co., Ltd., Shanghai, China) at 4°C for 15 min, and then stained with 0.1% crystal violet (Beyotime Biotechnology Co., Ltd., Shanghai, China) for 10 min at room temperature.
The treated HNSCC cells were collected in 1.5 mL centrifuge tubes, resuspended in precooled 70% ethanol, and fixed overnight at 4°C. After rinsing twice with precooled PBS, 25 μL of PI, 500 μL of staining buffer, and 10 μL of RNase A (Beyotime Biotechnology Co., Ltd., Shanghai, China) were added to each sample for 30 min at 37°C, or samples were stained with 5 μL of Annexin V-FITC and 5 μL of PI for 15 min at room temperature (Solarbio Science & Technology Co., Ltd., Beijing, China). Cell cycle and apoptosis analyses were performed by Calibur flow cytometry (Becton, Dickinson and Company, Franklin Lakes, New Jersey, USA).
R software (version 4.1; PBC, Boston, MA, USA) and GraphPad Prism 7.0 (version 8.0; La Jolla, CA, USA) were used for the statistical analyses. All data are expressed as the mean ± standard deviation. T test and one-way ANOVA were used to compare data between two or more groups that conformed to the normal distribution, respectively. The Wilcoxon rank sum test was used for data that did not conform to a normal distribution, and p < 0.05 was considered to indicate a significant difference.
Discovery of differentially expressed ARGs
Firstly, we downloaded transcript data of 44 normal and 504 cancer samples from the TCGA. Expression values for a total of 364 ARGs were then extracted from HNSCC RNA-seq data (Suppl. Table S2). Fifty differentially expressed ARGs were obtained by screening according to the criteria of p < 0.01 and |Log2 (Fold Change)| > 2. The expression volcano plot is shown in Fig. 2A. Twelve genes were downregulated in HNSCC tissues compared with the levels in the surrounding normal tissues (LTF, PDK4, ELANE, HRC, BCL2L15, CEACAM5, CEACAM1, NTRK3, CRYAB, CCDC178, CLU, and F10), and 38 genes were upregulated (CAV1, BIRC5, TUBB3, CDH2, NTRK1, LAMB3, FADD, NOX4, CDC25C, UBE2C, ITGA6, CSPG4, FOXC2, IFI27, TNC, COL4A2, COL13A1, E2F1, AFP, MNX1, ITGA5, PLAU, BST2, SPP1, LAMA3, ADCY10, SERPINE1, FN1, SPINK1, PTHLH, CDKN2A, LAMC2, SLCO1B3, MMP9, ONECUT1, HOTAIR, MMP11, and MMP13) (Figs. 2B and 2C).
Figure 2: Differentially expressed ARGs. (A) Volcano plot of 364 differentially expressed ARGs. Blue points represent log2FC < (−2), and red points represent log2FC > 2, **p < 0.01. (B) Heatmap for 50 differentially expressed ARGs. (C) Box plot of differentially expressed ARGs.
Prognostic model establishment and verification
A univariate COX analysis was performed on the 50 ARGs, and variables with p < 0.05 in the univariate COX analysis were examined (Fig. 3A) in a multivariate analysis. A total of three ARGs (CDKN2A, BIRC5, and PLAU) were significantly associated with prognosis, leading to the construction of a prognostic model (Fig. 3B). We determined risk scores for all cases with expression levels for risk regression coefficients and ARGs. Risk score = CDKN2A expression × (−0.01388) + BIRC5 expression × 0.013027 + PLAU expression × 0.001909. All patients were ranked according to their risk scores, and the differential expression profiles for three ARGs between the high- and low-risk groups were displayed in a heatmap (Fig. 3C), showing that the CDKN2A expression level was obviously decreased in the high-risk group, whereas the BIRC5 and PLAU expression levels were both significantly increased. Further analysis of patient survival status showed that patients in the high-risk group had worse survival outcomes and a higher likelihood of death than those in the low-risk group (Figs. 3D and 3E). The subsequent survival analysis results confirmed that the overall survival (OS) rate of the high-risk group was lower than that of the low-risk group (p < 0.001) (Fig. 3F). ROC curves were used to demonstrate the predictive performance of the model, with areas under the curve (AUC) of 0.714, 0.703, and 0.704 for 1-, 3-, and 5-year OS rates, respectively (Fig. 3G), indicating the good predictability of the prognostic model for HNSCC patients.
Figure 3: Prognostic model establishment and verification. (A) Univariate COX regression analysis. (B) Multivariate COX regression analysis. (C) Heatmap for the three prognosis-related ARGs. (D) Risk score distribution chart. (E) Distribution of the survival status of the high- and low-risk groups. (F) Kaplan-Meier curves for the prognostic model in high- and low-risk groups. (G) ROC curves and AUC values for 1-, 3-, and 5-year survival. *p < 0.05; **p < 0.01; ***p < 0.001.
