Open Access
REVIEW
The Interface of Cancer, Their Microenvironment and Nanotechnology
1 Instituto Estadual do Cérebro Paulo Niemeyer (IECPN)-Secretaria de Estado de Saúde, Rio de Janeiro, 20231-092, Brazil
2 Department of Chemistry, University College London, London, WC1H 0AJ, UK
3 NOVA Medical School-Faculdade de Ciências Médicas (NMS|FCM), Coimbra, 1169-056, Portugal
4 Fluminense Federal University, Campus do Gragoatá, Niterói, 24210-200, Brazil
5 Centogene GmbH. Am Strande 7, Rostock, PLZ 18055, Germany
* Corresponding Author: Tania Cristina Leite de Sampaio e Spohr. Email:
# Natalia Roque and Diana Matias contributed equally to this review
Oncologie 2022, 24(3), 371-411. https://doi.org/10.32604/oncologie.2022.024035
Received 22 May 2022; Accepted 19 August 2022; Issue published 19 September 2022
Abstract
Cancer is one of the deadliest diseases with a cure far from being found. Despite the extraordinary advances in the therapy approaches, only a few patients respond to treatments. The tumor microenvironment (TME) plays a crucial role in cancer progression by contributing to the chemoresistance. Thus, emerging efforts are being made in nanotechnology research focusing on nanoparticles’ potential role and their application in immune system modulation. Moreover, the omics have contributed to bioengineering and nanotechnology development by elucidating the mechanisms of cancer and specific biomarkers that could be used as new therapeutic targets. Furthermore, the non-coding microRNA as a target for cancer treatment and creation of organoids for the study of new treatments helped for the new therapeutics’ era called personalized medicine. Here we will discuss the role played by TME in tumor initiation and progression we will describe the recent nanotechnology applied to cancer treatment. Specifically, we will describe the potential role of nanoparticles (NPs) and their application in immune system modulation, ultimately leading to circumventing tumor cell proliferation.Keywords
Nomenclature
ACT | adoptive T-cell therapy |
ADCs | antibody–drug conjugates |
ALCL | anaplastic large-cell lymphoma |
AML | acute myeloid leukemia |
AnAc | anacardic acid |
APCs | antigen-presenting cells |
ATCT | genetic engineered T cells |
B2M | beta-2 microglobulin |
BA-TPQ | 7-(benzylamino)-3,4-dihydro-pyrrolo[4,3,2-de]quinolin-8(1H)-one |
BBB | blood-brain barrier |
BRCA1 | breast cancer 1 |
BRCA2 | breast cancer 2 |
CA125 | cancer antigen 125 |
CA19 | carbohydrate antigen 19 |
CAF | cancer-associated fibroblasts |
CAR-T | chimeric antigen receptor T cells |
CD226 | cluster of differentiation 226 |
CD28 | cluster of differentiation 28 |
CICs | cancer initiating cells |
CNS | central nervous system |
CRC | colorectal cancer |
CRISPR | clustered regularly interspaced short palindromic repeat |
CSF-1 | colony stimulating factor 1 |
CSF1R | colony-stimulating factor 1 receptor |
CTCs | circulating tumor cells |
ctDNA | circulating cell-free tumor DNA |
CTLA-4 | cytotoxic T-lymphocyte-associated protein 4 |
CCL2 | also known as MCP1 (monocyte chemoattractant protein 1) |
CCL22 | regulatory T cells chemoattractant |
CCL3 | also known as MIP-1-alpha (macrophage inflammatory protein 1-alpha) |
CCL4 | also known as MIP-1β (macrophage inflammatory protein-1β) |
CFS2 | also known as GM-CSF-Granulocyte-macrophage colony-stimulating factor |
CHILP | helper innate lymphoid precursor |
CILP | common ILC precursor |
CLP | common lymphoid progenitor |
CXCL10 | also known as IP-10 (Interferon gamma-induced protein 10) |
CXCL12 | also known as SDF1 (stromal cell-derived factor 1) |
CXCL8 | also known as IL-8 (Interleukin 8) |
CXCL9 | also known as MIG (monokine induced by gamma interferon) |
DCs | dendritic cells |
DDS | drug-delivery system |
ddPCR | droplet digital PCR |
DOX | doxorubicin |
EAC | esophageal adenocarcinoma |
ECM | extracellular matrix |
EGF | epidermal growth factor |
EGFR | epidermal growth factor receptor |
EGFR-TKIs | epidermal growth factor receptor tyrosine kinase inhibitor |
EPR | enhanced permeability and retention |
ERBB2 | receptor tyrosine-protein kinase erbB-2, also known as CD340 (cluster of differentiation 340) |
ESCC | esophageal squamous cell carcinoma |
FDA | US Food and Drug Administration |
FGF | fibroblast growth factor |
GATA3 | GATA-binding factor 3 |
GBM | glioblastoma |
GM-CSF | Granulocyte-macrophage colony-stimulating factor |
GPRC5A | retinoic acid-induced protein 3 |
HER2 | human epidermal growth factor receptor 2 |
hESCs | human embryonic stem cells |
HL | Hodgkin lymphoma |
HLA | human leukocytes antigen |
HNSCC | head and neck squamous cell carcinomas |
HPMA | N-(2-hydroxypropyl) methacrylamide |
HPV | human-papilloma viruses |
ICB | immune checkpoints blockade |
IECPN | Instituto Estadual do Cérebro Paulo Niemeyer |
IFN-γ | interferon gamma |
IL | interleukin |
ILCs | innate lymphoid cells |
IMMU-132 | sacituzumab govitecan |
IP-10 | interferon gamma-induced protein 10 |
iPSC | induced pluripotent stem cell |
ITGA5 | integrin alpha-5 |
mAb | monoclonal antibodies |
MCs | mast cells |
MDM2 | murine double minute2 |
MDS | myelodysplastic syndromes |
MDSCs | myeloid-derived suppressor cells |
MHC | major histocompatibility system complex |
MIG | monokine induced by gamma interferon |
miRNA | microRNA |
MLH1 | MutL homolog 1 |
MMAE | monomethyl auristatin |
MMP | matrix metallopeptidase |
MSH2 | DNA mismatch repair protein 2 |
MSH6 | DNA mismatch repair protein 6 |
NK | natural killer cells |
nm | nanometer |
NME2 | nucleoside diphosphate kinase B |
NO | nitric oxide |
NPs | nanoparticles |
NSCLC | non-small cell lung cancer |
OncomiR | oncogenic miRNAs |
PD-1 | programmed cell death protein 1 |
PD-L1 | programmed cell death protein ligand 1 |
PDGFRB | beta-type platelet-derived growth factor receptor |
PEG | poly (ethyleneglycol) |
PEG-S-DAGs | ceramides (PEG-Cer)18 or diacylglycerols |
PMAA | polymer redox-sensitive poly (methacrylic acid) |
PMS2 | mismatch repair endonuclease 2 |
PRAME | preferentially express antigen of melanoma |
PSA | prostate-specific antigen |
ROS | reactive oxygen species |
RT | radiotherapy |
scFV | single chain fragment variable |
SDF1 | stromal cell-derived factor 1 |
sgRNA | single-guide RNA |
SHH | Sonic hedgehog |
siRNA | small interfering RNA |
SNPs | single nucleotide polymorphisms |
SRM | selected reaction monitoring |
TALEN | transcription activator-like effector nucleases |
TAM | Tumor-associated macrophages |
TAMCs | tumor-associated mast cells |
TANs | tumor associated neutrophils |
TCGA | The Cancer Genome Atlas Research Network |
TCR-T cells | T cells expressing a tumor-targeting T-cell receptor |
TECs | tumor endothelial cells |
TFRC | transferrin receptor protein 1 |
TGFα | transforming growth factor alfa |
TGF-β | transforming growth factor beta |
TH | T helper |
TIGIT | T cell immunoreceptor with Ig and ITIM domains |
TILs | tumor-infiltrating lymphocytes |
TMA | Tissue Microarray Analysis |
TME | tumor microenvironment |
TNF-α | tumor necrosis factor alfa |
TP53 | tumor protein p53 |
TRAC | TCR alpha constant |
T-regs | regulatory T cells |
TSNs | thermosensitive nanoparticles |
uPA | Urokinase-type-plasminogen-activator |
UTR | untranslated region |
VEGF | vascular endothelial growth factor |
VEGF-A | vascular endothelial growth factor A |
WT1 | Wilms tumor 1 |
ZFDBDs | zinc finger DNA-binding domains |
ZFN | zinc-finger nucleases. |
Cancer is a highly aggressive and yet incomprehensible disease, widely distributed worldwide. Thus, many scientists have focused on unraveling the biological mechanisms involved in cancer cell systems, including the development of convenient and efficient biopharmaceuticals.
The TME, composed of stromal, immune cells, blood vessels, and the extracellular matrix, plays a pivotal role in tumor development, progression and metastasis [1]. Moreover, TME influences the drug resistance of tumor cells and, consequently, the patient outcome and recurrence [2]. Hence, efforts are being made to understand and characterize the TME. For instance, cell-type-specific markers have been used to identify ‘normal cells’ in the TME, such as cancer-associated fibroblasts (CAF), and immune-inflammatory cells, including Tumor-associated microglia/macrophages (TAM/M), lymphocytes and neutrophils [1]. All these TME components can manipulate signaling pathways and interactions between transformed and normal cells in an unlimited crosstalk [3]. Thus, many therapeutic strategies have emerged targeting TME. The inhibition of immune checkpoints (i.e., regulators of immune activation) is thought to be a promising therapeutic approach for treating some solid tumors, such as melanoma and lung cancer [4]. However, there is still an unmet need to explain why most cancer patients do not respond to this type of immunotherapy, whereas TME heterogeneity might be responsible [4]. It is already demonstrated that, the TME components have the capacity to alter and even to deregulate signaling pathways and interactions between transformed and normal cells in an uninterrupted cross-talk [5]. Taking this into account, currently inhibition of immune checkpoint (that are regulators of immune activation) pathways is thought to be a promising therapeutic approach for the treatment of some solid tumors, such as melanoma and lung cancer [4]. Nevertheless, most of the cancer patients do not respond to this type of immunotherapy, and the real reasons are still unknown, but the TME composition plays for sure a crucial role [6].
Nanotechnology has been used to overcome cancer treatment failure through drug delivery systems (DDS), including engineered nanoparticles (NPs) to precise target tumor cells [7]. Among all the advantages of using DSS, such as reducing the adverse effects of drugs in undesired cells/tissues, it allows the delivery of therapeutic compounds by passive and active targeting. Most solid tumors present leaky blood vessels or dysfunctional lymphatic drainage allowing the entrance of macromolecules and NPs with larger sizes being retained in tumors, named “Enhanced permeability and retention” (EPR) effect [8]. Passive targeting depends on the EPR effect since the carriers do not possess a specific tissue/cell ligand. EPR-based therapies intend to improve efficacy and sustain tolerability by modulating the biodistribution and pharmacokinetics of the drug [9]. On the other hand, the active targeting is based on the functionalization of NP surfaces with ligands (e.g., monoclonal antibodies mAb or peptides) to specifically recognize tumor receptors by improving their affinity and enhancing the drug delivery and penetration [9,10].
Besides nanotechnology, bioengineering is also applied in immunology. Immunotherapy using the adoptive T-cell transfer (ACT) is based on genetically engineered T cells for fighting cancer. This technology transforms T cells genetically by modulating their receptors and redirecting them for the destruction of specific tumor cells [11]. Different types of immunotherapies can be applied to cancer treatment involving T cells: tumor-infiltrating lymphocytes (TILs), T-cell receptor (TCR-T) T cells and chimeric antigen (CAR-T) cells [12–14]. Nevertheless, identifying specific antigens in tumor cells is crucial for successful immunotherapy using ACT approaches, working as potential therapeutic targets and new biomarkers in cancer, allowing the improvement of immunotherapy approaches and nanomedicine. Several biomolecules have already been discovered as cancer biomarkers, which include proteins, genetic material such as methylated DNAs, DNAs, microRNAs (miRNAs) and RNAs, lipids, oligosaccharides, and metabolites [15]. A vast amount of data identified miRNAs released by tumors into serum, urine, sputum, or other body fluids, which are essential for the diagnosis [16]. Biomarker ‘era’ is the ‘gold standard assay’ for prognostics and diagnostics of cancer, contributing to ‘personalized medicine’. According to each patient’s specific characteristics, tailoring the medical treatment by dividing individuals into subpopulations with distinct susceptibility to a particular disease or their response to a specific treatment is the basis of personalized medicine [17].
This review will exploit the TME role and how nanotechnology can be applied to modulate the immune system to overcome the therapeutic resistance in cancer patients by opening the doors for personalized medicine.
2 Tumor Microenvironment and Its Complexity-Implications in Cancer Progression
2.1 Tumor Microenvironment Crosstalk
Malignant changes in healthy cells are maintained and followed by altered stromal cells, extracellular matrix (ECM) components, immune cells, blood and lymphatic vessels, and CAF [5]. This dynamic and active crosstalk between the subset of cells that compose the TME allows the tumor to establish and proliferate, evading the anti-cancer therapies and the host immune surveillance [5]. Thus, TME can be a target for long-established treatments that enhance the therapeutic outcomes for cancer [18] and unraveling the dynamic crosstalk is a matter of importance. Many growth factors present in TME are secreted by different cells, including fibroblast growth factor (FGF) [19], Sonic hedgehog (SHH) [20], epidermal growth factor (EGF) [21], chemokines (such as CXCL12-also known as SDF1 (stromal cell-derived factor 1) and CCL22 (regulatory T cells chemoattractant) [22] and ECM (such as proteoglycans and glycoproteins) [23]. All of them are not only important for the survival and growth of the malignant cells but also act as a chemoattractant by recruiting other cells into the TME [24]. Indeed, tumor cells hijack endothelial cells to supply nutrients and oxygen and support their progression, providing a portal for tumor metastasis [25]. Endothelial cells secrete tumor stimulating factors, such as IL-6 (interleukin-6), CXCL8 (also known as IL-8), and EGF [2] to promote tumor resistance and invasiveness. On the other hand, CAFs secret high levels of CXCL15 to induce the expression of programmed cell death protein ligand 1 (PD-L1) in tumors creating the immunosuppressive TME [26] (Scheme 1).
Scheme 1: Tumor environment crosstalk (Scheme done by Spohr, TCLS)
The different responses to the treatments and distinct prognoses in cancer patients are due to the distinct types of immune cells present in TME. The innate lymphoid cells (ILCs) are a group of lymphoid cells that do not perform the rearrangement of their antigen receptors [27]. The ILCs are divided into three groups according to the type of cytokine production and the expression of transcription factor. ILC1 expresses the transcriptional factor T-bet and the cytokines IFN-g and TNF; the ILC2 expresses GATA-binding factor 3 (GATA3) and produces the cytokines IL-4, IL-5, IL-9 and, while IL-13 and ILC3 express the retinoic acid receptor-related orphan receptor-γt (RORγt) and the cytokines IL-17A and IL-22 [28]. As part of the innate and adaptive immune response, ILCs can either contribute to tumor regression by inducing T cell priming, for instance, or stimulate tumor evasion by releasing immunosuppressive molecules [29].
Moreover, regulatory T cells (T-regs) have an essential role in immune homeostasis and self-tolerance [30], being part of the adaptive immune response. In cancer, Tregs usually contribute to the development of an immune-suppressive TME by suppressing the anti-tumor immune response, and consequently promoting cancer progression and immune evasion [31]. The main immune-suppressive cytokines secreted by T-regs cells are TGF-β, IL-10, and IL-35 [30]. Nevertheless, in distinct types of cancers, (i.e., gastric and colorectal), T-regs play a protective role by controlling inflammation associated with the neoplastic transformation [32,33]. In this context, it seems reasonable to manipulate Treg numbers and functions to improve a patient’s prognosis [34]. Yet, this approach needs deep investigation since T-reg depletion is involved with autoimmune development.
Another type of lymphoid cell in TME is the gamma delta-T cells (gd-T cells) characterized by unique features resembling innate cells since they recognize conserved non-peptide antigens from stressed cells. They act as adaptive cells by undergoing clonal expansion and developing antigen-specific memory [35]. Differentially from the ILCs, gd-T cells are part of a lymphocyte group able to perform surface rearrangements [36], originating from double negative thymocytes (CD4-/CD8-) [37]. These cells have the peculiar characteristic of acting independently of MHC and migrate to peripheral tissues poor in T and B cells [38]. In TME, those cells are implicated in the early phase of the immune response, by secreting IL-17, CXCL8, CFS2, and transforming growth factor alpha (TGFα), which support the survival of myeloid-derived suppressor cells (MDSCs) and helps the new vessels formation and tumor growth [39]. Besides, gdT-cells can produce pro-inflammatory cytokines such as IFN-gamma, having an anti-tumoral profile [40].
Natural killer cells, the most studied population of ILCs (ILC 1), revealed great importance in anti-tumor immunization by producing TNF-α, IFN-g, perforin, and granzymes in response to IL-12, IL-15, and IL-18 [41]. The ILC3 group cells play a critical role in the inflammatory response and tumorigenesis [42,43]. Some studies demonstrated that ILC3 cells can have a dual role in tumor context [44–47]. On the one hand, ILC3 cells can play a pro-tumorigenic role by inducing angiogenesis, inhibiting apoptosis, and tumor evasion mainly by secreting IL-22. ILC3’s pro-tumorigenic role is dependent on IL-22/IL-22R-AKT signaling pathway inducing pancreatic cancer cell proliferation and invasion [48]. On the other hand, they can have an anti-tumorigenic role by activating and recruiting Tregs, NK cells, and neutrophils to the TME by secreting IFN-g and IL-17 [45,49]. Thus, the anti-tumor role of ILC3 cells depends on the recruitment of immune cells, such as CD8T cells, activated myeloid cells, NKT and NK cells [44]. For instance, the anti-tumor role of ILC3 cells depends on the release of IFN-g and TRAIL in hepatocellular carcinoma [46]. On the other hand, the pro or anti-tumor activities depend on the stage of development and the type of tumor, the complex network of calibrated incoming signals that controls cell-cell interactions in the microenvironment.
The immature myeloid cells (the precursors of the MDSCs and dendritic cells (DCs)) invade the TME and promote tumor immune evasion and angiogenesis [50]. Among all the roles played by MDSCs, in cancer, they are responsible for the switch off the immune response due to the secretion of several factors, such as transforming growth factor-beta (TGF-β), IL-10, and generation of nitric oxide (NO) and reactive oxygen species (ROS) [51]. Indeed, the circulating MDSC levels are correlated with the overall survival, clinical-stage, metastatic burden, vasculogenesis, and tumor evasion [52].