Risk scores independently predicted HNSCC prognosis
The relationship of each clinical parameter to the risk score was investigated by clinicopathological analysis. The results were plotted as a heatmap (Fig. 4A), which showed that the risk scores were significantly associated with N stage (p < 0.05), T stage (p < 0.001), tumor stage (p < 0.001), and grade (p < 0.01) but were not relevant to age and sex. The relationship between the risk score and M stage could not be assessed due to the lack of M stage information. The difference in the prognosis predicted by the risk score with other clinicopathological features was assessed by the multivariate ROC curve. As shown in Fig. 4B, the AUC value of the risk score was 0.714, which significantly exceeded that of the additional clinical indicators. The combined ROC curve for T stage, N stage, and risk score was then plotted (Fig. 4C). The AUC value of the combined ROC curve was 0.768, which was significantly higher than that of any other clinical feature. These results suggest that the risk score is a more accurate prognostic predictor for HNSCC patients than other clinical features and a useful complementary means to predict HNSCC patients’ prognosis according to the TNM stage. In addition, we performed univariate and multivariate COX regression analyses to verify the independent prognostic significance of the risk score for HNSCC patient OS outcomes. The univariate COX regression analysis revealed significant differences in age, sex, grade, tumor stage, and risk score (Fig. 4D), while the multivariate COX regression analysis showed that the risk score could be an independent prognostic factor for HNSCC patients (Fig. 4E) (HR = 2.134, 95% CI = 1.524–2.988). In conclusion, the superiority of the model for predicting the prognosis of HNSCC patients was demonstrated. The model-based risk score can be used as an independent prognostic factor in HNSCC patients.
Figure 4: Risk scores independently predict HNSCC prognosis. (A) Heatmap for the correlation between prognosis-related ARGs and clinical indicators. (B) ROC curves and AUC values for risk scores and clinical parameters. (C) Combined ROC curves and AUC values for T and N stage and risk score. (D) Univariate COX regression analysis. (E) Multivariate COX regression analysis. *p < 0.05; **p < 0.01; ***p < 0.001.
Investigation of immunity status and clinical treatment response analyses in high- and low-risk groups
We next evaluated the relationship between the risk model and immune infiltration. The immune cell infiltration analysis showed that the low-risk group had higher immune cell infiltration levels, such as B cells, CD8+ T cells, interdigitating dendritic cells (iDCs), macrophages, mast cells, NK cells, plasmacytoid dendritic cells (pDCs), T helper cells, follicular helper T cell (Tfh), T helper 2 cells (Th2), and tumor-infiltrating lymphocytes (TILs) (Fig. 5A). In addition, immune function was compared between the two groups, with the low-risk group demonstrating more prosperous immune function (Fig. 5B). The above results indicate a large difference in immune status between the high- and low-risk groups, with the low-risk group showing higher immune infiltration levels. Sensitivity analysis of 198 drugs was performed in HNSCC. The low-risk group had smaller IC50 in 41 drugs, including targeted drugs (e.g., Alpetisib) and immunotherapeutic agents (e.g., Ribociclib) (Fig. 5C), than the high-risk group. All 41 chemicals were shown in Suppl. Fig. S1. Twelve drugs had lower IC50 in the high-risk group (Suppl. Fig. S2), of which some drugs including Luminespib were associated with immunotherapy (Fig. 5D).
Figure 5: Immune cell infiltration and drug sensitivity analyses in high- and low-risk groups. (A) Comparison of immune cell infiltration in high- and low-risk HNSCC patients. (B) Comparison of immune function between high- and low-risk HNSCC patients. (C) Two representative drugs with lower IC50 values in the low-risk group. (D) Two representative drugs with lower IC50 values in the high-risk group. *p < 0.05; **p < 0.01; ***p < 0.001.
External validation of the prognostic model
We evaluated ARGs expression in HNSCC cells using a dataset from the TISCH database. In the dataset HNSCC_GSE103322, CDKN2A (Figs. 6A and 6B), BIRC5 (Fig. 6C), and PLAU (Fig. 6D) were mainly expressed in HNSCC malignant cells, and their proportions in malignant cells were 76.4%, 50.5%, and 58.9%, respectively (Suppl. Fig. S3). These results were consistent with the results of TCGA database analysis, and again confirmed that CDKN2A, BIRC5, and PLAU have great potential as prognostic markers in HNSCC patients. Then risk scores were calculated for each patient in the GSE27020 and GSE41613 datasets using the same formula used for the external validation of the prognostic model. All patients in the two datasets were divided into high- and low-risk groups as previously described using the median risk score for each dataset. The survival curves of the two datasets were as expected, with significantly lower OS rates in the high-risk group than in the low-risk group (p < 0.01) (Figs. 6E and 6F). The ROC curves in GSE27020 had AUC values of 0.719, 0.697, and 0.671 for 1-, 3-, and 5-year OS rates, respectively (Fig. 6G). The AUC values in GSE41613 were 0.729, 0.763, and 0.732 for 1-, 3-, and 5-year OS rates, respectively (Fig. 6H). These results again confirmed the ability of the prognostic model to predict prognosis. Unfortunately, the lack of clinical information, such as sex, T stage, and N stage, prevented the comparison of the predictive ability difference between clinical features and risk scores in the dataset. Nevertheless, the results were sufficient and highlighted the outstanding ability of the model to predict prognosis.