Mast cells (MCs) arise from bone marrow pluripotent stem cells CD34+ and CD117+. In 1878, Paul Ehrlich firstly described the infiltration of presence of MCs in human tumors, and it is well established that they infiltrate in hematological and solid tumors (such as stomach, thyroid, prostate, pancreas, breast cancer and melanoma), being termed tumor-associated mast cells (TAMCs) [53]. Their role of TAMCs in tumor progression is controversial, displaying antitumorigenic or pro-tumorigenic phenotypes as they can exert support tumor cells or inhibit function. For instance, antitumorigenic TAMCs were found in breast and ovarian cancer and the pro-tumorigenic phenotype in gastric and bladder cancer. In breast and ovarian cancer, TAMCs plays an antitumorigenic role, while in gastric and bladder cancer, they play a protumorigenic role [54,55]. TAMCs antitumorigenic behavior colonizes the tumor periphery by playing cytolytic activity against tumor cells [56]. On the other hand, TAMCs with a pro-tumorigenic TAMCs role infiltrate the tumor bulk, contributing to tumor cell proliferation, invasion, angiogenesis, and extravasation of several cytokine-producing cells [57,58].
Besides, tumor cells secrete factors that directly affecting the immune and non-immune stromal cells within TME. These tumor-induced immunological changes are essential for tumor establishment but are even more important for the cancer progression and metastasis [59]. Not only do monocytes and macrophages play crucial roles during tumor development and metastasis, but also neutrophils that are also very abundant innate immune cells in the peripheral blood., However, although the role of neutrophils is still controversial [39,60,61]. Some studies pointed to a pro-metastatic (N2) property of tumor-associated neutrophils (TANs) instead of an anti-metastatic (N1) function [60,62,63]. It was demonstrated that the TGF-β cytokine plays a crucial role in inducing the differentiation of N1-TANs into N2-TANs [64,65]. The pro-metastatic and proangiogenic role of TANs is mainly due to its capacity to secrete several proangiogenic or immunosuppressive molecules, such as VEGF, IL-1β, TGFα, FGF2; enzymes involved in ECM remodeling such as MMP9 and several chemokines (i.e., CXCL1, CCL3, CCL4, CXCL8, CXCL9 and CXCL10) [66]. In obese patients diagnosed with kidney cancer, the increased expression of arginase-1 was involved in T cell suppression [67]. Several evidence showed the presence of inflammatory CD66b+ neutrophils in both TME and peripheral blood in cancer patients and correlate their presence with poor clinical outcome [68,69].
However, the most abundant immune cells in tumors are TAMs [70] and their infiltration is associated with clinical stage, overall survival and recurrence-free survival in different cancers, such as gastric, breast, ovarian, oral, bladder, brain, when a high infiltration of TAMs is associated with a better survival [71–74]. According to the classical classification, TAMs usually observed in tumors display an ‘M2-like’ phenotype, a suppressive behavior promoting tumor progression angiogenesis [6]. However, this oversimplified and widely used nomenclature used, proposed 20 years ago, now refers the “M2-like” phenotype as the cells that have anti-inflammatory characteristics, which produce TGFβ and are activated by IL-13 or IL-4. On the other hand, “M1-like” macrophages are pro-inflammatory and activated by IFN-g, TNFa and Toll-like receptor (TLR) ligands [75,76]. For many years, it was thought that the brain was immune privileged only with the presence of specific immune cells called microglial cells, but recently it was shown that was not entirely true [77,78].
Recently, studies indicated a strong link between both tumor phenotype and genotype, and TME composition, which directly influence the secretion of cytokines (such as IL-6, IL-10 and vascular endothelial growth factor (VEGF)), chemokines (such as CCL4 and CCL2) and humoral factors of the immune system [59]. These recruited immune cells infiltrate into the tumor bulk and secrete cytokines that act paracrinaly into the tumor cells influencing their phenotype and sooner or later their metastasis. TAMs are directly involved in this tumorigenic reprogramming by secreting high levels of colony-stimulating factor 1 (CSF-1), a potent chemoattractant, differentiation and survival factor for macrophages and monocytes [79,80]. Moreover, TAMs potentiate the secretion of protumoral survival factors, proteases and angiogenic growth factors, including VEGF-A, matrix metallopeptidase 9 (MMP-9), EGF and Urokinase-type-plasminogen-activator (uPA) under stimulation of interleukin-4 (IL-4) and IL-13 secreted by T helper 2–polarized CD4+ T cells [81,82].
Moreover, the blood-brain barrier (BBB) may be damaged due to tumor growth which allows the entrance of macrophages and T-cells to the TME [83]. Müller and colleagues showed that this concept is controversial through the analysis of scRNA-seq data of human gliomas. Recently it was demonstrated that in human glioblastomas if there is a higher amount of microglia compared to TAMs, it is related to a better patient prognostic and survival [84], where blood-derived TAMs (macrophages) co-express ‘M1 and M2-like’ phenotypes in human gliomas [85]. This is also present in other cancers such as lung cancer, where 25% of patients present an M2-like TAM phenotype co-expressed with M1-like signatures [86]. On the other hand, it was also recently demonstrated, that high high-grade glioma-associated microglia (HGG-AM) plays an important role in adult glioblastoma progression, by secreting TGF-β1, and exhibiting proliferation and pro-inflammation signatures [87].
Regarding TME complexity and its importance for tumorigenesis, it is crucial to bear in mind that cancer type, stage and location can influence the unique immune scenery and TME of each cancer.
2.2 Tumor Microenvironment as a New Player against Cancer
The evolution of immunotherapies focused on immune checkpoint blockade (ICB) has revolutionized cancer treatments. However, we are still far from having a successful treatment for most cancers. This concept is based on the blockade of immune-suppressive checkpoints, namely T cell immunoreceptor with Ig and ITIM domains (TIGIT), PD-L1, and cytotoxic T-lymphocyte-associated protein 4 (CTLA-4). On the other hand, it has also been used to activate immune-stimulatory checkpoints, particularly CD28 and CD226 on some effector cells, such as T cells and NK cells [88–90]. Unfortunately, the clinical success of these immunotherapies was quite surprising but disappointing in some cases. In cancer patients with equal diagnosis, different responses to the ICB therapy were observed, raising the importance of the TME role in modulating immune response against tumors [59]. In melanoma, the gut microbiome plays a pivotal role in ICB therapy. Differences in the composition and variety of the patients’ gut microbiome of ICB responders vs. non-responders showed an unquestionably higher relative abundance of specific bacteria, named Ruminococcaceae family, which is correlated with the presence of CD8+ T cells in the TME in responding melanoma patients [91]. Therefore, a deeper analysis of the TME complexity during the tumor diagnosis is crucial to improve the outcome of ICB and develop next-generation immunotherapies. Indeed, some ICBs are in clinical use for several types of cancers (see Table 1).
TAMs have been suggested as the main target in the therapeutic field by recurring to the immunotherapies using antagonists of checkpoints [6]. Thus, reducing the number of TAMs using blocking colony-stimulating factor 1 receptor (CSF1R), which is crucial for the recruitment, differentiation, and survival of TAMs impairs tumor development and progression.
Moreover, the establishment and progression of primary tumors are directly correlated with the global proportion and phenotype of T cells within the TME [92]. In an early phase of the tumoral process, antitumor T cells cannot govern tumor growth due to the tumor-induced tolerance mechanisms (state of unresponsiveness of the immune system), which induces T cell exhaustion, reversible or irreversible [92]. Studies demonstrated crosstalk between immunosuppressive TME and T cell exhaustion, due to weak interactions of DCs present in the TME [59]. The irreversible T cell exhaustion leads to a non-response to ICB-like anti-PD-1/anti-PD-L1 therapy [93], provoked by the arrangement of chronic exposure to tumor antigen and to immunosuppressive cytokines and different cell types [59].
Among all anti-cancer therapies developed to target the immune or non-immune cells, it is important to consider the quantity, quality, and localization of these cells on TME. Unfortunately, most patients did not present a lasting response to ICB or other immunotherapies; indeed, some do not respond [39]. The heterogeneity of the tumor mass is highly relevant to multiple aspects of immunotherapy efficacy. Besides, most cancer treatments induce mutations and alterations in tumor cells, contributing to their heterogeneity [94]. Thus, there is an enormous necessity to discover new strategies to target the immune system with high efficiency and consequently increase the cancer therapies’ success.
3 Engineered Technologies for Specific Therapy in Cancer
3.1 Adoptive T-Cell Therapy (ACT): CAR-T Cells (Chimeric Antigen Receptor) and TCR-T Cells (T Cells Expressing a Tumor-Targeting T-Cell Receptor)
The fields of genetically engineered T cells is one of the most hopeful applications in the battle against cancer. The manipulation of T-cells in ex vivo and introducing them back to patients to fight the disease is closer to reality than ever. Adoptive T-cell therapy (ACT) is based on the genetic alteration of T cells by modulating their receptors and redirecting them towards specific tumor-associated antigens (TAAs), and consequently killing the tumor cells [11,95].
Two different immunotherapies have been developed for cancer treatment involving T cells: the genetically modified T cell receptor (TCR-T cells) or the chimeric antigen receptor, named CAR-T cells, the only ACT-approved FDA immunotherapy (Fig. 1) [11,95]. Moreover, genetic engineering methods such as ZFN (zinc-finger nucleases), TALEN (transcription activator-like effector nucleases), and CRISPR (clustered regularly interspaced short palindromic repeat)/Cas9 (CRISPR associated nuclease) can be used to modulate TCRs, for instance identifying specific TAAs in tumor cells could be the first step for the success of immunotherapy using TCR-T [96]. However, for efficient activation of T cells antigenic peptides must be presented by the major histocompatibility system complex (MHCI or MHCII) and, consequently, their docking to TCR [11,96].
Figure 1: Adoptive T-cell therapy (ACT): TCR-T and CAR-T cells. Adoptive T-cell therapy (ACT) is immunotherapy applied to cancer treatment involving T cells that are genetically modified, such as T cell receptors (TCR-T cells) or the chimeric antigen receptor, named CAR-T cells. The T-cells from the patients are isolated and cultured through leukapheresis. Then, the T-cells presenting TCR (endogenous TCR) are activated using artificial dendritic cells (antibodies-coated beads). After their activation, the T-cells are genetically manipulated (inactivation of the endogenous TCR) using different mechanisms. Currently, the genetic modulation of T-cells involves the transcription activator-like effector nucleases (TALENS), Zinc-finger nucleases, and clustered regularly interspaced short palindromic repeat (CRISPR)/Cas9 (CRISPR associated nuclease) system to introduce or delete (in the cases of eliminating inhibitory receptors at T-cell surface) a specific sequence. These approaches contribute to T-cell receptor recognition mechanisms by a specific target neoantigen. After cloning the genetically modified T-cells, they are replenished in the patient (Figure done by Matias, D.)
Currently, gene edition machinery allows efficient and relatively simple gene insertion, modification and deletion, to generate ‘universal T cells’ or T cells resistant to exhaustion or to reduce the autoreactivity of redirected T cells [97]. The gene-editing of patient immune cells can be achieved also through the CRISPR/Cas9 system, considered the most flexible and efficient genome editing system and has been largely applied in several cell types and organisms. Unlike ZFNs and TALENs, which use protein domains, CRISPR-Cas9 technology uses a single-guide RNA (sgRNA) for site recognition, depending on simple base pairing between sgRNA and target DNA [98].
Although CRISPR/Cas9 technology has shown great potential for cancer immunotherapy, several challenges exist for its complete transformation into clinical treatment. For instance: 1) choose the optimal nuclease platform and rationally design the sgRNA; 2) decrease the potential off-target effects with enhanced CRISPR-Cas9 specificity, which might be achieved by increasing the specificity of nuclease-mediated cleavage of the target site and restricting the duration of nuclease expression; 3) edited cells must possess better proliferation capability or adaptability than the unedited cells. These obstacles may be partially overcome through in vitro genome editing, and then the edited cells could be reinfused into the patients after being expanded to the appropriate amount [99].
A phase 1 clinical trial reported that CRISPR-Cas9 gene editing (clinicaltrials.gov; trial NCT03399448) was used in three patients with advanced cancer, two with advanced refractory myeloma and one with metastatic sarcoma. The T lymphocytes were collected from patients and CRISPR-Cas9 was used to modify TRAC, TRBC and PDCD1 genes to improve the antitumor immunity. Moreover, a synthetic cancer-specific TCR transgene (NY-ESO-1) was introduced to target tumor cells specifically. Patient biopsies revealed a residual tumor and increased trafficking of T cells to the tumor sites in all three patients [100].
Therefore, ZFN, TALENs and CRISPR-Cas9 have been the main approaches used to genetically engineer T cells, CRISPR-Cas9 being the most exploited to date. Several obstacles must be solved for the using this approach in clinic patients, but the preclinical results and data from early clinical trials are promising.
However, one major concern for the development of CAR-T cells is their alloreactivity and consequently the risk of graft vs. host disease (GvHD) which can be solved by disrupting the expression of the TCR on CAR-T cells by targeting the TCR alpha constant domain (TRAC) [101]. The creation of TCR-negative universal T cells from a third-party donor could benefit patients who cannot obtain enough T cells for gene editing. Among all the factors, GvHD is the main responsible cause for TCR-negative universal T cells recognition of non-self HLA. Thus, eliminating HLA molecules from CAR-T cells by using gene-editing technologies has been suggested as an approach to avoid this rejection [102]. Ultimately, the clinical use of these “universal” CAR-T cells has been reached [101]. In T cell physiology, inhibitory signals induce T cell activation avoiding autoimmunity or uncontrolled inflammation. Increasing expression of these inhibitory molecules, such as PD-1 and CTLA-4 in T cells, allows immune evasion by tumor cells [103]. Thus, therapies that specifically block this inhibitory signal might prevent T cell immunosuppression in solid [23] and hematologic malignancies [104], such as genetic engineering (Fig. 1).
The first human clinical trial using TCR-T therapy was applied to patients with acute myeloid leukemia and myelodysplastic syndromes [105]. The TCR-T cells were prepared with a single-chain fragment variable (scFV) against the Wilms tumor 1 (WT1) antigen due to its high expression on the surface of many malignant cells and, consequently, its potential as a therapeutic target. The AML patients received the transplant of WT1 antigen-stem cell donor-derived T cell clones and displayed immune reactivity to WT1, which allowed the activation of immune response and, consequently, the destruction of the tumor cells [105].
The field of genetic engineering has progressed in the last decade. The role of TALEN editing in clinical trials has been mostly restricted to the creation of universal CAR-T cells. CAR-T cells triggered the attention of many researchers, clinicians, and companies as a successful tool for treating several cancers, specifically for ‘liquid tumors’ such as lymphomas and leukemia’s [11]. This is in contrast to the role of CRISPR-Cas9 editing, which has also been used in the generation of PD-1 knockout autologous lymphocytes for the treatment of different types of solid malignancies, such as non-small cell lung cancer (NCT02793856), renal cell carcinoma (NCT02867332), prostate cancer (NCT02867345), bladder cancer (NCT02863913), hepatocellular carcinoma (NCT04417764), gastrointestinal cancer (NCT04426669) [101] (clinicaltrials.gov accessed date 11/22/2020).
The results of a clinical trial of phase 1 performed in B-cell acute lymphoblastic leukemia patients using CAR-T cells were revealed [106]. In this study, a cohort of 53 patients treated with the chimeric antigen receptor CD19-specific CAR-T cells (named 19–28z CAR-T cells) demonstrated that 83% of the patients presented a complete remission with a median overall survival of 12.9 months [106]. Regarding that, almost all solid tumors share the same expression of antigens as the normal tissues, which can cause side-effects, since the CAR-T cells cannot distinguish which cell is cancerous or normal. Therefore, most studies and clinical trials involving CAR-T therapy have been performed in “liquid tumors” and are required for further studies on their application in solid tumors. Still, in a phase 1 study, the CAR-T cells therapy was applied to breast cancer and glioblastoma patients (NCT01837602 and NCT02209376, respectively). For instance, a human phase I study in 10 patients with EGFRvIII-expressing recurrent glioblastoma (GBM) showed that CAR-T cells that target EGFRvIII could be a valuable tool for GBM treatment. However, the CAR-T-EGFRvIII-treated patients demonstrated an increased immunosuppression microenvironment, which means that CAR-T therapy needs a reformulation to reduce the side effects such as inflammatory molecules [107]. To achieve this goal, a phase 1 study of EGFRvIII-Directed CAR-T cells combined with PD-1 inhibition with glioblastoma patients is currently active (NCT03726515) clinicaltrials.gov accessed date 11/22/2020. Moreover, to improve CAR-T treatment, Choi and colleagues created EGFRvIII CAR-T, and by using CRISPR-Cas9, disrupted the genes of the endogenous T-cell receptor (TRAC), beta-2 microglobulin (B2M) developing allogeneic universal cells and PD-1 (PDCD1), providing an enhanced response in the pre-clinical study [102].
Moreover, the metastatic breast cancer-expressing c-Met patients were submitted to intratumoral injections of mRNA c-Met-CAR-T, specifically targeting c-Met in tumor cells. The patients treated showed good tolerance and no inflammatory response by tumor cells; however, the tumor biopsies showed tumor necrosis areas. A study of phase 1 is ongoing to understand if these CAR-T cells have therapeutic potential for breast cancer patients (NCT03060356) [108].
In other solid tumors, such as melanoma and medulloblastoma, high levels of antigens expressed only by melanoma cells (PRAME) were observed, which means that it is a useful candidate for immunotherapy using TCR-T cells [109]. In fact, downregulation of PRAME has been associated with a reduction of proliferation and induction of apoptosis in leukemic and hepatocellular carcinoma cells [110]. Thus, the introduction of the Cas9 system (an inducible Cas9, iCas9) into α and β chains of TCR-T for targeting PRAME on medulloblastoma cells (iC9-SLL TCR) reduced the toxicity provoked by TCR-T therapy [111] and reduced the tumor growth in vivo. For instance, iC9-SLL TCR started to be used in refractory myeloid neoplasms patients in clinical trial phase 1; however, no results have been reported yet (ClinicalTrials.gov Identifier: NCT02743611).
Despite all the significant breakthroughs in the ACT area, many challenges remain difficult to manipulate. Among all, tumor heterogeneity challenges the identification of immune effector cells with different specifications without considering the time and cost that it takes to perform these therapies. Overall, the possibility of applying personalized therapy to all cancer patients is getting closer and closer to reality.
Cancer is one of the main reasons for death worldwide, and their known molecular alterations have been correlated with uncontrolled incidence and the lack of successful therapies. Those alterations and the strategies to circumvent them will be explored by us here. Nanomedicine has had incredible progress towards therapeutic efficacy in dreaded diseases such as cancer [9]. Nanotechnology is based on science engineering to create and design systems on a nano scale. This technology has been used to fight against cancer as a drug delivery system (DDS) to circumvent conventional drugs’ poor specificity and accuracy in targeting tumor cells [10]. Nanoparticles (NPs) have shown higher tumor accumulation related to non-tumoral tissues, but only a smaller fraction of the total administered formulation is delivered to the intended target site, indeed [7].