Figure 6: External validation of the three ARGs and prognostic model. (A-D) Distribution of CDKN2A, BIRC5, and PLAU in different cells. (E) Kaplan‒Meier curves for prognostic models of GSE27020 in the high- and low-risk groups. (F) Kaplan‒Meier curves for prognostic models of GSE41613 in the high- and low-risk groups. (G) ROC curves and AUC values for 1-, 3-, and 5-year survival in GSE27020. (H) ROC curves and AUC values for 1-, 3-, and 5-year survival in GSE41613. *p < 0.05; **p < 0.01; ***p < 0.001.
L1000FWD analysis to identify potential target drugs
We searched for potential target drugs of HNSCC by uploading up- and down-regulated ARGs to the L1000FWD database and obtaining the top ten candidates. The basic drug information is shown in Suppl. Table S3. We selected the top three small-molecule drugs (radicicol, dasatinib, and BRD-K85660637) for visualization. The 2D and 3D structures are shown in Fig. 7. Radicicol, the top-ranked fungicide, was selected for verification in a series of in vitro experiments.
Figure 7: Structure of the top three small molecule drugs. (A) The 2D structure of radicicol. (B) The 2D structure of dasatinib. (C) The 2D structure of BRD-K85660637. (D) The 3D structure of radicicol. (E) The 3D structure of dasatinib. (F) The 3D structure of BRD-K85660637.
Exploration of the expression characteristics of ARGs and their effect on anoikis in vitro
The mRNA expression level of three ARGs was detected in three pairs of matched HNSCC (T), paracancerous normal tissues (N), HOK cells, and two HNSCC cell lines. As shown in Figs. 8A and 8B, the relative mRNA expression levels of CDKN2A, BIRC5, and PLAU were higher in HNSCC tissues than in paracancerous normal tissues, while the relative mRNA expression levels of CDKN2A, BIRC5, and PLAU were also higher in both HNSCC cell lines than in HOK cells. Therefore, the expression level of ARGs was consistent with the results of our model analysis. Subsequently, we prevented cell adhesion by cultivating HNSCC cells on nonadherent plates to mimic the environment of cancer cells to verify the correlation between the ARG and anoikis. We selected one of the ARGs (BIRC5) for experimental verification and knocked down BIRC5 in WSU-HN6 and CAL-27 cells, then cultured the cell suspension for 24 h. Flow cytometry was used to assess the proportion of cells undergoing anoikis. The proportion of apoptotic cells was increased significantly in the BIRC5-knockdown group compared with that in the si-NC group (Figs. 8C and 8D). Combined with previous results, the overexpression of ARGs in HNSCC tissues and cells can significantly promote anoikis resistance in tumor cells.
Figure 8: Expression of ARGs in tissues and cell lines and its effect on anoikis. (A) Expression of CDKN2A, BIRC5, and PLAU in three pairs of matched HNSCC (T) and paracancerous normal tissues (N). (B) Expression of CDKN2A, BIRC5, and PLAU in the HOK, WSU-HN6, and CAL-27 cell lines. (C, D) Anoikis of WSU-HN6 and CAL-27 cells and apoptotic cell proportions in each group. The apoptotic cells shown in the graph are the proportion of early anoikis + late anoikis. *p < 0.05, **p < 0.01, ***p < 0.001, compared with si-NC group; n = 3.
Radicicol can stably bind to ARGs and inhibit their expression level
The lower the binding energy between the ligand and the receptor, the more stable it is. Therefore, a binding energy ≤ –5.0 kcal/mol was chosen as the screening condition. In this study, the binding energies of the three ARGs and radicicol were much lower than the set conditions (the binding energies of CDKN2A, BIRC5, and PLAU with radicicol were –7.0, –7.0, and –7.8 kcal/mol, respectively), indicating a good binding effect between radicicol and each ARG (Fig. 9A). We measured the expression levels of CDKN2A, BIRC5, and PLAU to dissect the relationship between radicicol and the three ARGs. RT–PCR showed that the relative mRNA expression levels of CDKN2A, BIRC5, and PLAU were decreased after radicicol treatment (Figs. 9B and 9C). Therefore, radicicol can regulate the mRNA expression level of ARGs, suggesting that radicicol may target the ARGs in the prognostic model.
Figure 9: Radicicol may target the ARGs in the prognostic model. (A) Molecular docking diagrams of radicicol with CDKN2A, BIRC5, and PLAU. (B, C) Expression of CDKN2A, BIRC5, and PLAU in the WSU-HN6 and CAL-27 cell lines after coculture with radicicol. *p < 0.05, **p < 0.01, ***p < 0.001, compared with 0 μM; n = 3.
Radicicol inhibited the survival rate of HNSCC cells
In the present study, we explored the relationship between the radicicol concentration and the survival rate of HNSCC cells. Three different concentrations (5, 10, and 20 μM) were used to coculture two HNSCC cell lines (WSU-HN6 and CAL-27) for 24, 48, and 72 h. The OD of each well was measured, and the cell viability was subsequently calculated. The results showed that the cell survival rate of both cell lines was significantly reduced with the three different concentrations of radicicol compared with the survival rate in the control group (p < 0.05), and radicicol inhibited cells survival rate in a dose- and time-dependent manner (Figs. 10A and 10B). A comparison of the two cell lines revealed that radicicol was more effective in inhibiting the survival rate of CAL-27 cells than WSU-HN6 cells (the mean cell viability rate at each concentration and time point are shown below, and all results are expressed as WSU-HN6 vs. CAL-27. 5 μM, 24 h: 79.38% vs. 67.63%; 10 μM, 24 h: 69.92% vs. 55.20%; 20 μM, 24 h: 69.82% vs. 28.03%; 5 μM, 48 h: 48.95% vs. 23.93%; 10 μM, 48 h: 39.53% vs. 21.28%; 20 μM, 48 h: 27.66% vs. 8.59%; 5 μM, 72 h: 37.20% vs. 7.25%; 10 μM, 72 h: 29.27% vs. 5.29%; 20 μM, 72 h: 20.04% vs. 7.13%). Moreover, the number of colonies in the three radicicol groups was reduced compared with that of the control group (Fig. 10C).