NP-based drug-delivery systems can be based on metals, proteins, peptides, biodegradable and biocompatible materials, such as lipid-based nanoparticles (LNPs) and synthetic or natural polymers (e.g., polymersomes) [10,112]. The NPs usually comprised a hydrophilic surface, a target-oriented biocompatible outer layer, and a central hydrophobic core or vice-versa. Many compounds or cargos can be loaded within the NP core, decorated on their surface, entrapped in the layers or conjugated into their chemical structures [7]. Indeed, the current understanding of NPs targeted delivery to tumors is based on a combination of various independent concepts: 1) EPR effect; 2) NP properties and design; 3) increased retention in the circulation due to the Poly (ethylene glycolethyleneglycol) (PEG)ylation process, and 4) ligand-receptor type interactions [113]. LNPs are one of the most biocompatible drug carriers with loading and delivery properties for both hydrophilic and hydrophobic compounds. Since their discovery in the 1960s by Bangham, in vitro studies have indicated that these NPs are taken by cells via endocytosis. With this process, the efficiency of intracellular drug delivery. On the other hand, after the cell’s endocytosis, liposomes are entrapped in the endosome organelle and destined to the lysosome, where the contents are posteriorly degraded by the lysosome’s enzymes, which limit the intracellular concentration of the drug payload [114,115]. There is a range of different kinds, namely the positively-charged liposomes, pH-sensitive liposomes, ligand or peptide grafted liposomes, virosomes, magnetic liposomes, and gold or silver particle-containing liposomes [116]. Among all the nanomedicines developed until now, LNPs have the highest approval rate by FDA and EMA [117–119] (European Medicines Agency).
Among other features of NPs, size is critical for controlling tumor accumulation kinetics and for preventing diffusion back into the systemic vascular bed. For instance, early studies have shown that liposomes (with 90 nm of diameter, approximately) extravasate from cracked tumor vessels but do not diffuse away adequately from the tumor even after a week [120]. Since the accumulation of NPs in tumors is based on blood circulation and extravasation, the lifetime of an NP in the systemic circulation is very important for a strategy taken to increment the fraction of NPs reaching the target. Cationic liposomes, and positively charged NPs interact with the negatively charged serum proteins forming aggregates or protein corona, which change their physicochemical properties (e.g., size, charge and stability). Thus, protein corona plays a crucial role in NPs circulation time promoting off-target sites and their recognition by immune cells [121]. PEGylation has been used extensively to modify the drug’s pharmacokinetics and the NPs. PEGylated NPs have higher systemic circulation times, due to their hydrophilic nature, providing an aqueous shield around the NP surface. Thus, reducing opsonization of NPs, and the subsequent recognition as non-intruders by the macrophages induces an NP blood residence lifetime [122]. However, only about 5% of the applied PEG-NPs remain in the systemic circulation after 12 h, while 80% of the initial dose is removed in a few hours in the spleen and liver, with a lesser extent in the kidneys and lungs [123]. To overcome the poor circulation time, several PEG-LNPs with different lengths of the acyl chain anchor were synthesized, such as ceramides (PEG-Cer)18 or diacylglycerols (PEG-S-DAGs), since the length of the acyl chain increases the PEG time on the NP surface [124]. Moreover, Near-infrared fluorophore-conjugated polyethylene glycols (PEG-ZW800s) with less than 20 kDa and 12 nm, were more efficient in targeting the tumor with less nonspecific uptakes with a higher tumor-to-background ratio (TBR) when compared with larger PEGs (>20 kDa and 13 nm) [125]. Nevertheless, a few limitations have been associated with PEG-LNPs, namely presence of anti-PEG antibodies which may lead to their rapid blood clearance [126], however this is still under discussion.
To further improve the intracellular delivery drug content to the cytosol by escaping the lysosome, pH-sensitive NPs were designed [127]. These NPs quickly become destabilized in the acidic pH environment of the late endosome (pH < 5.5) by enhancing cellular internalization and rapid intracellular drug release in cancer cells [128]. Polymeric NPs (also known as polymersomes) are a very handful tool due to the controlled size and shape, but more importantly, the possibility of functionalizing their surface with peptides or proteins for specific targeting. Recently, it was shown that pH-responsive polymersomes such as PMPC-Poly(2-(diisopropylamine) ethyl methacrylate (PDPA) can be used to specifically target and modulate monocytes through the clusterization of scavenger receptors expressed in inflammatory cells due to the phosphorylcholine group present in their surface [129]. Moreover, other functionalized polymeric NPs have been developed lately (Table 2). For instance, Angiopep-2 decorated polymersomes showed promising efficacy in crossing the BBB through LRP1 mediated transcytosis and specifically target tumor cells [130,131].
Nevertheless, the industry of NPs is still emerging, and a few NPs are under preclinical or clinical trials due to the improved therapeutic benefit. Liposomal doxorubicin (DoxilTM/CaelyxTM) was the first NP approved by the FDA in 1995. Since then, other NPs have also been approved for clinical use, namely MyocetTM, DaunoXomeTM, DepocytTM, AbraxaneTM, GenexolPMTM and OnivydeTM [9]. The most important NPs currently in clinical trials are summarized in Table 2.
Understanding the interactions of NPs with the immune and inflammatory systems is crucial, especially how the immune and inflammatory cells can recognize and eliminate those NPs, influencing their future design and therapeutic potential [146]. The specific power of the NPs to initiate and modulate the immune responses is due to the inherent capacity to target antigen-presenting cells (APCs) and conduct coordinated signals, leading to an antigen-specific immune response [147]. Regarding the inflammatory system, NPs have been used as a delivery tool for cytokines, such as IL-2 and IFNγ, which would help better control the inflammation usually associated with cancer treatments. Early studies demonstrated that LNPs composed of DSPC, DMPC and DPPC loaded these cytokines increased the therapeutic benefits compared with free formulations in an in vivo animal model of renal cell carcinoma and metastatic sarcoma in lungs [148]. Besides the LNPs, the folate-conjugated polymeric system of polyethyleneimine linked by β-cyclodextrin encapsulated with IL-2 showed similar efficacy when compared with the adenoviral gene therapy for recombinant IL-2 in an in vivo melanoma model [149]. Moreover, biomimetic NPs (leukosomes) loaded with rapamycin (mTOR inhibitor) were described to reduce pro-inflammatory cytokines and decrease MMP activity in the vascular inflammation model [150].
Recently, a dual-NP delivery system, incorporating acid-sensitive hydrazone linker into thermosensitive NPs (TSNs) loaded with doxorubicin (DOX) and IFNγ was exploited to verify the co-delivery potential of chemotherapy and immunotherapy agents against melanoma. This multifunctional TSNs system has a sustained and sensitive drug release, long circulation times and synergistic anti-tumor efficacy against B16F10 melanoma tumor bearing mice by activating CD4+ T cells, cytotoxic T lymphocytes and natural killer cells. Besides, a strong downregulation of immunosuppressive cytokines including IL-10 and TGFβ followed by increased levels of IL1 and TNFα were observed [151]. Overall, and particularly in cancer, NP platforms can help to significantly encourage immunogenicity of tumor antigens through the unification of the antigen and adjuvant transport kinetics by targeting specifically the APCs, which enables more efficient antigen processing and presentation [152]. Polymeric nanocarriers, namely the N-(2-hydroxypropyl) methacrylamide (HPMA) copolymers, can potentiate the delivery of drugs to tumor cells, avoiding the systemic toxicity of conventional chemotherapy. These HPMA-NPs can target inflammation sites and accumulate within innate immune cells, including TAMs by specifically targeting tumor-promoting macrophage activation [153]. Thus, NPs are a potential therapeutic approach either to target cancer cells or to modulate the immune system, ultimately improving the therapeutic response.
Currently, there are sixteen new clinical trials using ABI-009, NP albumin-bound mTOR inhibitor in bladder cancer, malignant perivascular epithelioid cell tumor (PEComa), or pulmonary arterial hypertension [154].
4 New-Engineered Therapeutic Approaches to Destroy Cancer Cells
During the last years, many groups have tested new therapeutic approaches using new biotechnologies for cancer treatment. Here, we will discuss the recent new-engineered discoveries applied to cancer treatment, namely the usage of injectable hydrogel-based drug delivery systems and the antibody–drug conjugates (ADCs).
Among the significant promising applications in the cancer field using biotechnology tools is the hydrogels to deliver locally drugs instead of systemic therapies. One of the major limitations of systemic treatment in cancer patients is related to the drug’s efficacy, lower biocompatibility and high cytotoxicity [155]. Hydrogels have low costs and easy manipulation of their structure, including the biodegradation, the pore size, and the stimuli necessary for the delivery of the biochemical molecules (i.e., pH, temperature) [156,157].
The chemotherapeutic drugs used demonstrated high efficacy in melanoma patients, such as doxorubicin. However, the side effects are extremely aggressive, and so other therapeutic approaches have been applied, namely hydrogels [158]. In vivo studies showed that the subcutaneous injection of DOX-incorporated hydrogel reduced melanoma growth in mice [159]. Hydrogel nanoparticle technology was improved by incorporating Fe3O4 magnetic nanoparticles into alginate (Alg) and gelatin (Gel) hydrogel loaded with DOX. In vitro model with Hela cells, Alg-Gel/Fe3O4 magnetic hydrogel displays a drug release behavior pH-dependent [160].
Hydrogels can be applied in cubical shape as an oral therapeutic approach to treat liver cancer patients. These hydrogels are composed of the polymer redox-sensitive poly (methacrylic acid) (PMAA), which incorporates a potent antineoplastic drug named 7-(benzylamine)−3, 4-dihydro-pyrrolo[4, 3, 2-de]quinolin-8(1H)-one (BA-TPQ) in cubic shape [161]. In vitro studies showed that HepG2 tumor cells were treated with BA-TPQ loaded-hydrogel presents a reduction of E3 ubiquitin-protein ligase Mdm2 MDM2 protein expression and consequently increased levels of tumor suppressor proteins, namely the P53 and P21 [161].
FDA has approved an absorbable hydrogel named SpaceOAR for prostate tumor treatment. A randomized phase III study showed that this hydrogel could reduce the toxicity and increase the quality of life of prostate cancer patients submitted to radiotherapy (RT). Currently, there are seven clinical trials using hydrogel systems for cancer care. For imaging purposes, the TraceIT, a hydrogel polyethylene glycol (PEG) micro-particle covalently bound with iodine, is being used to marker cancer tissues for up to 3 months. This approach will improve tumor visualization by computer tomography, resonance imaging, or ultrasound during surgical procedures [162].
However, the hydrogels are still under investigation regarding their usefulness as a therapeutic approach. Several groups are currently improving their applicability for the successful cancer treatment cancer [163–165].
4.1 Antibody–Drug Conjugates (ADCs)
In recent years, many efforts have been made to discover molecules only expressed by cancer cells which can be used as therapeutic targets to destroy them. In the 90s, the first therapy was approved using a monoclonal antibody (mAb) to treat patients with low-grade B cell lymphoma [166]. This therapy used specific non-human antibodies to target the specific molecules expressed on tumor cells. The failure of this type of therapy was attributed to the presence of immune cells that were detected as intruders and consequently destroyed. This therapy displays many limitations, namely the size of mAbs that limited their efficacy to target all tumor cells and the lower cytotoxic activity [167]. Thus, to attempt the limitations of using mAbs, another approach has been made to improve their efficacy, based on the usage of the mAbs in association with small molecules already used in chemotherapy, known as antibody-drug conjugates (ADCs) [168] (Fig. 2). Among all ADCs properties, the most advantageous is their stability during blood circulation and their ability to be released after internalization. Moreover, these features combined with the capability to deliver a high concentration of chemotherapeutic compounds to the tumor site, contribute to the reduced side effects compared to what is observed in conventional cancer treatments [168]. During the last years, ADC research increased, and many other ways to conjugate the ADC with target molecules to be used in cancer treatment have been discovered [169]. Although many of the ADCs are still in clinical phase studies, few were approved by the FDA, as pointed below. The first ADC approved by FDA was the brentuximab vedotin, also named Adcetris, which consists of conjugation of anti-CD30 antibody with monomethyl auristatin (MMAE) linked by a plasma-stable linker to be used in refractory Hodgkin lymphoma (HL) and systemic anaplastic large-cell lymphoma (ALCL) [137]. The HL and ALCL patients treated with Adcetris presented a therapeutic response of 75% and 86%, respectively, while the complete remissions were 34% for HL and 53% for ALCL-treated patients [137]. Moreover, these ADCs demonstrated high efficacy in reducing the progression of HL by inducing the cycle arrest and apoptosis in the CD30+ tumor cells-released MMAE [170].
Figure 2: Antibody-drug conjugates (ADCs). The ADCs are composed of antibodies with coupled anti-cancer drugs. They have more stability during blood circulation and better properties to be uptake by the cells. Firstly, the binding of the ACD to the specific receptor in cancer cells induces the endocytosis mechanism, for instance. Therefore, the dissociation of the ADC occurs in the endosome, and then ADC is degraded by the lysosome releasing the anti-tumoral payload drug into the cells (Figure done by Matias, D.)
A new ADC, the sacituzumab govitecan (IMMU-132), composed of antibody-Trop-2, a glycoprotein commonly highly expressed in epithelial tumors, and the topoisomerase inhibitor-SN-38, was described to have high efficacy in the reduction of the progression of the disease of the metastatic non–small-cell lung cancer (NSCLC) patients [171]. These studies are examples of the easy manipulation of ADC, which supports their importance as a therapeutic approach for many cancers, supporting personalized therapy. However, many side-effects induced by the ADCs have been observed in patients after the treatment, namely hepatic disturbances, neutropenia, diarrhea, leukopenia and toxicity in different structures and organs of the body such as skin and endothelial cells [167]. Thus, many efforts are underway to reduce the side effects and increase the outcome and quality of life of patients submitted to ADC treatment.
5.1 The Interface between the Omics and Cancer
As the cure for most cancers is far from being found, developing appropriate and effective biopharmaceuticals is still the focus of intense research. The development and improvement of technologies associated with the science of omics, such as sequencing techniques, allowed the evaluation of pathophysiological patterns in organisms via gene expression, protein, and DNA methylation analysis. This has increased the possibility of identifying potential therapeutic targets, enabling the development of personalized therapies and probably improving early diagnosis for different types of cancers [172]. These techniques also permit the generation of several data related to cellular heterogeneity, which could be associated with different diseases [173]. These data could be used then to enrich different sectors of the omics, and an excellent example of a database focused on this type of information is The Cancer Genome Atlas Research Network (TCGA) (https://cancergenome.nih.gov), which has free access to genomic data related to different types of human cancers.
The omics suffix is associated with the complete analysis of a specific biomolecule, whether a gene, protein, metabolite or lipid, among others [174]. The increase in data production in these sectors has allowed the discovery of countless biomarkers, which are defined as biological signatures capable of quantifying any physiological state or pathological condition of a given disease [173]. Moreover, it has supported our understanding of new biomarkers, making these new tools less invasive for diagnosis as they can be analyzed in blood, saliva, and urine [175,176], see Table 3.
Genomics has provided a robust amount of information that generates an increased understanding of the biology of cancers as a whole [177]. Although much has already advanced regarding oncogenes, the challenge of the applicability of this knowledge in development of effective therapies remains with respect to cancer treatment [178]. The data generated through genomics allows the analysis of the difference in copy number to be seen and partial deletion or replication of chromosomes, generating various gene expressions. This is usually seen in cases of patients with esophageal squamous cell carcinoma (ESCC) or esophageal adenocarcinoma (EAC), for example, where ERBB2 (Receptor tyrosine-protein kinase erbB-2, also known as CD340 (cluster of differentiation 340)) and VEGFA overexpression is observed and, mutations of these genes could be identified and used in personalized therapy [172]. Moreover, these patients could use inhibitory drugs for these genes, such as trastuzumab and ramucirumab, respectively, palbociclib, that target the cell cycle proteins. Furthermore, ESCC presents a molecular profile similar to the lung and head cancer subtypes, which could generate similar and effective personalized therapy for these cases [172].
Since transcriptomics helps to understand biological processes and how drugs act in different pathways of cellular metabolism, this field of omics has taken a unique approach to oropharyngeal cancers caused by Human-papilloma viruses (HPV), such as head and neck cancers, promoting high analysis of transcripts and genetic and epigenetics events [179,180]. Not only in breast cancer cell lines, but it was also demonstrated that the transcriptomic profile of these cells treated with anacardic acid (AnAc) showed a variation in the number of responsive genes, including the MIR22HG lncRNA [180]. Furthermore, AnAc (a dietary product) was implicated in TNFα inhibition in breast cells, inducing anti-proliferative and pro-apoptotic activities, being, therefore, a potential drug for the treatment and prevention of breast cancer [180].
Another omics-related study is proteomics, which can generate data relating to protein-protein interactions [173]. Hence, with proteomics, it is possible to reveal interferences in cell signaling pathways and in proteins related to biological processes, such as ones from the cytoskeleton, detecting interventions in nucleotide synthesis, signal transduction, and glycolysis [181]. All these data can be used to develop therapies and find biomarkers for different types of cancers [181]. Using proteomic analysis, such as MALDI-TOF and 2D-DIGE approaches, it was demonstrated that combining murine double minute2 (MDM2) (JNJ-26854165) and EGFR inhibitors (gefitinib) synergistically on various ovarian cell lines have therapeutic potential. Proteomic analysis showed that the ovarian cell lines treated with these inhibitors exhibited down-regulation of proteins involved in nucleotide biosynthesis, such as nucleoside diphosphate kinase B (NME2) [181]. Moreover, quantitative proteomic analysis of membrane proteins, from colorectal cancer tissue, with subsequent verification using Tissue Microarray Analysis (TMA) and Selected Reaction Monitoring (SRM), showed substantial differences in several proteins expression, including integrin alpha-5 (ITGA5), beta-type platelet-derived growth factor receptor (PDGFRB), retinoic acid-induced protein 3 (GPRC5A) and transferrin receptor protein 1 (TFRC). In particular, it was demonstrated that high levels of UPF0670 protein C8orf55 (C8orf55) with unknown function, highly expressed in different cancers, such as CRC and breast, suggest their use as a biomarker candidate for cancer in the diagnosis [182].
Epigenomics is based on modifications in gene expression patterns not caused by DNA nucleotide sequence alterations, but by histone modification, chromatin remodeling, DNA methylation, among other mechanisms [174]. Studies demonstrated that epigenetic deregulations are associated with the pathogenesis of several cancers. DNA methylation is the principal epigenetic modification that is powerfully involved in the physiological control of genome expression, and it is a hallmark of cancer [183]. It is important to note that when gene promoter regions are hypomethylated, it can result in the activation of various proto-oncogenes and chromatin restructuring [184].
On the other hand, DNA hypermethylation in cancer usually induces several gene silencing, and it usually is site-specific in CpG islands in gene promoter regions [185]. Recently, Chen and colleagues proposed a glioma classification based on DNA methylated profile as an approach to characterize molecular subtypes or new glioma markers to elucidate the glioma patient subtype [186]. As DNA methylation status is correlated with cancer prognosis, this supports the therapeutic potential of epigenomics [172,185,187,188].