Figure 10: Radicicol inhibited survival rate of WSU-HN6 and CAL-27 cells. (A, B) Survival rate of WSU-HN6 and CAL-27 cells after coculture for 24, 48, and 72 h with radicicol. (C) Plate clone assay to assess the colony formation ability of two cell lines under different concentrations of radicicol. *p < 0.05, **p < 0.01, ***p < 0.001, compared with 0 μM; n = 3.
Radicicol can regulate the cell cycle and promote anoikis
Since cell survival rate was inhibited in the radicicol groups, we hypothesized that one or more cell cycle phases might be blocked during this process. Flow cytometry analysis was performed to test this hypothesis. The radicicol groups had a significantly higher proportion of cells in the G2/M phase and a significantly lower proportion in the G1 and S phases than that in the control group (p < 0.001) (Figs. 11A and 11B), indicating that the cell cycle was blocked in the G2/M phase.
Figure 11: Radicicol can block G2/M phase and promote anoikis. (A, B) Distribution and statistics of the cell cycle phases of WSU-HN6 and CAL-27 cells in each group. (C, D) Anoikis of WSU-HN6 and CAL-27 cells and apoptotic cell proportions in each group. The apoptotic cells shown in the graph are the proportion of early anoikis + late anoikis. *, #, & p < 0.05, **, ##, && p < 0.01, ***, ###, &&& p < 0.001. * indicates G1 phase or apoptotic cells compared to the radicicol groups at 0 μM, # indicates S phase compared to the radicicol groups at 0 μM; & indicates G2/M phase compared to the radicicol groups at 0 μM; n = 3.
As previously mentioned, HNSCC cells were cultured on nonadherent panels to investigate the effect of radicicol on anoikis. Radicicol was cocultured with suspension culture cells for 24 h, and the cells were stained using Annexin V-FITC/PI to analyze the level of anoikis through the intensity of the cell fluorescence signal. Annexin V-FITC-positive and PI-negative cells represented early anoikis, while Annexin V-FITC-positive and PI-positive cells represented late anoikis. The results showed that the percentage of anoikis cells (percentage of early + late anoikis) was significantly increased in the radicicol groups vs. the control group (Figs. 11C and 11D). A dose-dependent effect was demonstrated in all of the above experiments, and the effect of radicicol was more pronounced in CAL-27 cells. These results confirm that radicicol can block the G2/M phase and promote anoikis.
The prognosis of HNSCC depends on various factors, including age, lifestyle habits, and treatment, with metastases predominating the prognosis of HNSCC [16]. Several models currently use tumor metastasis to predict the prognosis of HNSCC. TNM staging is currently an accepted model for predicting prognosis; however, N staging only examines the number and size of positive lymph nodes, which are affected by the type of neck lymphatic dissection and the total number of lymph nodes removed [17,18]. The lymph node ratio (LNR) can be a valid predictor of prognosis in HNSCC [19]; however, the LNR cannot be used to assess patient prognosis when the number of positive lymph nodes is zero. Therefore, the creation of a prognostic discriminating model for patients with HNSCC based on tumor metastasis capacity is critical for post-operative patient observation and directing clinical medication use.
Anoikis resistance is a crucial molecular mechanism for survival during the metastatic cascade of tumor cells and is one of the hallmarks of tumorigenesis in EMT and a signature trait of tumor stem cells [20]. The development of anoikis resistance leads to an increased potential for tumor cell metastasis, an expansion of cancer stem cell subpopulations, chemoresistance, and a higher likelihood of recurrence, all of which are significantly related to a poor prognosis in HNSCC [21]. ARG-based predictive models play a significant role in determining the prognosis of many malignancies [22–24]; thus, anoikis resistance has major therapeutic implications. In this study, we used bioinformatics analysis to build an ARG prognostic model for HNSCC to predict patient prognosis and immune activity.