Lipids play pivotal roles in cellular functions, such as proliferation, survival, and death. Comprehensive identification and quantification of all lipids, and the characterization of their interaction with proteins, other lipids and gene expression by lipidomics opens an avenue to discover new tumor biomarkers and targets [189]. Recently, it was possible to identify plasma differences in lipidome profile between different types of lung cancer and a healthy patient [190]. Besides, lung cancer-specific and subtype specific lipidomics are present in the circulation, demonstrating the relevant potential of these targets as biomarkers [190]. The potential of lipids as cancer biomarkers was exploited in patients with high-grade ovary carcinoma. Overall decreases in different classes of lipids, namely ceramide, phosphatidylcholines, lysophosphatidylcholines, sphingomyelins, and triacylglycerol in serum and tumor samples from these patients were identified [191]. Diacylglycerol is upregulated in metastatic osteosarcoma cell lines compared to nonmetastatic and osteoblast cell lines. Besides the potential use of diacylglycerol as a biomarker, it was shown that pharmacological inhibition of this lipid synthesis induced apoptosis and reduced migration on the metastatic osteosarcoma cell lines, highlighting the possibility for new targets for cancer treatment [192].
Metabolomics, unlike other omics, evaluates small molecules (less than 1,500 Dalton) generated by stimuli from an endogenous or exogenous origin [193]. Metabolites from the endogenous origin are naturally produced by cells and those from the exogenous origin are produced after stimulation with drugs and cosmetics [193]. Furthermore, metabolic profiles are a fertile system for defining phenotypes of several diseases, such as cancer, reflecting modifications in the epigenome, genome, proteome, and environment (lifestyle and exposures) [193]. For instance, succinate secretion for cancer cells can polarize macrophages to the M2 profile and promote cancer metastasis [194]. Considering the cellular metabolism in cancer, where high levels of cellular proliferation and metabolic remodeling occur, it is possible to establish a parameter for tumor grade differentiation [195].
Biomarkers are defined as measurable phenotypic parameters that can be used to differentiate the abnormal from normal status or a response to a particular therapeutic intervention [198]. This measurement commonly involves the assessment of genomic alterations or a difference in protein expression in the cell/tissues [199]. Cancer biomarkers are biomolecules applied for medical purposes, such as proteins, lipids, oligosaccharides, metabolites, and genetic material, including methylated DNAs, DNAs, microRNAs (miRNAs), and RNAs. Since cancers are heterogeneous diseases reflected by gene and protein alterations, identifying cancer biomarkers are critical for the success of personalized therapy [200]. DNA-based markers include chromosomal aberrations, single nucleotide polymorphisms (SNPs), changes in DNA copy number, microsatellite instability, and differential promoter-region methylation. The RNA-based biomarkers comprise over-expressed or under-expressed transcripts and regulatory RNAs, for instance, the miRNAs. In contrast, protein markers include cell surface receptors (i.e., CD20), tumor antigens such as prostate-specific antigen (PSA), phosphorylation states, carbohydrates determinants, and peptides released by tumors into serum, urine, sputum, or other body fluids. Since proteins are the main functional units of biological processes, almost all the FDA-approved cancer biomarkers are proteins [201], see Table 4
Biomarkers can be used in multiple clinical settings for patient evaluation, which include estimating the risk of disease, screening for silent cancers, and differentiating benign from malignant or one type of malignancy from another. Furthermore, it can be used to determine a tumor’s prognosis and recurrence in the absence of further treatment. If a prognostic biomarker indicates aggressive disease, further treatment is required to reduce the chance of recurrence. Besides, biomarkers can be used to predict treatment for patients diagnosed with cancer by helping foretell responses to specific therapeutic regimens and monitoring the disease status [201]. Some ideal properties of biomarkers are: (1) involvement in the process that causes the disease; (2) changes in the biomarker should be highly related to disease alterations; (3) should be capable of performing screening, predisposition, early detection, prognosis and drug response; (4) biomarkers levels should change only in response to successful treatment and (5) reduction in misclassification of exposure or risk factors and disease [202]. The concept of cancer biomarker development has also been evolving with the rapid expansion of omics analysis. Omics research has revealed molecular targets, and therapeutic and diagnostic assays for biomarker development, creating the basis for the so-called ‘Personalized medicine’ [202,203].
The first test recognized for common cancer was reported in 1965 by Dr. Joseph Gold, who found a substance in the blood of patients with colon cancer, typically found in fetal tissues, named carcinoembryonic antigen [204]. Afterward, potential tests were developed for various cancers [205]. Protein markers in clinical use nowadays include CA125 (cancer antigen 125) for ovarian cancer [206], CA19 (carbohydrate antigen 19) for pancreatic cancer [207], and PSA for prostate cancer [208], C3b and CYFRA 21–1 for bladder cancer [209]. However, they have shown limitations regarding their use due to low sensitivity and specificity in the early stages and the inability to distinguish aggressive from indolent tumors [210]. Human epidermal growth factor receptor 2 (HER2) is overexpressed/amplified and predicts the prognosis of response to monoclonal antibodies, including trastuzumab. This biomarker became the second targeted therapy for breast cancer, and companion diagnostics (used to determine the correct therapeutic agent for a patient) that assess levels of HER2 by immunohistochemistry or in situ hybridization have now been approved by the FDA [203]. Since then, several drugs that target EGFR have been approved, including the small molecule inhibitor gefitinib [211].
Impressive advance in personalized treatment due to genomic analysis has revealed that common tumors, namely breast cancer, are, in fact, a mixture of several molecular entities, where targeted drugs that inhibit biochemical pathways have become available. Advances in genomics and the application of genetic testing are now being used very effectively in oncology [212]. Clinical applications of novel genetic tools include sequencing and analysis of germline genomic rearrangements of key cancer genes, including BRCA1 (breast cancer 1), BRCA2 (breast cancer 2), TP53 (tumor protein p53); mismatched repair genes as MLH1 (MutL homolog 1), MSH2 (DNA mismatch repair protein 2), MSH6 (DNA mismatch repair protein 6) and PMS2 (mismatch repair endonuclease 2); KRAS gene mutation in colorectal cancer [213].
Many attempts and successes have been reported using nucleic acid-based methods to diagnose cancer or provide tools for patient follow-up [210]. Several studies have shown that increased levels of DNA fragments are often found in the blood of cancer patients [214], indicating the release of tumor-derived sequences into the plasma and providing a possibility for non-invasive cancer testing.
Besides, human blood samples contain biological materials such as cell-free DNA and RNA, proteins, circulating cells, and vesicles (i.e., exosomes) that can originate from different tissues, including cancer [215]. The ability to detect and characterize circulating cell-free tumor DNA (ctDNA) and circulating tumor cells (CTCs) by liquid biopsy analysis allows the use of repeated blood samples to verify and trace the ctDNA changes during disease progression or cancer treatment, highlighting their potential as blood biomarkers [216]. Although ctDNA analysis can have increased sensitivity and specificity compared to the analysis of other circulating biomarkers, taking a multi-marker approach may offer a more comprehensive insight into a patient’s disease [217].
The emergence of various therapeutic regimens to contain this fatal disease was witnessed [218]. Nevertheless, survival rates for patients with cancers, especially those detected at an advanced stage, remain discouragingly low, as observed in patients with breast, lung, colon, prostate, and ovarian cancers with hidden metastatic colonies in 60% of the cases. At this stage, therapeutic modalities are limited and ineffective. Thereby using sensitive biomarkers could help in early disease detection.
However, cancer is not a stable disease since diverse critical pathways are altered during the tumor progression. Multiple biomarker profiling seems to have a better advantage over a single marker [219]. DNA sequencing improves and becomes more cost-effectively; whole-genome sequencing could be routinely used for early tumor detection by identifying rare germline alterations. By using complex computational analysis and bioinformatics algorithms, it would be possible to integrate different biomarkers that are not restricted to genomic profiles and include transcriptome, methylation, and protein expression [199]. Thus, multiplexing will enable better diagnosis based on a more informative assessment of biomarker panels, providing a better disease prognosis.
6 Future Directions in a Personalized Cancer Therapy-Is This Possible?
6.1 MicroRNA and DNA Sequencing
The human genome is responsible for transcribing less than 2% of proteins, while 70% of the whole human genome encodes a subset of RNAs that cannot translate to proteins, named non-coding RNA [230]. That non-coding RNA, initially named riborégulateurs due to their function in regulating protein synthesis from mRNA, is emerging as a key to understanding of epigenetics regulation [231]. The database (miRBase Sequence Database-Release 22) contains 38589 entries representing hairpin precursor miRNAs, expressing 48885 mature miRNA products (http://www.mirbase.org, accessed on July 25th, 2018).
Several non-coding RNAs have been described and classified according to their size: short RNAs [microRNA (miRNA), small interfering RNA (siRNA), piwi-interacting RNA], with less than 200 nucleotides; and long RNA, comprising more than 200 nucleotides [232]. The miRNAs have been described as a post-transcriptional regulator of gene expression by their ability to attach to the 3´ untranslated region (UTR) of mRNA, leading to RNA degradation and/or translational repression [233]. Indeed, a single miRNA can also target different mRNA regions, as several miRNAs could attach to only one mRNA [234].
It has been described that miRNAs expression is altered in diverse pathological conditions, such as cancer [235]. As a promising non-invasive biomarker, miRNAs are easy to find and quantify in the serum [176]. The miRNA found in cancer patients is described to have a role as oncogenic miRNAs (OncomiR) or tumor suppressors’ miRNAs, depending on the context. For instance, the miRNA-29a/-b-c was reported as a tumor suppressor in lung tumors. However, in breast cancer, it has the opposite performance acting as an oncogene [236]. In bladder cancer, loss of miR-23b is associated with increased migration and invasion [237]. On the other hand, the same miRNA knockdown induces apoptosis and, therefore, a reduction in invasion in the renal cell carcinoma model [238]. The recognition of different functions of miRNAs indicates that in the future, the patient prognosis should be possible by analyzing specific miRNAs variations.
Recently, it was shown that miRNAs expression changes in CD3+ T cells stimulated with anti-CD3 antibodies appear to be a promising immunomodulatory agent for autoimmune diseases and organ transplantation treatment [239]. In breast cancer, miRNAs could modulate chemotherapeutic response increasing drug responsiveness [240]. Besides, treatment with antiangiogenic therapy and chemotherapy also demonstrated different miRNA profiles after the treatment [241]. Emerging evidence demonstrated that miRNAs signature identification could also provide an alternative treatment for cancer therapies.
Personalized medicine in cancer is based on molecular analysis of cancer cells better to understand cancer physiology to drug response [242]. The differences are comparable to unaffected tissue, skin biopsy, peripheral blood, or public databases to associate the mutation with tumor phenotype and assist in the chemotherapy choice [243].
The new medical advances in CAR-T cell therapy to treat cancer can be used as personalized approaches for patients. CAR-T cells targeting CD19 induced deep molecular remission in patients with refractory and relapsed chronic and acute lymphocytic leukemia [244]. It is believed that it was associated with CAR-T cells’ long-lasting persistence and function [244].
Nevertheless, not only could CAR-T cells be used in personalized medicine in cancer, but thus identification of protein mutation is also an excellent step toward a successful treatment. For instance, the epidermal growth factor receptor (EGFR) is overexpressed in many of NSCLC committed patients. Those patients are usually treated with epidermal growth factor receptor tyrosine kinase inhibitor (EGFR-TKIs) [245], and identifying EGFR mutations allows the specific targeting and, consequently, the precise treatment approach. The first generation of EGFR-TKIs (G719X/L861Q/S768I), including erlotinib and gefitinib, showed less efficacy and progression-free survival in patients with uncommon EGFR mutation as compared with patients with common mutations [246].
Thus, a full genetic screen of the DNA of cancer patients is necessary before treatment initiation to apply the best therapeutic approach. It is widely described the emergence of cancer drug resistance during the treatments related to new mutations [247]. Droplet digital PCR (ddPCR) is a key approach to following the patient during the treatment. Thus, this approach allows the identification of low-frequency mutation, which can arise during the treatment, supporting an early change if necessary [248]. By following up on the patient’s ctDNA, it could be possible to identify the new mutations before the phenotype resurgence to change the treatment earlier.
New technological advances allowed organoid models to be a powerful tool for personalized therapy. The rudiment principle of organoid formation in vitro began with developing several methodologies, including the culture of parts of tissue, to understand organogenesis [249]. Much progress has been made in this field, and the discovery of the potential of pluripotency of human embryonic stem cells (hESCs) allowed the scientist to culture, in vitro, somatic cell types and differentiate them under defined and specific conditions. Moreover, the manipulation of hESCs provides the creation of different models of diseases, such as organoids [250]. Recently, Marina Simian and Mina J. Bissell described the definition of organoid as “…a unit of function of a given organ that can reproduce, in culture, a biological structure similar in architecture and function to its counterpart in vivo ” [251].
The progress of organoid methodology enables organoid biobanks derived from adult stem cells genetically modified (iPSCs) from healthy subjects and patient-derived tumor cells [252].
The establishment of an organoid biobank with 20 colorectal cancer patients and their equivalent healthy tissue-derived organoids in 2015 allowed for two years later the creation of proteomic and transcriptomic bases [253]. Substantial differences in protein profile between tumor organoids from different patients highlight the possibility of using the organoid model to test several drugs to be applied as personalized medicine. Moreover, the differences between tumor organoid proteins and the matching organoids derived from normal tissue have been exploited [253]. Recently, organoids cultured from endoscopic biopsies of esophageal adenocarcinoma resembled the originating tumor histology, molecular features, and sensitivity to anti-HER2 treatment using trastuzumab [254].
Nevertheless, we must consider the necessity to analyze the proteins from patient tumor biopsies to confirm if the protein profile is preserved on the organoids.
6.4 Tumor Microenvironment and Therapy
Efforts are also being made to modulate TME concerning cancer treatment. CAFs suffer a metabolic reprogramming dictated by the tumor cells [255]. Previous studies have demonstrated that CAFs are associated with a phenomenon named the “reverse Warburg effect” by metabolizing glucose through anaerobic glycolysis and exporting lactate that will be uptaken by oxidative cancer cells to raise their tumorigenic potential [256]. Cancer cells usually induce this phenomenon in response to oxidative stress injuries or hypoxic conditions [257]. Nowadays, this concept proposes that the treatment can prevent the transport and generation of lactate through the inhibition of monocarboxylate transporters and, in turn, combat the cancer cells by arresting them along with a silencing TME [258].
The remodeling of extracellular matrix (ECM) is a hallmark of cancer propitiating the invasion of cancer cells for other tissues [218]. It was recently described the role of metalloproteinase-14 (MMP14) deletion in fibroblasts as a modulator of ECM affecting the melanoma progression through collagen XIV accumulation [259]. The ECM are also involved regulating the exomes implied with immune evasion and TME rearrangement as extensively reviewed by Karampoga et al. [260]. Another critical player in the formation and progression of cancer is the vasculature niche within the TME, having tumor-promoting effects [261]. remodeling It has been shown that tumor endothelial cells are essential to promote resistance to chemotherapy and lymphoma cell invasiveness [262]. Tumor endothelial cells (TECs) create perivascular niches in some types of cancers, such as head and neck squamous cell carcinomas, where most cancer-initiating cells (CICs) are located. For instance, endothelial cells secrete factors that promote CICs self-renewal, proliferation, and survival [263]. Endothelial cells also secrete tumor stimulating factors, such as IL-6, CXCL8, and EGF [2]. The interaction between endothelial cells and bladder cancer cells induces CXC chemokine and CXCR2 pathways culminating with endothelial cell recruitment and cancer progression [264].
Moreover, TECs form blood vessels that supply the tumor’s nutrients and oxygen, support its progression and provide a portal for tumor metastasis [25]. So far, some anti-angiogenic drugs have been developed for targeting the VEGF/VEGF receptor (vascular endothelial growth factor), which are administered with chemotherapeutic drugs in many cancers. However, the side effects provoked by this treatment are unfortunately very severe (intestinal perforations and fatal hemoptysis) [265,266].
Here exploited the recent hallmarks concerning cancer treatment, diagnosis and prognosis. Cancer is a deadly disease, and the search for effective therapeutic approaches is further far from being discovered. TME has gained tremendous attention, as pivotal in tumor initiation, progression and metastasis [59]. There is no doubt that many factors can change the features of TME that should be considered when therapy is planned [2].
Future therapies will have to consider the composition of the TME of the patients, detection of cancer biomarkers in body fluids, search for mutations, and test possible therapies using organoid models for ‘Personalized medicine’. Therefore, the identification and validation of robust biomarkers, which refers to tumor characteristics or a body response to it, can be measured and evaluated with the ability to predict sensitivity or resistance to such therapies.
Unfortunately, the role of organoids in drug development is still under discussion. However, it is considered the closest model from the patient and an accurate clinical model compared with 2D culture cells and mice models. Drug screening showed a correlation between organoid drug response and patients, of which 88% predicted the efficacy of a specific drug and 100% the susceptibility to work or not for a specific cancer [267].
Nevertheless, the total number of cured cancer patients is still far from “reality”; the progression of nanomedicine combined with personalized medicine models shows that science is in the right direction to achieve this goal.
Authorship Statement: The authors confirm contribution to the paper as follows: study conception and design: Natalia Roque, Diana Isabel Lourenço Matias, Tania Cristina Leite de Sampaio e Spohr; data collection: Joana Balça Pinheiro da Costa e Silva, Valéria Pereira Ferrer, Luciana Santos Pessoa. Draft manuscript preparation: Natalia Roque, Diana Isabel Lourenço Matias, Tania Cristina Leite de Sampaio e Spohr. All authors reviewed the results and approved the final version of the manuscript.
Ethics Approval and Informed Consent Statement: Not applicable.
Funding Statement: The authors received no specific funding for this study.
Conflicts of Interest: The authors declare that they have no conflicts of interest to report regarding the present study.
References
1. Anderson, N. M., Simon, M. C. (2020). The tumor microenvironment. Current Biology, 30(16), R921–R925. DOI 10.1016/j.cub.2020.06.081. [Google Scholar] [CrossRef]
2. Kolenda, T., Przybyła, W., Kapałczyńska, M., Teresiak, A., Zajączkowska, M. et al. (2018). Tumor microenvironment-unknown niche with powerful therapeutic potential. Reports of Practical Oncology and Radiotherapy, 23(3), 143–153. DOI 10.1016/j.rpor.2018.01.004. [Google Scholar] [CrossRef]
3. Lim, A. R., Rathmell, W. K., Rathmell, J. C. (2020). The tumor microenvironment as a metabolic barrier to effector T cells and immunotherapy. elife, 9, e55185. DOI 10.7554/eLife.55185. [Google Scholar] [CrossRef]
4. Cassetta, L., Kitamura, T. (2018). Targeting tumor-associated macrophages as a potential strategy to enhance the response to immune checkpoint inhibitors. Frontiers in Cell and Developmental Biology, 6, 38. DOI 10.3389/fcell.2018.00038. [Google Scholar] [CrossRef]
5. Caja, F., Vannucci, L. (2015). TGFbeta: A player on multiple fronts in the tumor microenvironment. Journal of Immunotoxicology, 12(3), 300–307. DOI 10.3109/1547691X.2014.945667. [Google Scholar] [CrossRef]
6. Mantovani, A., Marchesi, F., Malesci, A., Laghi, L., Allavena, P. (2017). Tumour-associated macrophages as treatment targets in oncology. Nature Reviews Clinical Oncology, 14(7), 399–416. DOI 10.1038/nrclinonc.2016.217. [Google Scholar] [CrossRef]
7. Ashfaq, U. A., Riaz, M., Yasmeen, E., Yousaf, M. Z. (2017). Recent advances in nanoparticle-based targeted drug-delivery systems against cancer and role of tumor microenvironment. Critical Reviews in Therapeutic Drug Carrier Systems, 34(4), 317–353. DOI 10.1615/CritRevTherDrugCarrierSyst.2017017845. [Google Scholar] [CrossRef]
8. Matsumura, Y. (2014). The drug discovery by NanoMedicine and its clinical experience. Japanese Journal of Clinical Oncology, 44(6), 515–525. DOI 10.1093/jjco/hyu046. [Google Scholar] [CrossRef]
9. Hare, J. I., Lammers, T., Ashford, M. B., Puri, S., Storm, G. et al. (2017). Challenges and strategies in anti-cancer nanomedicine development: An industry perspective. Advanced Drug Delivery Reviews, 108, 25–38. DOI 10.1016/j.addr.2016.04.025. [Google Scholar] [CrossRef]
10. Duro-Castano, A., Moreira Leite, D., Forth, J., Deng, Y., Matias, D. et al. (2020). Designing peptide nanoparticles for efficient brain delivery. Advanced Drug Delivery Reviews, 160, 52–77. DOI 10.1016/j.addr.2020.10.001. [Google Scholar] [CrossRef]
11. Kasakovski, D., Xu, L., Li, Y. (2018). T cell senescence and CAR-T cell exhaustion in hematological malignancies. Journal of Hematology & Oncology, 11(1), 91. [Google Scholar]
12. June, C. H., Sadelain, M. (2018). Chimeric antigen receptor therapy. New England Journal of Medicine, 379(1), 64–73. [Google Scholar]
13. Hong, M., Clubb, J. D., Chen, Y. Y. (2020). Engineering CAR-T cells for next-generation cancer therapy. Cancer Cell, 38(4), 473–488. [Google Scholar]
14. van den Berg, J. H., Heemskerk, B., van Rooij, N., Gomez-Eerland, R., Michels, S. et al. (2020). Tumor infiltrating lymphocytes (TIL) therapy in metastatic melanoma: Boosting of neoantigen-specific T cell reactivity and long-term follow-up. Journal for Immunotherapy of Cancer, 8(2), e000848. [Google Scholar]
15. Qian, J., Olbrecht, S., Boeckx, B., Vos, H., Laoui, D. et al. (2020). A pan-cancer blueprint of the heterogeneous tumor microenvironment revealed by single-cell profiling. Cell Research, 30(9), 745–762. [Google Scholar]
16. Sohel, M. M. H. (2020). Circulating microRNAs as biomarkers in cancer diagnosis. Life Sciences, 248, 117473. [Google Scholar]
17. Schilsky, R. L. (2014). Implementing personalized cancer care. Nature Reviews Clinical Oncology, 11(7), 432–438. [Google Scholar]
18. Hui, L., Chen, Y. (2015). Tumor microenvironment: Sanctuary of the devil. Cancer Letters, 368(1), 7–13. [Google Scholar]
19. Im, J. H., Buzzelli, J. N., Jones, K., Franchini, F., Gordon-Weeks, A. et al. (2020). FGF2 alters macrophage polarization, tumour immunity and growth and can be targeted during radiotherapy. Nature Communications, 11(1), 4064. [Google Scholar]
20. Petty, M. A., Lo, E. H. (2002). Junctional complexes of the blood-brain barrier: Permeability changes in neuroinflammation. Progress in Neurobiology, 68(5), 311–323. [Google Scholar]
21. Chen, M., Sharma, A., Lin, Y., Wu, Y., He, Q. et al. (2019). Insluin and epithelial growth factor (EGF) promote programmed death ligand 1 (PD-L1) production and transport in colon cancer stem cells. BMC Cancer, 19(1), 1–12. DOI 10.1186/s12885-019-5364-3. [Google Scholar] [CrossRef]
22. Orimo, A., Gupta, P. B., Sgroi, D. C., Arenzana-Seisdedos, F., Delaunay, T. et al. (2005). Stromal fibroblasts present in invasive human breast carcinomas promote tumor growth and angiogenesis through elevated SDF-1/CXCL12 secretion. Cell, 121(3), 335–348. DOI 10.1016/j.cell.2005.02.034. [Google Scholar] [CrossRef]
23. Klionsky, D. J., Abdelmohsen, K., Abe, A., Abedin, M. J., Abeliovich, H. et al. (2016). Guidelines for the use and interpretation of assays for monitoring autophagy (3rd edition). Autophagy, 12(1), 1–222. DOI 10.1080/15548627.2015.1100356. [Google Scholar] [CrossRef]
24. Winkler, J., Abisoye-Ogunniyan, A., Metcalf, K. J., Werb, Z. (2020). Concepts of extracellular matrix remodelling in tumour progression and metastasis. Nature Communications, 11(1), 5120. DOI 10.1038/s41467-020-18794-x. [Google Scholar] [CrossRef]
25. Hida, K., Maishi, N., Annan, D. A., Hida, Y. (2018). Contribution of tumor endothelial cells in cancer progression. International Journal of Molecular Sciences, 19(5), 1–12. DOI 10.3390/ijms19051272. [Google Scholar] [CrossRef]
26. Li, Z., Zhou, J., Zhang, J., Li, S., Wang, H. et al. (2019). Cancer-associated fibroblasts promote PD-L1 expression in mice cancer cells via secreting CXCL5. International Journal of Cancer, 145(7), 1946–1957. DOI 10.1002/ijc.32278. [Google Scholar] [CrossRef]
27. Spits, H., Artis, D., Colonna, M., Diefenbach, A., di Santo, J. P. et al. (2013). Innate lymphoid cells--A proposal for uniform nomenclature. Nature Reviews Immunology, 13(2), 145–149. DOI 10.1038/nri3365. [Google Scholar] [CrossRef]
28. Sonnenberg, G. F., Hepworth, M. R. (2019). Functional interactions between innate lymphoid cells and adaptive immunity. Nature Reviews Immunology, 19(10), 599–613. DOI 10.1038/s41577-019-0194-8. [Google Scholar] [CrossRef]
29. Hosseini, S. H., Sharafkandi, N., Seyfizadeh, N., Hemmatzadeh, M., Marofi, F. et al. (2020). Progression or suppression: Two sides of the innate lymphoid cells in cancer. Journal of Cellukar Biochemistry, 121(4), 2739–2755. DOI 10.1002/jcb.29503. [Google Scholar] [CrossRef]
30. Chaudhary, B., Elkord, E. (2016). Regulatory T cells in the tumor microenvironment and cancer progression: Role and therapeutic targeting. Vaccines, 4(3), 28. DOI 10.3390/vaccines4030028. [Google Scholar] [CrossRef]
31. Nishikawa, H., Sakaguchi, S. (2014). Regulatory T cells in cancer immunotherapy. Current Opinion in Immunology, 27, 1–7. DOI 10.1016/j.coi.2013.12.005. [Google Scholar] [CrossRef]
32. Haas, M., Dimmler, A., Hohenberger, W., Grabenbauer, G. G., Niedobitek, G. et al. (2009). Stromal regulatory T-cells are associated with a favourable prognosis in gastric cancer of the cardia. BMC Gastroenterology, 9, 65. DOI 10.1186/1471-230X-9-65. [Google Scholar] [CrossRef]
33. Salama, P., Phillips, M., Grieu, F., Morris, M., Zeps, N. et al. (2009). Tumor-infiltrating FOXP3+ T regulatory cells show strong prognostic significance in colorectal cancer. Journal of Clinical Oncology, 27(2), 186–192. DOI 10.1200/JCO.2008.18.7229. [Google Scholar] [CrossRef]
34. Ohue, Y., Nishikawa, H. (2019). Regulatory T (Treg) cells in cancer: Can treg cells be a new therapeutic target? Cancer Science, 110(7), 2080–2089. DOI 10.1111/cas.14069. [Google Scholar] [CrossRef]
35. Patil, R. S., Bhat, S. A., Dar, A. A., Chiplunkar, S. V. (2015). The jekyll and hyde story of IL17-producing gammadeltaT cells. Frontiers in Immunology, 6, 37. [Google Scholar]
36. Ribot, J. C., Lopes, N., Silva-Santos, B. (2021). γδ T cells in tissue physiology and surveillance. Nature Reviews Immunology, 21(4), 221–232. DOI 10.1038/s41577-020-00452-4. [Google Scholar] [CrossRef]
37. Prinz, I., Silva-Santos, B., Pennington, D. J. (2013). Functional development of γδ T cells. European Journal of Immunology, 43(8), 1988–1994. DOI 10.1002/eji.201343759. [Google Scholar] [CrossRef]
38. Willcox, C. R., Mohammed, F., Willcox, B. E. (2020). The distinct MHC-unrestricted immunobiology of innate-like and adaptive-like human γδ T cell subsets—Nature’s CAR-T cells. Immunological Reviews, 298(1), 25–46. DOI 10.1111/imr.12928. [Google Scholar] [CrossRef]
39. Albini, A., Bruno, A., Noonan, D. M., Mortara, L. (2018). Contribution to tumor angiogenesis from innate immune cells within the tumor microenvironment: Implications for immunotherapy. Frontiers Immunology, 9, 527. DOI 10.3389/fimmu.2018.00527. [Google Scholar] [CrossRef]
40. Rei, M., Pennington, D. J., Silva-Santos, B. (2015). The emerging protumor role of γδ T lymphocytes: Implications for cancer immunotherapy. Cancer Research, 75(5), 798–802. DOI 10.1158/0008-5472.CAN-14-3228. [Google Scholar] [CrossRef]
41. Morvan, M. G., Lanier, L. L. (2016). NK cells and cancer: You can teach innate cells new tricks. Nature Reviews Cancer, 16(1), 7–19. [Google Scholar]
42. Elinav, E., Nowarski, R., Thaiss, C. A., Hu, B., Jin C. et al. (2013). Inflammation-induced cancer: Crosstalk between tumours, immune cells and microorganisms. Nature Reviews Cancer, 13(11), 759–771. [Google Scholar]
43. Herszenyi, L., Barabas, L., Miheller, P., Tulassay, Z. (2015). Colorectal cancer in patients with inflammatory bowel disease: The true impact of the risk. Digestive Diseases, 33(1), 52–57. [Google Scholar]
44. Nussbaum, K., Burkhard, S. H., Ohs, I., Mair, F., Klose, C. S. N. et al. (2017). Tissue microenvironment dictates the fate and tumorsuppressive function of type 3 ILCs. The Journal of Experimental Medicine, 214(8), 2331–2347. [Google Scholar]
45. Bruchard, M., Ghiringhelli, F. (2019). Deciphering the roles of innate lymphoid cells in cancer. Frontiers in Immunology, 10, 656. [Google Scholar]
46. Ghaedi, M., Ohashi, P. S. (2020). ILC transdifferentiation: Roles in cancer progression. Cell Research, 30(7), 562–563. [Google Scholar]
47. Siegler, J. J., Correia, M. P., Hofman, T., Prager, I., Birgin, E. et al. (2022). Human ILC3 exert TRAIL-mediated cytotoxicity towards cancer cells. Frontiers Immunology, 13, 742571. [Google Scholar]
48. Xuan, X., Zhou, J., Tian, Z., Lin, Y., Song, J. et al. (2020). ILC3 cells promote the proliferation and invasion of pancreatic cancer cells through IL-22/AKT signaling. Clinical & Translational Oncology, 22, 563–575. [Google Scholar]
49. Liu, Y., Wei, G., Cheng, W. A., Dong, Z., Sun, H. et al. (2018). Targeting myeloid-derived suppressor cells for cancer immunotherapy. Cancer Immunology Immunotherapy, 67(8), 1181–1195. [Google Scholar]
50. Shurin, G. V., Ma, Y., Shurin, M. R. (2013). Immunosuppressive mechanisms of regulatory dendritic cells in cancer. Cancer Microenvironment, 6(2), 159–167. [Google Scholar]
51. Ibáñez-Vea, M., Zuazo, M., Gato, M., Arasanz, H., Fernández-Hinojal, G. et al. (2018). Myeloid-derived suppressor cells in the tumor microenvironment: Current knowledge and future perspectives. Archivum Immunologiae Therapiae Experimentalis, 66(2), 113–123. DOI 10.1007/s00005-017-0492-4. [Google Scholar] [CrossRef]
52. Safarzadeh, E., Orangi, M., Mohammadi, H., Babaie, F., Baradaran, B. (2018). Myeloid-derived suppressor cells: Important contributors to tumor progression and metastasis. Journal of Cellular Physiology, 233(4), 3024–3036. DOI 10.1002/jcp.26075. [Google Scholar] [CrossRef]
53. Aponte-López, A., Fuentes-Pananá, E. M., Cortes-Muñoz, D., Muñoz-Cruz, S. (2018). Mast cell, the neglected member of the tumor microenvironment: Role in breast cancer. Journal of Immunology Research, 2018, 2584243. DOI 10.1155/2018/2584243. [Google Scholar] [CrossRef]
54. Amini, R. M., Aaltonen, K., Nevanlinna, H., Carvalho, R., Salonen, L. et al. (2007). Mast cells and eosinophils in invasive breast carcinoma. BMC Cancer, 7, 165. DOI 10.1186/1471-2407-7-165. [Google Scholar] [CrossRef]
55. Rajput, A. B., Turbin, D. A., Cheang, M. C., Voduc, D. K., Leung, S. et al. (2008). Stromal mast cells in invasive breast cancer are a marker of favourable prognosis: A study of 4,444 cases. Breast Cancer Research and Treatment, 107, 249–257. DOI 10.1007/s10549-007-9546-3. [Google Scholar] [CrossRef]
56. della Rovere, F., Granata, A., Familiari, D., D’Arrigo, G., Mondello, B. et al. (2007). Mast cells in invasive ductal breast cancer: Different behavior in high and minimum hormone-receptive cancers. Anticancer Research, 27(4B), 2465–2471. [Google Scholar]
57. Ribatti, D., Crivellato, E. (2012). Mast cells, angiogenesis and tumour growth. Biochimica et Biophysica Acta, 1822(1), 2–8. DOI 10.1016/j.bbadis.2010.11.010. [Google Scholar] [CrossRef]
58. Yuan, H., Hsiao, Y. H., Zhang, Y., Wang, J., Yin, C. et al. (2013). Destructive impact of t-lymphocytes, NK and mast cells on basal cell layers: Implications for tumor invasion. BMC Cancer, 13, 258. DOI 10.1186/1471-2407-13-258. [Google Scholar] [CrossRef]
59. Binnewies, M., Roberts, E. W., Kersten, K., Chan, V., Fearon, D. F. et al. (2018). Understanding the tumor immune microenvironment (TIME) for effective therapy. Nature Medicine, 24(5), 541–550. DOI 10.1038/s41591-018-0014-x. [Google Scholar] [CrossRef]
60. Coffelt, S. B., Kersten, K., Doornebal, C. W., Weiden, J., Vrijland, K. et al. (2015). IL-17-producing gammadelta T cells and neutrophils conspire to promote breast cancer metastasis. Nature, 522(7556), 345–348. DOI 10.1038/nature14282. [Google Scholar] [CrossRef]
61. Nywening, T. M., Wang-Gillam, A., Sanford, D. E., Belt, B. A., Panni, R. Z. et al. (2016). Targeting tumour-associated macrophages with CCR2 inhibition in combination with FOLFIRINOX in patients with borderline resectable and locally advanced pancreatic cancer: A single-centre, open-label, dose-finding, non-randomised, phase 1b trial. The Lancet Oncology, 17(5), 651–662. DOI 10.1016/S1470-2045(16)00078-4. [Google Scholar] [CrossRef]
62. Finisguerra, V., di Conza, G., di Matteo, M., Serneels, J., Costa, S. et al. (2015). MET is required for the recruitment of anti-tumoural neutrophils. Nature, 2015, 522(7556), 349–353. DOI 10.1038/nature14407. [Google Scholar] [CrossRef]
63. Wculek, S. K., Malanchi, I. (2015). Neutrophils support lung colonization of metastasis-initiating breast cancer cells. Nature, 528, 413–417. DOI 10.1038/nature16140. [Google Scholar] [CrossRef]
64. Fridlender, Z. G., Sun, J., Kim, S., Kapoor, V., Cheng, G. et al. (2009). Polarization of tumor-associated neutrophil phenotype by TGF-β: “N1” versus “N2” TAN. Cancer Cell, 16(3), 183–194. DOI 10.1016/j.ccr.2009.06.017. [Google Scholar] [CrossRef]
65. Saha, S., Biswas, S. K. (2016). Tumor-associated neutrophils show phenotypic and functional divergence in human lung cancer. Cancer Cell, 30(1), 11–13. DOI 10.1016/j.ccell.2016.06.016. [Google Scholar] [CrossRef]
66. De, P. M., Biziato, D., Petrova, T. V. (2017). Microenvironmental regulation of tumour angiogenesis. Nature Reviews Cancer, 17(8), 457–474. DOI 10.1038/nrc.2017.51. [Google Scholar] [CrossRef]
67. Margaroli, C., Cardenas, M. A., Jansen, C. S., Moon Reyes, A., Hosseinzadeh, F. et al. (2020). The immunosuppressive phenotype of tumor-infiltrating neutrophils is associated with obesity in kidney cancer patients. Oncoimmunology, 9(1), 1747731. DOI 10.1080/2162402X.2020.1747731. [Google Scholar] [CrossRef]
68. Donskov, F. (2013). Immunomonitoring and prognostic relevance of neutrophils in clinical trials. Seminars in Cancer Biology, 23(3), 200–207. DOI 10.1016/j.semcancer.2013.02.001. [Google Scholar] [CrossRef]