CDKN2A, BIRC5, and PLAU were found to be closely related to patient prognosis, consistent with the results of previous studies. CDKN2A was first identified by Kamb and can encode two proteins, namely, p16INK4a and p14ARF, thus exerting cell cycle regulation through multiple pathways [25]. CDKN2A gene expression abnormalities, primarily deletions, mutations, and abnormal hypermethylation have now been reported in a range of malignancies, including HNSCC [26]. Patients with CDKN2A deletion in HNSCC have a generally poor prognosis and are considerably more likely to experience HNSCC recurrence [27,28]. And targeting CDKN2A and/or the PI3K-AKT-mTOR pathway may be a valuable direction to develop precise therapy for HNSCC [29]. BIRC5 was first isolated and identified in a human gene bank screen by Ambrosini in 1997 and is believed to be the smallest member of the apoptosis suppressor protein family [30]. Moreover, studies have shown that abnormal expression of BIRC5 can be used as a diagnostic marker in HNSCC patients [31] and that BIRC5 is an important predictor of poor prognosis [32]. The PLAU gene is located on human chromosome 10q22.2 and encodes urokinase-type plasminogen activator [33]. PLAU has been shown to be closely related to tumor diagnosis and patient prognosis [34]. Chen demonstrated PLAU may function as an oncogene in HNSCC and regulate the EMT signaling pathway in vitro and in vivo [35]. Li demonstrated that PI3K-Akt pathway might underly the mechanism of PLAU’s oncogene role in HNSCC [36]. The elevation of CDKN2A, BIRC5, and PLAU expression seen in our tissue samples was consistent with prior studies; however, more clinical samples are needed to determine the link between these three genes’ expression levels and metastasis. In addition, although CDKN2A, BIRC5, and PLAU are associated with the prognosis of HNSCC, their effects on anoikis have not been experimentally verified. We selected BIRC5 to transfect siRNA in vitro to detect its effect on anoikis. According to the flow cytometry results, the inhibition of BIRC5 expression increased tumor cell apoptosis and decreased HNSCC anoikis resistance.
According to current studies, the tumor microenvironment (TME) provides a permissive environment for tumor progression and metastasis [37]. Therefore, considering that the TME can regulate anoikis resistance, we conducted ssGSEA to explore the immune status of high- and low-risk groups. The immune cells (B cells, CD8+ T cells, iDCs, macrophages, mast cells, NK cells, pDCs, T helper cells, Tfh, Th2, and TIL) and immune roles (checkpoint, cytolytic activity, HIL, inflammation-promoting, T cell co-inhibition, T cell co-stimulation, and type I (or II) IFN response) were more active in the low-risk group. These results imply that we can use the risk scores of ARGs to effectively distinguish the immune infiltration status of HNSCC and develop personalized immunotherapy. As for drug sensitivity, the low-risk group was more sensitive to multiple inhibitors of the PI3K/AKT/mTOR pathway, such as OSI-027, which not only enhanced immunotherapeutic effects [38] but also blocked the progression of HNSCC [39]. One study has found that PI3K/AKT/mTOR axis is highly activated in HNSCC, which is related to the proliferation, migration, invasion, and other biological behaviors of tumor cells [29]. As a result, our study can guide clinical treatment and provide evidence for improving future immunotherapy and seeking appropriate target populations.
In this study, differentially expressed ARGs were divided into up-regulated and down-regulated groups, with a view to using the database to develop effective drugs against anoikis. The radicicol ranks first among the obtained small molecule drugs. Radicicol, a macrolide antibiotic isolated from Monosporium bonorden by Delmotte et al. [40], is still in its infancy as a novel Hsp90 inhibitor. Since Hsp90 has regulatory effects on a variety of substrate proteins, inhibition of Hsp90 can regulate many signaling pathways, thereby inhibiting tumor proliferation, metastasis, and other processes [41], which has made clinically feasible Hsp90 small-molecule inhibitors a research focus. Interestingly, in the previous drug sensitivity analyses, we also found an Hsp90 inhibitor had a lower IC50 in the high-risk group, indicating that Hsp90 inhibitor has great potential to become a novel drug targeting ARGs prognostic model. However, radicicol was discovered only a short time ago and has the disadvantage of low activity in vivo; thus, it has not yet entered the clinical research stage, especially for HNSCC. A recent study demonstrated that radicicol promoted anoikis in glioblastoma by causing endoplasmic reticulum stress and preventing AKT/mTOR/p3S3K phosphorylation activation, thus confirming that radicicol was closely associated with PI3K/AKT/mTOR and anoikis [42]. In addition, we demonstrated in vitro that radicicol modulated mRNA expression levels of the three ARGs included in the prognostic model, and that radicicol inhibited HNSCC cells survival rate and promoted anoikis. In summary, combined with the studies of scholars, we hypothesized that radicicol may promote the anoikis of HNSCC by inhibiting the expressions of CDKN2A, BIRC5, and PLAU, thereby blocking the PI3K/AKT/mTOR signaling pathway. However, the specific molecular mechanism still needs to be confirmed by subsequent experiments.
Our study provides a new perspective for exploring the role of ARGs in the development and metastasis of HNSCC. We constructed a prognostic model, identified novel drug that promotes anoikis, and validated the results of the database analysis in vitro. However, it must be acknowledged that there are still some limitations in this study. The model was derived from a public database analysis; thus, its applicability and accuracy need to be further explored in clinical HNSCC patients. The effect of radicicol on anoikis in vivo and its molecular mechanisms still need to be further explored. In conclusion, the ARG prognostic model is expected to be a novel biomarker for HNSCC diagnosis and treatment decisions, and ARGs can be considered drug development targets for reducing HNSCC metastasis.
Acknowledgement: The authors would like to thank the TCGA and GEO database for the availability of the data.
Funding Statement: This work was supported by the National Nature Science Foundation of China (Grant Numbers 81072214, 30371547) and the National Key R&D Program of China (Grant Number 2016YFC1102603).
Author Contributions: LQ, AT and XQ performed experiments; FL and CL guided experiments; LQ, XG and CL wrote the manuscript. All authors listed have made a substantial, direct, and intellectual contribution to the work and approved it for publication.
Availability of Data and Materials: All data used and analyzed in this study are available from the corresponding author on reasonable request.
Ethics Approval: The studies involving human participants were reviewed and approved by the Biomedical Ethics Committee of Peking University Stomatological Hospital. The ethical code is PKUSSIRB-202274058.
Conflicts of Interest: The authors declare that the research was conducted in the absence of any commercial or financial relationships that could be construed as a potential conflict of interest.
References
1. Sung, H., Ferlay, J., Siegel, R. L., Laversanne, M., Soerjomataram, I. et al. (2021). Global cancer statistics 2020: GLOBOCAN estimates of incidence and mortality Worldwide for 36 cancers in 185 Countries. CA-A Cancer Journal For Clinicians, 71(3), 209–249. https://doi.org/10.3322/caac.21660 [Google Scholar] [PubMed] [CrossRef]
2. Suh, Y., Amelio, I., Urbano, T. G., Tavassoli, M. (2014). Clinical update on cancer: Molecular oncology of head and neck cancer. Cell Death & Disease, 5(1), e1018-29. https://doi.org/10.1038/cddis.2013.548 [Google Scholar] [PubMed] [CrossRef]
3. Leemans, C. R., Braakhuis, B. J. M., Brakenhoff, R. H. (2011). The molecular biology of head and neck cancer. Nature Reviews Cancer, 11(1), 9–22. https://doi.org/10.1038/nrc2982 [Google Scholar] [PubMed] [CrossRef]
4. Chambers, A. F., Groom, A. C., MacDonald, I. C. (2002). Dissemination and growth of cancer cells in metastatic sites. Nature Reviews Cancer, 2(8), 563–572. https://doi.org/10.1038/nrc865 [Google Scholar] [PubMed] [CrossRef]
5. Leemans, C. R., Tiwari, R., Msc, J., Isaa van Der Waal, D. D. S., Snow, G. B. (1994). Recurrence at the primary site in head and neck cancer and the significance of neck lymph node metastases as a prognostic factor. Cancer, 73(1), 187–190. https://doi.org/10.1002/(ISSN)1097-0142 [Google Scholar] [CrossRef]
6. Cerezo, L., Millan, I., Torre, A., Aragon, G., Otero, J. (1992). Prognostic factors for survival and tumor control in cervical lymph node metastases from head and neck cancer: A multivariate study of 492 cases. Cancer, 69(5), 1224–1234. https://doi.org/10.1002/cncr.2820690526 [Google Scholar] [PubMed] [CrossRef]
7. Frisch, S. M., Francis, H. (1994). Disruption of epithelial cell-matrix interactions induces apoptosis. Journal of Cell Biology, 124(4), 619–626. https://doi.org/10.1083/jcb.124.4.619 [Google Scholar] [PubMed] [CrossRef]
8. Tang, D. L., Kang, R., Berghe, T., Vandenabeele, V., Kroemer, P. et al. (2019). The molecular machinery of regulated cell death. Cell Research, 29(5), 347–364. https://doi.org/10.1038/s41422-019-0164-5 [Google Scholar] [PubMed] [CrossRef]
9. Xie, Y., Hou, W., Song, X., Yu, Y., Huang, J. et al. (2016). Ferroptosis: Process and function. Cell Death Differentiation, 23(3), 369–379. https://doi.org/10.1038/cdd.2015.158 [Google Scholar] [PubMed] [CrossRef]
10. Tan, K., Goldstein, D., Crowe, P., Yang, J. L. (2013). Uncovering a key to the process of metastasis in human cancers: A review of critical regulators of anoikis. Journal of Cancer Research and Clinical Oncology, 139(11), 1795–1805. https://doi.org/10.1007/s00432-013-1482-5 [Google Scholar] [PubMed] [CrossRef]
11. Yang, J., Zheng, Z. C., Yan, X. J., Li, X., Liu, Z. et al. (2013). Integration of autophagy and anoikis resistance in solid tumors. Anatomical Record-Advances in Integrative Anatomy and Evolutionary Biology, 296(10), 1501–1508. https://doi.org/10.1002/ar.22769 [Google Scholar] [PubMed] [CrossRef]
12. Swan, E. A., Jasser, S. A., Holsinger, F. C., Doan, D., Bucana, C. et al. (2003). Acquisition of anoikis resistance is a critical step in the progression of oral tongue cancer. Oral Oncology, 39(7), 648–655. https://doi.org/10.1016/S1368-8375(03)00049-6 [Google Scholar] [PubMed] [CrossRef]