69. Galdiero, M. R., Bianchi, P., Grizzi, F., di Caro, G., Basso, G. et al. (2016). Occurrence and significance of tumor-associated neutrophils in patients with colorectal cancer. International Journal of Cancer, 139(2), 446–456. DOI 10.1002/ijc.30076. [Google Scholar] [CrossRef]
70. Qian, B. Z., Pollard, J. W. (2010). Macrophage diversity enhances tumor progression and metastasis. Cell, 141(1), 39–51. DOI 10.1016/j.cell.2010.03.014. [Google Scholar] [CrossRef]
71. Zhang, J., Sarkar, S., Cua, R., Zhou, Y., Hader, W. et al. (2012). A dialog between glioma and microglia that promotes tumor invasiveness through the CCL2/CCR2/interleukin-6 axis. Carcinogenesis, 33(2), 312–319. DOI 10.1093/carcin/bgr289. [Google Scholar] [CrossRef]
72. Cavnar, M. J., Turcotte, S., Katz, S. C., Kuk, D., Gönen, M. et al. (2017). Tumor-associated macrophage infiltration in colorectal cancer liver metastases is associated with better outcome. Annals of Surgical Oncology, 24(7), 1835–1842. DOI 10.1245/s10434-017-5812-8. [Google Scholar] [CrossRef]
73. Yin, S., Huang, J., Li, Z., Zhang, J., Luo, J. et al. (2017). The prognostic and clinicopathological significance of tumor-associated macrophages in patients with gastric cancer: A meta-analysis. PLoS One, 12(1), e0170042. DOI 10.1371/journal.pone.0170042. [Google Scholar] [CrossRef]
74. Zhao, X., Qu, J., Sun, Y., Wang, J., Liu, X. et al. (2017). Prognostic significance of tumor-associated macrophages in breast cancer: A meta-analysis of the literature. Oncotarget, 8(18), 30576–30586. DOI 10.18632/oncotarget.15736. [Google Scholar] [CrossRef]
75. Nahrendorf, M., Swirski, F. K. (2016). Abandoning M1/M2 for a network model of macrophage function. Circulation Research, 119(3), 414–417. DOI 10.1161/CIRCRESAHA.116.309194. [Google Scholar] [CrossRef]
76. Ma, R. Y., Black, A., Qian, B. Z. (2022). Macrophage diversity in cancer revisited in the era of single-cell omics. Trends in Immunology, 43(7), 546–563. DOI 10.1016/j.it.2022.04.008. [Google Scholar] [CrossRef]
77. Pasciuto, E., Burton, O. T., Roca, C. P., Lagou, V., Rajan, W. D. et al. (2020). Microglia require CD4 T cells to complete the fetal-to-adult transition. Cell, 182(3), 625–640. DOI 10.1016/j.cell.2020.06.026. [Google Scholar] [CrossRef]
78. Ochocka, N., Segit, P., Walentynowicz, K. A., Wojnicki, K., Cyranowski, S. et al. (2021). Single-cell RNA sequencing reveals functional heterogeneity of glioma-associated brain macrophages. Nature Communications, 12(1), 1151. DOI 10.1038/s41467-021-21407-w. [Google Scholar] [CrossRef]
79. Lin, E. Y., Nguyen A., V., Russell, R. G., Pollard, J. W. (2001). Colony-stimulating factor 1 promotes progression of mammary tumors to malignancy. The Journal of Experimental Medicine, 193(6), 727–740. DOI 10.1084/jem.193.6.727. [Google Scholar] [CrossRef]
80. Condeelis, J., Pollard, J. W. (2006). Macrophages: Obligate partners for tumor cell migration, invasion, and metastasis. Cell, 124(2), 263–266. DOI 10.1016/j.cell.2006.01.007. [Google Scholar] [CrossRef]
81. Pollard, J. W. (2004). Opinion: Tumour-educated macrophages promote tumour progression and metastasis. Nature Reviews Cancer, 4(1), 71–78. DOI 10.1038/nrc1256. [Google Scholar] [CrossRef]
82. DeNardo, D. G., Barreto, J. B., Andreu, P., Vasquez, L., Tawfik, D. et al. (2009). CD4+ T cells regulate pulmonary metastasis of mammary carcinomas by enhancing protumor properties of macrophages. Cancer Cell, 16(2), 91–102. DOI 10.1016/j.ccr.2009.06.018. [Google Scholar] [CrossRef]
83. Hambardzumyan, D., Gutmann, D. H., Kettenmann, H. (2016). The role of microglia and macrophages in glioma maintenance and progression. Nature Neuroscience, 19(1), 20–27. DOI 10.1038/nn.4185. [Google Scholar] [CrossRef]
84. Woolf, Z., Swanson, M. E. V., Smyth, L. C., Mee, E. W., Schweder, P. et al. (2021). Single-cell image analysis reveals a protective role for microglia in glioblastoma. Neuro-Oncology Advance, 3(1), vdab031. DOI 10.1093/noajnl/vdab031. [Google Scholar] [CrossRef]
85. Muller, S., Kohanbash, G., Liu, S. J., Alvarado, B., Carrera, D. et al. (2017). Single-cell profiling of human gliomas reveals macrophage ontogeny as a basis for regional differences in macrophage activation in the tumor microenvironment. Genome Biology, 18(1), 1–14. DOI 10.1186/s13059-017-1362-4. [Google Scholar] [CrossRef]
86. Garrido-Martin, E. M., Mellows, T. W. P., Clarke, J., Ganesan, A. P., Wood, O. et al. (2020). M1 hot tumor-associated macrophages boost tissue-resident memory T cells infiltration and survival in human lung cancer. Journal for Immunotherapy of Cancer, 8(2), e000778. DOI 10.1136/jitc-2020-000778. [Google Scholar] [CrossRef]
87. Liu, H., Sun, Y., Zhang, Q., Jin, W., Gordon, R. E. et al. (2021). Pro-inflammatory and proliferative microglia drive progression of glioblastoma. Cell Reports, 36(11), 109718. DOI 10.1016/j.celrep.2021.109718. [Google Scholar] [CrossRef]
88. Durham, N. M., Nirschl, C. J., Jackson, C. M., Elias, J., Kochel, C. M. et al. (2014). Lymphocyte activation gene 3 (LAG-3) modulates the ability of CD4 T-cells to be suppressed in vivo. PLoS One, 9(11), e109080. DOI 10.1371/journal.pone.0109080. [Google Scholar] [CrossRef]
89. Mahoney, K. M., Freeman, G. J., McDermott, D. F. (2015). The next immune-checkpoint inhibitors: PD-1/PD-L1 blockade in melanoma. Clinical Therapeutics, 37(4), 764–782. DOI 10.1016/j.clinthera.2015.02.018. [Google Scholar] [CrossRef]
90. Thaker, Y. R., Schneider, H., Rudd, C. E. (2015). TCR and CD28 activate the transcription factor NF-kappaB in T-cells via distinct adaptor signaling complexes. Immunology Letters, 163(1), 113–119. DOI 10.1016/j.imlet.2014.10.020. [Google Scholar] [CrossRef]
91. Gopalakrishnan, V., Spencer, C. N., Nezi, L., Reuben, A., Andrews, M. C. et al. (2018). Gut microbiome modulates response to anti-PD-1 immunotherapy in melanoma patients. Science, 359(6371), 97–103. DOI 10.1126/science.aan4236. [Google Scholar] [CrossRef]
92. DuPage, M., Cheung, A. F., Mazumdar, C., Winslow, M. M., Bronson, R. et al. (2011). Endogenous T cell responses to antigens expressed in lung adenocarcinomas delay malignant tumor progression. Cancer Cell, 19(1), 72–85. DOI 10.1016/j.ccr.2010.11.011. [Google Scholar] [CrossRef]
93. Pauken, K. E., Sammons, M. A., Odorizzi, P. M., Manne, S., Godec, J. et al. (2016). Epigenetic stability of exhausted T cells limits durability of reinvigoration by PD-1 blockade. Science, 354(6316), 1160–1165. DOI 10.1126/science.aaf2807. [Google Scholar] [CrossRef]
94. Andrews, M. C., Reuben, A., Gopalakrishnan, V., Wargo, J. A. (2018). Concepts collide: Genomic, immune, and microbial influences on the tumor microenvironment and response to cancer therapy. Frontiers in Immunology, 9, 946. DOI 10.3389/fimmu.2018.00946. [Google Scholar] [CrossRef]
95. Sadelain, M., Rivière, I., Brentjens, R. (2003). Targeting tumours with genetically enhanced T lymphocytes. Nature Reviews Cancer, 3(1), 35–45. DOI 10.1038/nrc971. [Google Scholar] [CrossRef]
96. Ikeda, H., (2016). T-cell adoptive immunotherapy using tumor-infiltrating T cells and genetically engineered TCR-T cells. International Immunology, 28(7), 349–353. DOI 10.1093/intimm/dxw022. [Google Scholar] [CrossRef]
97. Manfredi, F., Cianciotti, B. C., Potenza, A., Tassi, E., Noviello, M. et al. (2020). TCR redirected T cells for cancer treatment: Achievements, hurdles, and goals. Frontiers in Immunology, 11, 1689. DOI 10.3389/fimmu.2020.01689. [Google Scholar] [CrossRef]
98. Nerys-Junior, A., Braga-Dias, L. P., Pezzuto, P., Cotta-de-Almeida, V., Tanuri, A. (2018). Comparison of the editing patterns and editing efficiencies of TALEN and CRISPR-Cas9 when targeting the human CCR5 gene. Genetics and Molecular Biology, 41(1), 167–79. DOI 10.1590/1678-4685-gmb-2017-0065. [Google Scholar] [CrossRef]
99. Xia, A. L., He, Q. F., Wang, J. C., Zhu, J., Sha, Y. Q. et al. (2019). Applications and advances of CRISPR-Cas9 in cancer immunotherapy. Journal of Medical Genetics, 56(1), 4–9. DOI 10.1136/jmedgenet-2018-105422. [Google Scholar] [CrossRef]
100. Stadtmauer, E. A., Fraietta, J. A., Davis, M. M., Cohen, A. D., Weber, K. L. et al. (2020). CRISPR-engineered T cells in patients with refractory cancer. Science, 367(6481). DOI 10.1126/science.aba7365. [Google Scholar] [CrossRef]
101. Sunderland, M. W., Peggs, K. S. (2018). Successful translation and future prospects of TALEN editing for leukemia patients. Expert Opinion on Biological Therapy, 18(7), 725–726. DOI 10.1080/14712598.2018.1484105. [Google Scholar] [CrossRef]
102. Choi, B. D., Yu, X., Castano, A. P., Darr, H., Henderson, D. B. et al. (2019). CRISPR-Cas9 disruption of PD-1 enhances activity of universal EGFRvIII CAR T cells in a preclinical model of human glioblastoma. Journal for Immunotherapy Cancer, 7(1), 304. DOI 10.1186/s40425-019-0806-7. [Google Scholar] [CrossRef]
103. Wherry, E. J., Kurachi, M. (2015). Molecular and cellular insights into T cell exhaustion. Nature Reviews Immunology, 15(8), 486–499. DOI 10.1038/nri3862. [Google Scholar] [CrossRef]
104. Ansell, S. M., Lesokhin, A. M., Borrello, I., Halwani, A., Scott, E. C. et al. (2015). PD-1 blockade with nivolumab in relapsed or refractory hodgkin’s lymphoma. The New England Journal of Medicine, 372(4), 311–319. DOI 10.1056/NEJMoa1411087. [Google Scholar] [CrossRef]
105. Tawara, I., Kageyama, S., Miyahara, Y., Fujiwara, H., Nishida, T. et al. (2017). Safety and persistence of WT1-specific T-cell receptor gene−transduced lymphocytes in patients with AML and MDS. Blood, 130(18), 1985–1994. DOI 10.1182/blood-2017-06-791202. [Google Scholar] [CrossRef]
106. Park, J. H., Rivière, I., Gonen, M., Wang, X., Sénéchal, B. et al. (2018). Long-term follow-up of CD19 CAR therapy in acute lymphoblastic leukemia. The New England Journal of Medicine, 378(5), 449–459. DOI 10.1056/NEJMoa1709919. [Google Scholar] [CrossRef]
107. O’Rourke, D. M., Nasrallah, M. P., Desai, A., Melenhorst, J. J., Mansfield, K. et al. (2017). A single dose of peripherally infused EGFRvIII-directed CAR T cells mediates antigen loss and induces adaptive resistance in patients with recurrent glioblastoma. Science Translational Medicine, 9(399), eaaa0984. DOI 10.1126/scitranslmed.aaa0984. [Google Scholar] [CrossRef]
108. Tchou, J., Zhao, Y., Levine, B. L., Zhang, P. J., Davis, M. M. et al. (2017). Safety and efficacy of intratumoral injections of chimeric antigen receptor (CAR) T cells in metastatic breast cancer. Cancer Immunology Research, 5(12), 1152–1161. DOI 10.1158/2326-6066.CIR-17-0189. [Google Scholar] [CrossRef]
109. Holingue, C., Newill, C., Lee, L. C., Pasricha, P. J., Daniele Fallin, M. (2018). Gastrointestinal symptoms in autism spectrum disorder: A review of the literature on ascertainment and prevalence. Autism Research, 11(1), 24–36. DOI 10.1002/aur.1854. [Google Scholar] [CrossRef]
110. Du, W., Li, H., Sun, J., Xia, Y., Zhu, R. et al. (2018). The prognostic value of serum neuron specific enolase (NSE) and s100b level in patients of acute spinal cord injury. Medical Science Monitor, 24, 4510–4515. DOI 10.12659/MSM.907406. [Google Scholar] [CrossRef]
111. Orlando, D., Miele, E., de Angelis, B., Guercio, M., Boffa, I. et al. (2017). Adoptive immunotherapy using PRAME-specific T cells in medulloblastoma. Cancer Research, 78(12), 3337–3349. DOI 10.1158/0008-5472.CAN-17-3140. [Google Scholar] [CrossRef]
112. Rodríguez-Arco, L., Poma, A., Ruiz-Pérez, L., Scarpa, E., Ngamkham, K. (2019). Molecular bionics–engineering biomaterials at the molecular level using biological principles. Biomaterials, 192, 26–50. DOI 10.1016/j.biomaterials.2018.10.044. [Google Scholar] [CrossRef]
113. Bae, Y. H., Park, K. (2011). Targeted drug delivery to tumors: Myths, reality and possibility. Journal of Controlled Release, 153(3), 198–205. DOI 10.1016/j.jconrel.2011.06.001. [Google Scholar] [CrossRef]
114. Bangham, A. D., Horne, R. W. (1964). Negative staining of phospholipids and their structural modification by surface-active agents as observed in the electron microscope. Journal of Molecular Biology, 8(5), 660–668. DOI 10.1016/S0022-2836(64)80115-7. [Google Scholar] [CrossRef]
115. Wang, A. Z., Langer, R., Farokhzad, O. C. (2012). Nanoparticle delivery of cancer drugs. Annual Review of Medicine, 63, 185–198. DOI 10.1146/annurev-med-040210-162544. [Google Scholar] [CrossRef]
116. Karim, R., Palazzo, C., Evrard, B., Piel, G. (2016). Nanocarriers for the treatment of glioblastoma multiforme: Current state-of-the-art. Journal of Controlled Release, 227, 23–37. DOI 10.1016/j.jconrel.2016.02.026. [Google Scholar] [CrossRef]
117. Đorđević, S., Gonzalez, M. M., Conejos-Sánchez, I., Carreira, B., Pozzi, S. et al. (2022). Current hurdles to the translation of nanomedicines from bench to the clinic. Drug Delivery and Translational Research, 12(3), 500–525. DOI 10.1007/s13346-021-01024-2. [Google Scholar] [CrossRef]
118. Polack, F. P., Thomas, S. J., Kitchin, N., Absalon, J., Gurtman, A. et al. (2020). Safety and efficacy of the BNT162b2 mRNA COVID-19 vaccine. New England Journal of Medicine, 383(27), 2603–2615. DOI 10.1056/NEJMoa2034577. [Google Scholar] [CrossRef]
119. Mullard, A. (2021). Pfizer’s COVID-19 vaccine secures first full FDA approval. Nature Reviews. Drug Discovery, 20(10), 728. [Google Scholar]
120. Huang, S. K., Papahadjopoulos, D., Berk, D. A., Jain, R. K. (1994). Microvascular permeability and interstitial penetration of sterically stabilized (Stealth) liposomes in a human tumor xenograft. Cancer Research, 54(13), 3352–3356. [Google Scholar]
121. Ban, Z., Yuan, P., Yu, F., Peng, T., Zhou, Q. et al. (2020). Machine learning predicts the functional composition of the protein corona and the cellular recognition of nanoparticles. PNAS, 117(19), 10492–10499. DOI 10.1073/pnas.1919755117. [Google Scholar] [CrossRef]
122. Gomes-da-Silva, L. C., Fonseca, N. A., Moura, V., Pedroso de Lima, M. C., Simoes, S. et al. (2012). Lipid-based nanoparticles for siRNA delivery in cancer therapy: Paradigms and challenges. Accounts of Chemical Research, 45(7), 1163–1171. DOI 10.1021/ar300048p. [Google Scholar] [CrossRef]
123. Hong, M., Zhu, S., Jiang, Y., Tang, G., Pei, Y. (2009). Efficient tumor targeting of hydroxycamptothecin loaded PEGylated niosomes modified with transferrin. Journal of Controlled Release, 133(2), 96–102. DOI 10.1016/j.jconrel.2008.09.005. [Google Scholar] [CrossRef]
124. Ambegia, E., Ansell, S., Cullis, P., Heyes, J., Palmer, L. et al. (2005). Stabilized plasmid-lipid particles containing PEG-diacylglycerols exhibit extended circulation lifetimes and tumor selective gene expression. Biochimica et Biophysica Acta (BBA)-Biomembranes, 1669(2), 155–163. DOI 10.1016/j.bbamem.2005.02.001. [Google Scholar] [CrossRef]
125. Kang, H., Rho, S., Stiles, W. R., Hu, S., Baek, Y. et al. (2020). Size-dependent EPR effect of polymeric nanoparticles on tumor targeting. Advanced Healthcare Materials, 9(1), e1901223. DOI 10.1002/adhm.201901223. [Google Scholar] [CrossRef]
126. Hong, L., Wang, Z., Wei, X., Shi, J., Li, C. (2020). Antibodies against polyethylene glycol in human blood: A literature review. Journal of Pharmacological and Toxicological Methods, 102, 106678. DOI 10.1016/j.vascn.2020.106678. [Google Scholar] [CrossRef]
127. Leite, E. A., Souza, C. M., Carvalho-Junior, A. D., Coelho, L. G., Lana, A. M. et al. (2012). Encapsulation of cisplatin in long-circulating and pH-sensitive liposomes improves its antitumor effect and reduces acute toxicity. International Journal of Nanomedicine, 7, 5259–5269. [Google Scholar]
128. Kocere, A., Resseguier, J., Wohlmann, J., Skjeldal, F. M., Khan, S. et al. (2020). Real-time imaging of polymersome nanoparticles in zebrafish embryos engrafted with melanoma cancer cells: Localization, toxicity and treatment analysis. eBioMedicine, 58, 102902. DOI 10.1016/j.ebiom.2020.102902. [Google Scholar] [CrossRef]