13. Taddei, M. L., Giannoni, E., Fiaschi, T., Chiarugi, P. (2012). Anoikis: An emerging hallmark in health and diseases. Journal of Pathology, 226(2), 380–393. https://doi.org/10.1002/path.3000 [Google Scholar] [PubMed] [CrossRef]
14. Guo, C., Xu, L. F., Li, H. M., Wang, W., Guo, J. H. et al. (2019). Transcriptomic study of the mechanism of anoikis resistance in head and neck squamous carcinoma. PeerJ, 7(5548), e6978. https://doi.org/10.7717/peerj.6978 [Google Scholar] [PubMed] [CrossRef]
15. Young, L., Sung, J. L., Stacey, G., Masterset, J. R. (2010). Detection of mycoplasma in cell cultures. Nature Protocols, 5(5), 929–934. https://doi.org/10.1038/nprot.2010.43 [Google Scholar] [PubMed] [CrossRef]
16. Caudell, J. J., Gillison, M. L., Maghami, E., Spencer, S., Pfister, D. G. et al. (2022). NCCN Guidelines® insights: Head and neck cancers, version 1.2022. Journal of the National Comprehensive Cancer Network, 20(3), 224–234. https://doi.org/10.6004/jnccn.2022.0016 [Google Scholar] [PubMed] [CrossRef]
17. Jan, J. C., Hsu, W. H., Liu, S. A., Wong, Y. K., Poon, C. K. et al. (2011). Prognostic factors in patients with buccal squamous cell carcinoma: 10-year experience. Journal of Oral and Maxillofacial Surgery, 69(2), 396–404. https://doi.org/10.1016/j.joms.2010.05.017 [Google Scholar] [PubMed] [CrossRef]
18. Shah, J. P., Gil, Z. (2009). Current concepts in management of oral cancer—Surgery. Oral Oncology, 45(4–5), 394–401. https://doi.org/10.1016/j.oraloncology.2008.05.017 [Google Scholar] [PubMed] [CrossRef]
19. Huang, T. H., Li, K. Y., Choi, W. S. (2019). Lymph node ratio as prognostic variable in oral squamous cell carcinomas: Systematic review and meta-analysis. Oral Oncology, 89(8), 133–143. https://doi.org/10.1016/j.oraloncology.2018.12.032 [Google Scholar] [PubMed] [CrossRef]
20. Frisch, S. M., Schaller, M., Cieply, B. (2013). Mechanisms that link the oncogenic epithelial-mesenchymal transition to suppression of anoikis. Journal of Cell Science, 126(1), 21–29. https://doi.org/10.1242/jcs.120907 [Google Scholar] [PubMed] [CrossRef]
21. Johnson, D., Burtness, E., Leemans, B., Lui, R., W., V. et al. (2020). Head and neck squamous cell carcinoma. Nature Reviews Disease Primers, 6(1), 92. https://doi.org/10.1038/s41572-020-00224-3 [Google Scholar] [PubMed] [CrossRef]
22. Jin, L. T., Chun, J., Pan, C. Y., Kumar, A., Zhang, G. J. et al. (2018). The PLAG1-GDH1 Axis promotes anoikis resistance and tumor metastasis through CamKK2-AMPK signaling in LKB1-deficient lung cancer. Molecular Cell, 69(1), 87–99. https://doi.org/10.1016/j.molcel.2017.11.025 [Google Scholar] [PubMed] [CrossRef]
23. Wang, Y. N., Zeng, Z. L., Lu, J. H., Wang, Y., Liu, Z. X. et al. (2018). CPT1A-mediated fatty acid oxidation promotes colorectal cancer cell metastasis by inhibiting anoikis. Oncogene, 37(46), 6025–6040. https://doi.org/10.1038/s41388-018-0384-z [Google Scholar] [PubMed] [CrossRef]
24. Chen, S., Gu, J. M., Zhang, Q. F., Hu, Y., Ge, Y. (2021). Development of biomarker signatures associated with anoikis to predict prognosis in endometrial carcinoma patients. Journal of Oncology, 2021(2), 3375297. https://doi.org/10.1155/2021/3375297 [Google Scholar] [PubMed] [CrossRef]
25. Kamb, A., Gruis, N. A., Weaver-Feldhaus, J., Liu, Q., Harshman, K. et al. (1994). A cell cycle regulator potentially involved in genesis of many tumor types. Science, 264(5157), 436–440. https://doi.org/10.1126/science.8153634 [Google Scholar] [PubMed] [CrossRef]
26. Pineau, P., Marchio, A., Cordina, E., Tiollais, P., Dejean, A. (2003). Homozygous deletions scanning in tumor cell lines detects previously unsuspected loci. International Journal of Cancer, 106(2), 216–223. https://doi.org/10.1002/(ISSN)1097-0215 [Google Scholar] [CrossRef]
27. Padhi, S. S., Roy, S., Kar, M., Saha, A., Roy, S. et al. (2017). Role of CDKN2A/p16 expression in the prognostication of oral squamous cell carcinoma. Oral Oncology, 73, 27–35. https://doi.org/10.1016/j.oraloncology.2017.07.030 [Google Scholar] [PubMed] [CrossRef]
28. Roshan, A. G. D., Sinto, M. S., Vargees, B. T., Kannan, S. (2019). Loss of CDKN2A and CDKN2B expression is associated with disease recurrence in oral cancer. Journal of Oral and Maxillofacial Pathology, 23(1), 82–89. https://doi.org/10.4103/jomfp.JOMFP_184_18 [Google Scholar] [PubMed] [CrossRef]
29. Schwaederle, M., Elkin, S. S., Tomson, B., Carter, N., L., J. et al. (2015). Squamousness: Next-generation sequencing reveals shared molecular features across squamous tumor types. Cell Cycle, 14(14), 2355–2361. https://doi.org/10.1080/15384101.2015.1053669 [Google Scholar] [PubMed] [CrossRef]
30. Ambrosini, G., Adida, C., Altieri, D. C. (1997). A novel anti-apoptosis gene, survivin, expressed in incèr and lymphoma. Nature Medicine, 3(8), 917–921. https://doi.org/10.1038/nm0897-917 [Google Scholar] [PubMed] [CrossRef]
31. Kim, Y. H., Kim, S. M., Kim, Y. K., Hong, S. P., Kim, M. J. et al. (2010). Evaluation of survivin as a prognostic marker in oral squamous cell carcinoma. Journal of Oral Pathology & Medicine, 39(5), 368–375. [Google Scholar]
32. Santarelli, A., Mascitti, M., Russo, L. L., Sartini, D., Troiano, G. et al. (2018). Survivin-based treatment strategies for squamous cell carcinoma. International Journal of Molecular Sciences, 9(4), 971–994. https://doi.org/10.3390/ijms19040971 [Google Scholar] [PubMed] [CrossRef]
33. Mekkawy, A. H., Pourgholami, M. H., Morris, D. L. (2014). Involvement of urokinase-type plasminogen activator system in cancer: An overview. Medicinal Research Reviews, 34(5), 918–956. https://doi.org/10.1002/med.21308 [Google Scholar] [PubMed] [CrossRef]
34. Novak, C. M., Horst, E. N., Taylor, C. C., Liu, C. Z., Mehta, G. (2019). Fluid shear stress stimulates breast cancer cells to display invasive and chemoresistant phenotypes while upregulating PLAU in a 3D bioreactor. Biotechnology and Bioengineering, 116(11), 3084–3097. https://doi.org/10.1002/bit.27119 [Google Scholar] [PubMed] [CrossRef]
35. Chen, G. J., Sun, J. W., Xie, M. R., Yu, S. L., Tang, Q. M. et al. (2021). PLAU promotes cell proliferation and epithelial-mesenchymal transition in head and neck squamous cell carcinoma. Frontiers in Genetics, 12, 651882. https://doi.org/10.3389/fgene.2021.651882 [Google Scholar] [PubMed] [CrossRef]
36. Li, Z., Chen, X., H., C., Wang, J., Wei, C. et al. (2021). Overexpressed PLAU and its potential prognostic value in head and neck squamous cell carcinoma. PeerJ, 9(4), e10746. https://doi.org/10.7717/peerj.10746 [Google Scholar] [PubMed] [CrossRef]
37. Curry, J., Sprandio, M., Cognetti, J., Luginbuhl, D., Barad, A. et al. (2014). Tumor microenvironment in head and neck squamous cell carcinoma. Seminars in Oncology, 41(2), 217–234. https://doi.org/10.1053/j.seminoncol.2014.03.003 [Google Scholar] [PubMed] [CrossRef]
38. Wang, H., Chen, H., Liu, S., Zhang, J., Lu, H. (2021). Costimulation of γδTCR and TLR7/8 promotes Vδ2 T-cell antitumor activity by modulating mTOR pathway and APC function. Journal for ImmunoTherapy of Cancer, 9(12), e003339. https://doi.org/10.1136/jitc-2021-003339 [Google Scholar] [PubMed] [CrossRef]
39. Cassell, A., Freilino, M. L., Lee, J., Barr, S., Wang, L. et al. (2012). Targeting TORC1/2 enhances sensitivity to EGFR inhibitors in head and neck cancer preclinical models. Neoplasia, 14(11), 1005–1014. https://doi.org/10.1593/neo.121212 [Google Scholar] [PubMed] [CrossRef]
40. Delmotte, P., Delmotte-Plaque, J. (1953). A new antifungal substance of fungal origin. Nature, 171(4347), 344. https://doi.org/10.1038/171344a0 [Google Scholar] [PubMed] [CrossRef]
41. Jhaveri, K., Taldone, T., Modi, S., Chiosis, G. (2012). Advances in the clinical development of heat shock protein 90 (Hsp90) inhibitorsin cancers. Biochimica et Biophysica Acta, 2012(3), 742–755. https://doi.org/10.1016/j.bbamcr.2011.10.008 [Google Scholar] [PubMed] [CrossRef]
42. Li, Z., Zhang, Y., Chen, C., Chen, L., D., B. et al. (2017). Radicol, a novel trinorguaiane-type sesquiterpene, induces temozolomide-resistant glioma cell apoptosis via ER stress and Akt/mTOR pathway blockade. Phytotherapy Research, 31(5), 719–739. https://doi.org/10.1002/ptr.5793 [Google Scholar] [PubMed] [CrossRef]
Figure S1: All 41 chemicals with lower IC50 values in the low-risk group.
Figure S2: All 41 chemicals with lower IC50 values in the low-risk group.
Figure S3: Distribution of CDKN2A, BIRC5, and PLAU expression in different cell types using violin plot, respectively.
Cite This Article
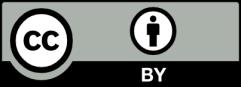