129. Acosta-Gutiérrez, S., Matias, D., Avila-Olias, M., Gouveia, V. M., Scarpa, E. et al. (2022). A multiscale study of phosphorylcholine driven cellular phenotypic targeting. ACS Central Science, 8(7), 891–904. DOI 10.1021/acscentsci.2c00146. [Google Scholar] [CrossRef]
130. Tian, X., Leite, D. M., Scarpa, E., Nyberg, S., Fullstone, G. et al. (2020). On the shuttling across the blood-brain barrier via tubule formation: Mechanism and cargo avidity bias. Science Adventure Series, 6(48), eabc4397. DOI 10.1126/sciadv.abc4397. [Google Scholar] [CrossRef]
131. He, C., Zhang, Z., Ding, Y., Xue, K., Wang, X. et al. (2021). LRP1-mediated pH-sensitive polymersomes facilitate combination therapy of glioblastoma in vitro and in vivo. Journal of Nanobiotechnology, 19(1), 19. DOI 10.1186/s12951-020-00751-x. [Google Scholar] [CrossRef]
132. Keefe, S. M., Hoffman-Censits, J., Cohen, R. B., Mamtani, R., Heitjan, D. et al. (2016). Efficacy of the nanoparticle-drug conjugate CRLX101 in combination with bevacizumab in metastatic renal cell carcinoma: Results of an investigator-initiated phase I-IIa clinical trial. Annals of Oncology, The Journal of the European Society for Medical Oncology, 27(8), 1579–1585. DOI 10.1093/annonc/mdw188. [Google Scholar] [CrossRef]
133. Dennis, M., Davies, M., Oliver, S., D’Souza, R., Pike, L. et al. (2012). Phase I study of the aurora B kinase inhibitor barasertib (AZD1152) to assess the pharmacokinetics, metabolism and excretion in patients with acute myeloid leukemia. Cancer Chemotherapy and Pharmacology, 70(3), 461–469. DOI 10.1007/s00280-012-1939-2. [Google Scholar] [CrossRef]
134. He, Z., Wan, X., Schulz, A., Bludau, H., Dobrovolskaia, M. A. et al. (2016). A high capacity polymeric micelle of paclitaxel: Implication of high dose drug therapy to safety and in vivo anti-cancer activity. Biomaterials, 101, 296–309. DOI 10.1016/j.biomaterials.2016.06.002. [Google Scholar] [CrossRef]
135. Hamaguchi, T., Matsumura, Y., Suzuki, M., Shimizu, K., Goda, R. et al. (2005). NK105, a paclitaxel-incorporating micellar nanoparticle formulation, can extend in vivo antitumour activity and reduce the neurotoxicity of paclitaxel. British Journal of Cancer, 92(7), 1240–1246. DOI 10.1038/sj.bjc.6602479. [Google Scholar] [CrossRef]
136. Barenholz, Y. C. (2012). Doxil®—The first FDA-approved nano-drug: Lessons learned. Journal of Controlled Release, 160(2), 117–134. DOI 10.1016/j.jconrel.2012.03.020. [Google Scholar] [CrossRef]
137. Ingram, I. (2011). FDA approves brentuximab vedotin for hodgkin lymphoma and systemic anaplastic large-cell lymphoma. Oncology, 25(10), 904. [Google Scholar]
138. Rivankar, S. (2014). An overview of doxorubicin formulations in cancer therapy. Journal of Cancer Research and Therapeutics, 2014(10), 853–858. DOI 10.4103/0973-1482.139267. [Google Scholar] [CrossRef]
139. Silverman, J. A., Deitcher, S. R. (2013). Marqibo® (vincristine sulfate liposome injection) improves the pharmacokinetics and pharmacodynamics of vincristine. Cancer Chemotherapy and Pharmacology, 71(3), 555–564. DOI 10.1007/s00280-012-2042-4. [Google Scholar] [CrossRef]
140. Passero, F. C., Grapsa, D., Syrigos, K. N., Saif, M. W. (2016). The safety and efficacy of onivyde (irinotecan liposome injection) for the treatment of metastatic pancreatic cancer following gemcitabine-based therapy.Expert Review of Anticancer Therapy, 16(7), 697–703. DOI 10.1080/14737140.2016.1192471. [Google Scholar] [CrossRef]
141. Wurz, G. T., Kao, C. J., Wolf, M., DeGregorio, M. W. (2014). Tecemotide: An antigen-specific cancer immunotherapy. Human Vaccines & Immunotherapeutics, 10(11), 3383–3393. DOI 10.4161/hv.29836. [Google Scholar] [CrossRef]
142. Hong, C. W., Libutti, S. K., Wood, B. J. (2013). Liposomal doxorubicin plus radiofrequency ablation for complete necrosis of a hepatocellular carcinoma. Current Oncology, 20(3), 274–277. DOI 10.3747/co.20.1266. [Google Scholar] [CrossRef]
143. Boulikas, T. (2009). Clinical overview on lipoplatinTM : A successful liposomal formulation of cisplatin. Expert Opinion on Investigational Drugs, 18(8), 1197–1218. DOI 10.1517/13543780903114168. [Google Scholar] [CrossRef]
144. Dragovich, T., Mendelson, D., Kurtin, S., Richardson, K., von Hoff, D. et al. (2006). A phase 2 trial of the liposomal DACH platinum L-NDDP in patients with therapy-refractory advanced colorectal cancer. Cancer Chemotherapy and Pharmacology, 58(6), 759–764. DOI 10.1007/s00280-006-0235-4. [Google Scholar] [CrossRef]
145. Seetharamu, N., Kim, E., Hochster, H., Martin, F., Muggia, F. (2010). Phase II study of liposomal cisplatin (SPI-77) in platinum-sensitive recurrences of ovarian cancer. Anticancer Research, 30(2), 541–545. [Google Scholar]
146. Boraschi, D., Italiani, P., Palomba, R., Decuzzi, P., Duschl, A. et al. (2017). Nanoparticles and innate immunity: New perspectives on host defence. Seminars in Immunology, 34, 33–51. DOI 10.1016/j.smim.2017.08.013. [Google Scholar] [CrossRef]
147. Kishimoto, T. K., Maldonado, R. A. (2018). Nanoparticles for the induction of antigen-specific immunological tolerance. Frontiers in Immunology, 9, 230. DOI 10.3389/fimmu.2018.00230. [Google Scholar] [CrossRef]
148. Oya, M. (1994). Antitumor effect of interleukin-2 entrapped in liposomes on murine renal cell carcinoma. The Keio Journal of Medicine, 43(1), 37–44. DOI 10.2302/kjm.43.37. [Google Scholar] [CrossRef]
149. Yao, H., Ng, S. S., Huo, L. F., Chow, B. K. C., Shen, Z. et al. (2011). Effective melanoma immunotherapy with interleukin-2 delivered by a novel polymeric nanoparticle. Molecular Cancer Therapeutics, 10(6), 1082–1092. DOI 10.1158/1535-7163.MCT-10-0717. [Google Scholar] [CrossRef]
150. Boada, C., Zinger, A., Tsao, C., Zhao, P., Martinez, J. O. et al. (2020). Rapamycin-loaded biomimetic nanoparticles reverse vascular inflammation. Circulation Research, 126(1), 25–37. DOI 10.1161/CIRCRESAHA.119.315185. [Google Scholar] [CrossRef]
151. Yin, Y., Hu, Q., Xu, C., Qiao, Q., Qin, X. et al. (2018). Co-delivery of doxorubicin and interferon-gamma by thermosensitive nanoparticles for cancer immunochemotherapy. Molecular Pharmacology, 15(9), 4161–4172. DOI 10.1021/acs.molpharmaceut.8b00564. [Google Scholar] [CrossRef]
152. Fang, R. H., Zhang, L. (2016). Nanoparticle-based modulation of the immune system. Annual Review of Chemical and Biomolecular Engineering, 7, 305–326. DOI 10.1146/annurev-chembioeng-080615-034446. [Google Scholar] [CrossRef]
153. Zimel, M. N., Horowitz, C. B., Rajasekhar, V. K., Christ, A. B., Wei, X. et al. (2017). HPMA-copolymer nanocarrier targets tumor-associated macrophages in primary and metastatic breast cancer. Molecular Cancer Therapeutics, 16(12), 2701–2710. DOI 10.1158/1535-7163.MCT-15-0995. [Google Scholar] [CrossRef]
154. Anselmo, A. C., Gokarn, Y., Mitragotri, S. (2018). Non-invasive delivery strategies for biologics. Nature Reviews Drug Discovery, 18(1), 19–40. DOI 10.1038/nrd.2018.183. [Google Scholar] [CrossRef]
155. Norouzi, M., Nazari, B., Miller, D. W. (2016). Injectable hydrogel-based drug delivery systems for local cancer therapy. Drug Discovery Today, 21(11), 1835–1849. DOI 10.1016/j.drudis.2016.07.006. [Google Scholar] [CrossRef]
156. Shukla, A., Singh, A. P., Maiti, P. (2021). Injectable hydrogels of newly designed brush biopolymers as sustained drug-delivery vehicle for melanoma treatment. Signal Transduction and Targeted Therapy, 6(1), 63. DOI 10.1038/s41392-020-00431-0. [Google Scholar] [CrossRef]
157. Zhang, J., Chen, C., Li, A., Jing, W., Sun, P. et al. (2021). Immunostimulant hydrogel for the inhibition of malignant glioma relapse post-resection. Nature Nanotechnology, 16(5), 538–548. DOI 10.1038/s41565-020-00843-7. [Google Scholar] [CrossRef]
158. Vishnubhakthula, S., Elupula, R., Durán-Lara, E. F. (2017). Recent advances in hydrogel-based drug delivery for melanoma cancer therapy: A mini review. Journal of Drug Delivery, 2017, 7275985. DOI 10.1155/2017/7275985. [Google Scholar] [CrossRef]
159. Liu, J., Qi, C., Tao, K., Zhang, J., Zhang, J. et al. (2016). Sericin/Dextran injectable hydrogel as an optically trackable drug delivery system for malignant melanoma treatment. ACS Applied Materials & Interfaces, 8(10), 6411–6422. DOI 10.1021/acsami.6b00959. [Google Scholar] [CrossRef]
160. Jahanban-Esfahlan, R., Derakhshankhah, H., Haghshenas, B., Massoumi, B., Abbasian, M. et al. (2020). Bio-inspired magnetic natural hydrogel containing gelatin and alginate as a drug delivery system for cancer chemotherapy. International Journal of Biological Macromolecules, 156, 438–445. DOI 10.1016/j.ijbiomac.2020.04.074. [Google Scholar] [CrossRef]
161. Xue, B., Wang, W., Qin, J. J., Nijampatnam, B., Murugesan, S. et al. (2017). Highly efficient delivery of potent anticancer iminoquinone derivative by multilayer hydrogel cubes. Acta Biomaterialia, 58, 386–398. DOI 10.1016/j.actbio.2017.06.004. [Google Scholar] [CrossRef]
162. Mandal, A., Clegg, J. R., Anselmo, A. C., Mitragotri, S. (2020). Hydrogels in the clinic. Bioengineering & Translational Medicine, 5(2), e10158. DOI 10.1002/btm2.10158. [Google Scholar] [CrossRef]
163. Hyun, H., Park, M. H., Lim, W., Kim, S. Y., Jo, D. et al. (2018). Injectable visible light-cured glycol chitosan hydrogels with controlled release of anticancer drugs for local cancer therapy in vivo: A feasible study. Artificial Cells, Nanomedicine, and Biotechnology, 46(sup2), 874–882. DOI 10.1080/21691401.2018.1470529. [Google Scholar] [CrossRef]
164. Liu, X., Li, Z., Loh, X. J., Chen, K., Li, Z. et al. (2018). Targeted and sustained corelease of chemotherapeutics and gene by injectable supramolecular hydrogel for drug-resistant cancer therapy. Macromolecular Rapid Communications, 40(5), e1800117. DOI 10.1002/marc.201800117. [Google Scholar] [CrossRef]
165. Ribeiro, V. P., Silva-Correia, J., Gonçalves, C., Pina, S., Radhouani, H. et al. (2018). Rapidly responsive silk fibroin hydrogels as an artificial matrix for the programmed tumor cells death. PLoS One, 13(4), e0194441. DOI 10.1371/journal.pone.0194441. [Google Scholar] [CrossRef]
166. Chiavenna, S. M., Jaworski, J. P., Vendrell, A. (2017). State of the art in anti-cancer mAbs. Journal of Biomedical Science, 24(1), 15. DOI 10.1186/s12929-016-0311-y. [Google Scholar] [CrossRef]
167. Donaghy, H. (2016). Effects of antibody, drug and linker on the preclinical and clinical toxicities of antibody-drug conjugates. mAbs, 8(4), 659–671. DOI 10.1080/19420862.2016.1156829. [Google Scholar] [CrossRef]
168. García-Alonso, S., Ocaña, A., Pandiella, A. (2018). Resistance to antibody–drug conjugates. Cancer Research, 78(9), 2159–2165. DOI 10.1158/0008-5472.CAN-17-3671. [Google Scholar] [CrossRef]
169. Ponziani, S., di Vittorio, G., Pitari, G., Cimini, A. M., Ardini, M. et al. (2020). Antibody-drug conjugates: The new frontier of chemotherapy. International Journal of Molecular Sciences, 21(15), 5510. DOI 10.3390/ijms21155510. [Google Scholar] [CrossRef]
170. Chen, B., Chen, R. (2015). Brentuximab vedotin for relapsed or refractory hodgkin’s lymphoma. Drug Design, Development and Therapy, 9, 1729–1733. [Google Scholar]
171. Heist, R. S., Guarino, M. J., Masters, G., Purcell, W. T., Starodub, A. N. et al. (2017). Therapy of advanced non–small-cell lung cancer with an SN-38-anti-trop-2 drug conjugate, sacituzumab govitecan. Journal of Clinical Oncology, 35(24), 2790–2797. DOI 10.1200/JCO.2016.72.1894. [Google Scholar] [CrossRef]
172. Peyser, N. D., Grandis, J. R. (2017). Cancer genomics: Spot the difference. Nature, 541(7636), 162–163. DOI 10.1038/nature21112. [Google Scholar] [CrossRef]
173. Pećina-Šlaus, N., Pećina, M. (2015). Only one health, and so many omics. Cancer Cell International, 15, 64. DOI 10.1186/s12935-015-0212-2. [Google Scholar] [CrossRef]
174. Schneider, M. V., Orchard, S. (2011). Omics technologies, data and bioinformatics principles. In: Methods in molecular biology, vol. 719. Humana Press. DOI 10.1007/978-1-61779-027-0_1. [Google Scholar] [CrossRef]
175. Leithner, D., Horvat, J. V., Ochoa-Albiztegui, R. E., Thakur, S., Wengert, G. et al. (2018). Imaging and the completion of the omics paradigm in breast cancer. Radiologe, 58(Suppl 1), 7–13. DOI 10.1007/s00117-018-0409-1. [Google Scholar] [CrossRef]
176. Rodrigues, L. F., Moura-Neto, V., Spohr, T. (2018). Biomarkers in spinal cord injury: From prognosis to treatment. Molecular Neurobiology, 55(8), 6436–6448. DOI 10.1007/s12035-017-0858-y. [Google Scholar] [CrossRef]
177. Dunn, B. K., Meerzaman, D. (2020). Editorial: Using cancer “Omics” to understand cancer. Frontiers in Oncology, 10, 1201. DOI 10.3389/fonc.2020.01201. [Google Scholar] [CrossRef]
178. Patel, L., Parker, B., Yang, D., Zhang, W. (2013). Translational genomics in cancer research: Converting profiles into personalized cancer medicine. Cancer Biology & Medicine, 10(4), 214–220. [Google Scholar]
179. Scherer, A., Christensen, G. B. (2016). Concepts and relevance of genome-wide association studies. Science Progress, 99, 59–67. DOI 10.3184/003685016X14558068452913. [Google Scholar] [CrossRef]
180. Schultz, D. J., Krishna, A., Vittitow, S. L., Alizadeh-Rad, N., Muluhngwi, P. et al. (2018). Transcriptomic response of breast cancer cells to anacardic acid. Scientific Reports, 8(1), 8063. DOI 10.1038/s41598-018-26429-x. [Google Scholar] [CrossRef]
181. Chang, S. J., Liao, E. C., Yeo, H. Y., Kuo, W. H., Chen, H. Y. et al. (2018). Proteomic investigating the cooperative lethal effect of EGFR and MDM2 inhibitors on ovarian carcinoma. Archives of Biochemistry and Biophysics, 647, 10–32. DOI 10.1016/j.abb.2018.04.004. [Google Scholar] [CrossRef]
182. Kume, H., Muraoka, S., Kuga, T., Adachi, J., Narumi, R. et al. (2014). Discovery of colorectal cancer biomarker candidates by membrane proteomic analysis and subsequent verification using selected reaction monitoring (SRM) and tissue microarray (TMA) analysis. Molecular & Cellular Proteomics, 13(6), 1471–1484. DOI 10.1074/mcp.M113.037093. [Google Scholar] [CrossRef]
183. Jones, P. A., Baylin, S. B. (2007). The epigenomics of cancer. Cell, 128, 683–692. DOI 10.1016/j.cell.2007.01.029. [Google Scholar] [CrossRef]
184. Ehrlich, M., Lacey, M. (2013). DNA hypomethylation and hemimethylation in cancer. Advances in Experimental Medicine and Biology, 754, 31–56. DOI 10.1007/978-1-4419-9967-2. [Google Scholar] [CrossRef]
185. Lindsay, C., Seikaly, H., Biron, V. L. (2017). Epigenetics of oropharyngeal squamous cell carcinoma: Opportunities for novel chemotherapeutic targets. Journal of Otolaryngology-Head & Neck Surgery, 46(1), 1–10. DOI 10.1186/s40463-017-0185-3. [Google Scholar] [CrossRef]
186. Chen, L. H., Pan, C., Diplas, B. H., Xu, C., Hansen, L. J. et al. (2020). The integrated genomic and epigenomic landscape of brainstem glioma. Nature Communications, 11(1), 3077. DOI 10.1038/s41467-020-16682-y. [Google Scholar] [CrossRef]
187. Gasche, J. A., Goel, A. (2012). Epigenetic mechanisms in oral carcinogenesis. Future Oncology, 8(11), 1407–1425. DOI 10.2217/fon.12.138. [Google Scholar] [CrossRef]
188. Yoshida, T., Kates, M., Sopko, N. A., Liu, X., Singh, A. K. et al. (2018). Ex vivo culture of tumor cells from N-methyl-N-nitrosourea–induced bladder cancer in rats: Development of organoids and an immortalized cell line. Urologic Oncology: Seminars and Original Investigations, 36(4), 160.e23–160.e32. DOI 10.1016/j.urolonc.2017.11.024. [Google Scholar] [CrossRef]
189. Perrotti, F., Rosa, C., Cicalini, I., Sacchetta, P., Del Boccio, P. et al. (2016). Advances in lipidomics for cancer biomarkers discovery. International Journal of Molecular Sciences, 17(12), 1992. DOI 10.3390/ijms17121992. [Google Scholar] [CrossRef]
190. Lv, J., Gao, D., Zhang, Y., Wu, D., Shen, L. et al. (2018). Heterogeneity of lipidomic profiles among lung cancer subtypes of patients. Journal of Cellular and Molecular Medicine, 22(10), 5155–5159. DOI 10.1111/jcmm.13782. [Google Scholar] [CrossRef]
191. Braicu, E., Darb-Esfahani, S., Schmitt, W. D., Koistinen, K. M., Heiskanen, L. et al. (2017). High-grade ovarian serous carcinoma patients exhibit profound alterations in lipid metabolism. Oncotarget, 8(61), 102912–102922. DOI 10.18632/oncotarget.22076. [Google Scholar] [CrossRef]
192. Roy, J., Dibaeinia, P., Fan, T. M., Sinha, S., Das, A. (2019). Global analysis of osteosarcoma lipidomes reveal altered lipid profiles in metastatic versus nonmetastatic cells. Journal of Lipid Research, 60(2), 375–387. DOI 10.1194/jlr.M088559. [Google Scholar] [CrossRef]
193. Mathé, E., Hays, J. L., Stover, D. G., Chen, J. L. (2018). The omics revolution continues: The maturation of high-throughput biological data sources. Yearbook of Medical Informatics, 27(1), 211–222. DOI 10.1055/s-0038-1667085. [Google Scholar] [CrossRef]
194. Wu, J. Y., Huang, T. W., Hsieh, Y. T., Wang, Y. F., Yen, C. C. et al. (2020). Cancer-derived succinate promotes macrophage polarization and cancer metastasis via succinate receptor. Molecular Cell, 77(2), 213–227.e5. DOI 10.1016/j.molcel.2019.10.023. [Google Scholar] [CrossRef]
195. Tian, Y., Xu, T., Huang, J., Zhang, L., Xu, S. et al. (2016). Tissue metabonomic phenotyping for diagnosis and prognosis of human colorectal cancer. Scientific Reports, 6, 20790. DOI 10.1038/srep20790. [Google Scholar] [CrossRef]
196. Chen, X., Zhao, C., Zhao, Z., Wang, H., Fang, Z. (2019). Specific glioma prognostic subtype distinctions based on DNA methylation patterns. Frontiers in Genetics, 10, 786. DOI 10.3389/fgene.2019.00786. [Google Scholar] [CrossRef]
197. Yao, Z., Han, L., Chen, Y., He, F., Sun, B. et al. (2018). Hedgehog signalling in the tumourigenesis and metastasis of osteosarcoma, and its potential value in the clinical therapy of osteosarcoma review. Cell Death & Disease, 9(6), 701. DOI 10.1038/s41419-018-0647-1. [Google Scholar] [CrossRef]
198. Negm, R. S., Verma, M., Srivastava, S. (2002). The promise of biomarkers in cancer screening and detection. Trends in Molecular Medicine, 8(6), 288–293. DOI 10.1016/S1471-4914(02)02353-5. [Google Scholar] [CrossRef]
199. Dumbrava, E. I., Meric-Bernstam, F., Yap, T. A. (2018). Challenges with biomarkers in cancer drug discovery and development. Expert Opinion on Drug Discovery, 13(8), 685–690. DOI 10.1080/17460441.2018.1479740. [Google Scholar] [CrossRef]
200. Hoseok, I., Cho, J. Y. (2015). Lung cancer biomarkers. Advances in Clinical Chemistry, 72, 107–170. DOI 10.1016/bs.acc.2015.07.003. [Google Scholar] [CrossRef]
201. Henry, N. L., Hayes, D. F. (2012). Cancer biomarkers. Molecular Oncology, 6, 140–146. DOI 10.1016/j.molonc.2012.01.010. [Google Scholar] [CrossRef]
202. Nair, M., Sandhu, S. S., Sharma, A. K. (2014). Prognostic and predictive biomarkers in cancer. Current Cancer Drug Targets, 14(5), 477–504. DOI 10.2174/1568009614666140506111118. [Google Scholar] [CrossRef]
203. Goossens, N., Nakagawa, S., Sun, X., Hoshida, Y. (2015). Cancer biomarker discovery and validation. Translational Cancer Research, 4(3), 256–269. [Google Scholar]
204. Gold, P., Freedman, S. O. (1965). Demonstration of tumor-specific antigens in human colonic carcinomata by immunological tolerance and absorption techniques. Journal of Experimental Medicine, 121(3), 439–462. DOI 10.1084/jem.121.3.439. [Google Scholar] [CrossRef]
205. Chatterjee, S. K., Zetter, B. R. (2005). Cancer biomarkers: Knowing the present and predicting the future. Future Oncology, 1(1), 37–50. DOI 10.1517/14796694.1.1.37. [Google Scholar] [CrossRef]
206. Scholler, N., Garvik, B., Hayden-Ledbetter, M., Kline, T., Urban, N. (2007). Development of a CA125-mesothelin cell adhesion assay as a screening tool for biologics discovery. Cancer Letter, 247(1), 130–136 DOI 10.1016/j.canlet.2006.03.029. [Google Scholar] [CrossRef]
207. Kim, S., Park, B. K., Seo, J. H., Choi, J., Choi, J. W. et al. (2020). Carbohydrate antigen 19–9 elevation without evidence of malignant or pancreatobiliary diseases. Scientific Reports, 10(1), 8820. DOI 10.1038/s41598-020-65720-8. [Google Scholar] [CrossRef]
208. Lamy, P. J., Allory, Y., Gauchez, A. S., Asselain, B., Beuzeboc, P. et al. (2018). Prognostic biomarkers used for localised prostate cancer management: A systematic review. European Urology Focus, 4(6), 790–803. DOI 10.1016/j.euf.2017.02.017. [Google Scholar] [CrossRef]
209. Lemańska-Perek, A., Lis-Kuberka, J., Lepczyński, A., Dratwa-Chałupnik, A., Tupikowski, K. et al. (2019). Potential plasma biomarkers of bladder cancer identified by proteomic analysis: A pilot study. Advances in Clinical and Experimental Medicine, 28(3), 339–346. DOI 10.17219/acem/79296. [Google Scholar] [CrossRef]
210. Hanash, S. M., Baik, C. S., Kallioniemi, O. (2011). Emerging molecular biomarkers--blood-based strategies to detect and monitor cancer. Nature Reviews Clinical Oncology, 8(3), 142–150. DOI 10.1038/nrclinonc.2010.220. [Google Scholar] [CrossRef]
211. Maemondo, M., Inoue, A., Kobayashi, K., Sugawara, S., Oizumi, S. et al. (2010). Gefitinib or chemotherapy for non-small-cell lung cancer with mutated EGFR. New England Journal of Medicine, 362(25), 2380–2388. DOI 10.1056/NEJMoa0909530. [Google Scholar] [CrossRef]
212. Kalia, M. (2013). Personalized oncology: Recent advances and future challenges. Metabolism, 62 (Suppl 1), S11–14. DOI 10.1016/j.metabol.2012.08.016. [Google Scholar] [CrossRef]
213. Daly, M. B., Pilarski, R., Berry, M., Buys, S. S., Farmer, M. et al. (2017). NCCN guidelines insights: Genetic/Familial high-risk assessment: Breast and ovarian, version 2.2017. Journal of the National Comprehensive Cancer Network, 15(1), 9–20. DOI 10.6004/jnccn.2017.0003. [Google Scholar] [CrossRef]
214. Hench, I. B., Hench, J., Tolnay, M. (2018). Liquid biopsy in clinical management of breast, lung, and colorectal cancer. Frontiers in Medicine, 5, 9. DOI 10.3389/fmed.2018.00009. [Google Scholar] [CrossRef]
215. Siravegna, G., Marsoni, S., Siena, S., Bardelli, A. (2017). Integrating liquid biopsies into the management of cancer. Nature Reviews Clinical Oncology, 14(9), 531–548. DOI 10.1038/nrclinonc.2017.14. [Google Scholar] [CrossRef]
216. Pessoa, L. S., Heringer, M., Ferrer, V. P. (2020). ctDNA as a cancer biomarker: A broad overview. Critical Reviews in Oncology/Hematology, 155, 103109. DOI 10.1016/j.critrevonc.2020.103109. [Google Scholar] [CrossRef]
217. Wan, J. C. M., Massie, C., Garcia-Corbacho, J., Mouliere, F., Brenton, J. D. et al. (2017). Liquid biopsies come of age: Towards implementation of circulating tumour DNA. Nature Reviews Cancer, 17(4), 223–238. DOI 10.1038/nrc.2017.7. [Google Scholar] [CrossRef]
218. Hanahan, D., Weinberg, R. A. (2011). Hallmarks of cancer: The next generation. Cell, 144(5), 646–674. DOI 10.1016/j.cell.2011.02.013. [Google Scholar] [CrossRef]
219. Ullah, M. F., Aatif, M. (2009). The footprints of cancer development: Cancer biomarkers. Cancer Treatment Reviews, 35(3), 193–200. DOI 10.1016/j.ctrv.2008.10.004. [Google Scholar] [CrossRef]
220. Sarhadi, V. K., Armengol, G. (2022). Molecular biomarkers in cancer. Biomolecules, 12(8), 1021. DOI 10.3390/biom12081021. [Google Scholar] [CrossRef]
221. Chen, Y. L., Chen, W. L., Cheng, Y. C., Lin, M. C., Yang, S. C. et al. (2021). Development of a novel ALK rearrangement screening test for non-small cell lung cancers. PLoS One, 16(9), e0257152. DOI 10.1371/journal.pone.0257152. [Google Scholar] [CrossRef]
222. De Bono, J. S., Scher, H. I., Montgomery, R. B., Parker, C., Miller, M. C. et al. (2008). Circulating tumor cells predict survival benefit from treatment in metastatic castration-resistant prostate cancer. Clinical Cancer Research, 14(19), 6302–6309. [Google Scholar]
223. Leighl, N. B., Page, R. D., Raymond, V. M., Daniel, D. B., Divers, S. G. et al. (2019). Clinical utility of comprehensive cell-free DNA analysis to identify genomic biomarkers in patients with newly diagnosed metastatic non-small cell lung cancer. Clinical Cancer Research, 25(15), 4691–700. DOI 10.1158/1078-0432.CCR-19-0624. [Google Scholar] [CrossRef]
224. Dankner, M., Rose, A. A. N., Rajkumar, S., Siegel, P. M., Watson, I. R. (2018). Classifying BRAF alterations in cancer: New rational therapeutic strategies for actionable mutations. Oncogene, 37(24), 3183–3199. DOI 10.1038/s41388-018-0171-x. [Google Scholar] [CrossRef]
225. Shao, C., Chang, M. S., Lam, F. C., Marley, A. R., Tang, H. et al. (2022). A systematic review and meta-analysis on the prognostic value of BRCA mutations, homologous recombination gene mutations, and homologous recombination deficiencies in cancer. Journal of Oncology, 2022, 1–12. DOI 10.1155/2022/5830475. [Google Scholar] [CrossRef]
226. Kaźmierczak, D., Eide, I. J. Z., Gencheva, R., Lai, Y., Lewensohn, R. et al. (2022). Elevated expression of miR-494-3p is associated with resistance to osimertinib in EGFR T790M-positive non-small cell lung cancer. Translational Lung Cancer Research, 11(5), 722–734. DOI 10.21037/tlcr. [Google Scholar] [CrossRef]
227. Luo, R., Liu, H., Chen, J. (2021). Reduced circulating exosomal miR-382 predicts unfavorable outcome in non-small cell lung cancer. International Journal of Clinical and Experimental Pathology, 14(4), 469–474. [Google Scholar]
228. Liu, M., Mo, F., Song, X., He, Y., Yuan, Y. et al. (2021). Exosomal hsa-miR-21-5p is a biomarker for breast cancer diagnosis. PeerJ, 9, e12147. DOI 10.7717/peerj.12147. [Google Scholar] [CrossRef]
229. Rode, M. P., Silva, A. H., Cisilotto, J., Rosolen, D., Creczynski-Pasa, T. B. (2021). miR-425-5p as an exosomal biomarker for metastatic prostate cancer. Cell Signaling, 87, 110113. DOI 10.1016/j.cellsig.2021.110113. [Google Scholar] [CrossRef]
230. Bartoszewski, R., Sikorski, A. F. (2018). Editorial focus: Entering into the non-coding RNA era. Cellular & Molecular Biology Letters, 23, 45. DOI 10.1186/s11658-018-0111-3. [Google Scholar] [CrossRef]
231. Gros, F. (2012). Les mondes nouveau de la biologie. Odile Jacob Science. [Google Scholar]
232. Bartel, D. P. (2018). Metazoan microRNAs. Cell, 173, 20–51. DOI 10.1016/j.cell.2018.03.006. [Google Scholar] [CrossRef]
233. Pasquinelli, A. E. (2012). MicroRNAs and their targets: Recognition, regulation and an emerging reciprocal relationship. Nature Reviews Genetics, 13(4), 271–282. DOI 10.1038/nrg3162. [Google Scholar] [CrossRef]
234. Gennarino, V. A., D’Angelo, G., Dharmalingam, G., Fernandez, S., Russolillo, G. et al. (2012). Identification of microRNA-regulated gene networks by expression analysis of target genes. Genome Research, 22(6), 1163–1172. DOI 10.1101/gr.130435.111. [Google Scholar] [CrossRef]
235. Rupaimoole, R., Slack, F. J. (2017). MicroRNA therapeutics: Towards a new era for the management of cancer and other diseases. Nature Reviews Drug Discovery, 16(3), 203–222. DOI 10.1038/nrd.2016.246. [Google Scholar] [CrossRef]
236. Gebeshuber, C. A., Zatloukal, K., Martinez, J. (2009). miR-29a suppresses tristetraprolin, which is a regulator of epithelial polarity and metastasis. EMBO Reports, 10(4), 400–405. DOI 10.1038/embor.2009.9. [Google Scholar] [CrossRef]
237. Majid, S., Dar, A. A., Saini, S., Deng, G., Chang, I. et al. (2013). MicroRNA-23b functions as a tumor suppressor by regulating Zeb1 in bladder cancer. PLoS One, 8(7), e67686. DOI 10.1371/journal.pone.0067686. [Google Scholar] [CrossRef]
238. Zaman, M. S., Thamminana, S., Shahryari, V., Chiyomaru, T., Deng, G. et al. (2012). Inhibition of PTEN gene expression by oncogenic miR-23b-3p in renal cancer. PLoS One, 7(11), e50203. DOI 10.1371/journal.pone.0050203. [Google Scholar] [CrossRef]
239. Balça-Silva, J., Matias, D., Do Carmo, A., Dubois, L. G., Gonçalves, A. C. et al. (2017). Glioblastoma entities express subtle differences in molecular composition and response to treatment. Oncology Reports, 38(3), 1341–1352. DOI 10.3892/or.2017.5799. [Google Scholar] [CrossRef]
240. Rizzo, S., Cangemi, A., Galvano, A., Fanale, D., Buscemi, S. et al. (2017). Analysis of miRNA expression profile induced by short term starvation in breast cancer cells treated with doxorubicin. Oncotarget, 8(42), 71924–71932. DOI 10.18632/oncotarget.18028. [Google Scholar] [CrossRef]
241. Lindholm, E. M., Leivonen, S. K., Undlien, E., Nebdal, D., Git, A. et al. (2018). miR-342-5p as a potential regulator of HER2 breast cancer cell growth. MicroRNA, 8(2), 155–165. DOI 10.2174/2211536608666181206124922. [Google Scholar] [CrossRef]
242. Gil, J., Laczmanska, I., Pesz, K. A., Sasiadek, M. M. (2018). Personalized medicine in oncology. New Perspectives in Management of Gliomas. Wspolczesna Onkologia-Contemporary Oncology, 22(1A), 1–2. [Google Scholar]
243. Kammesheidt, A., Tonozzi, T. R., Lim, S. W., Braunstein, G. D. (2018). Mutation detection using plasma circulating tumor DNA (ctDNA) in a cohort of asymptomatic adults at increased risk for cancer. International Journal of Molecular Epidemiology and Genetics, 9(1), 1–12. [Google Scholar]
244. Porter, D. L., Hwang, W. T., Frey, N. V., Lacey, S. F., Shaw, P. A. et al. (2015). Chimeric antigen receptor T cells persist and induce sustained remissions in relapsed refractory chronic lymphocytic leukemia. Science Translational Medicine, 7(303), 303ra139. DOI 10.1126/scitranslmed.aac5415. [Google Scholar] [CrossRef]
245. Tiefenbacher, A., Pirker, R. (2017). EGFR tyrosine kinase inhibitors as first-line therapy in advanced EGFR mutation-positive non-small cell lung cancer: Strategies to improve clinical outcome. Journal of Thoracic Disease, 9(11), 4208–4211. DOI 10.21037/jtd. [Google Scholar] [CrossRef]
246. Chiu, C. H., Yang, C. T., Shih, J. Y., Huang, M. S., Su, W. C. et al. (2015). Epidermal growth factor receptor tyrosine kinase inhibitor treatment response in advanced lung adenocarcinomas with G719X/L861Q/S768I mutations. Journal of Thoracic Oncology, 10(5), 793–799. DOI 10.1097/JTO.0000000000000504. [Google Scholar] [CrossRef]
247. Wang, W., Figg, W. D. (2008). Secondary BRCA1 and BRCA2 alterations and acquired chemoresistance. Cancer Biology and Therapy, 7(7), 1004–1005. DOI 10.4161/cbt.7.7.6409. [Google Scholar] [CrossRef]
248. Khalique, S., Pettitt, S. J., Kelly, G., Tunariu, N., Natrajan, R. et al. (2020). Longitudinal analysis of a secondary BRCA2 mutation using digital droplet PCR. The Journal of Pathology: Clinical Research, 6(1), 3–11. [Google Scholar]
249. Harrison, R. G. (1907). Observations on the living developing nerve fiber. Experimental Biology and Medicine, 4, 140–143. DOI 10.3181/00379727-4-98. [Google Scholar] [CrossRef]
250. Eiraku, M., Watanabe, K., Matsuo-Takasaki, M., Kawada, M., Yonemura, S. et al. (2008). Self-organized formation of polarized cortical tissues from ESCs and its active manipulation by extrinsic signals. Cell Stem Cell, 3(5), 519–532. DOI 10.1016/j.stem.2008.09.002. [Google Scholar] [CrossRef]
251. Simian, M., Bissell, M. J. (2017). Organoids: A historical perspective of thinking in three dimensions. Journal of Cell Biology, 216(1), 31–40. DOI 10.1083/jcb.201610056. [Google Scholar] [CrossRef]
252. Wensink, G. E., Elias, S. G., Mullenders, J., Koopman, M., Boj, S. F. et al. (2021). Patient-derived organoids as a predictive biomarker for treatment response in cancer patients. npj Precision Oncology, 5(1), 1–13. [Google Scholar]
253. Cristobal, A., van den Toorn, H. W. P., van de Wetering, M., Clevers, H., Heck, A. J. R. et al. (2017). Personalized proteome profiles of healthy and tumor human colon organoids reveal both individual diversity and basic features of colorectal cancer. Cell Reports, 18(1), 263–274. DOI 10.1016/j.celrep.2016.12.016. [Google Scholar] [CrossRef]
254. Derouet, M. F., Allen, J., Wilson, G. W., Ng, C., Radulovich, N. et al. (2020). Towards personalized induction therapy for esophageal adenocarcinoma: Organoids derived from endoscopic biopsy recapitulate the pre-treatment tumor. Scientific Reports, 10(1), 14514. DOI 10.1038/s41598-020-71589-4. [Google Scholar] [CrossRef]
255. Leca, J., Martinez, S., Lac, S., Nigri, J., Secq, V. et al. (2016). Cancer-associated fibroblast-derived annexin A6+ extracellular vesicles support pancreatic cancer aggressiveness. Journal of Clinical Investigation, 126(11), 4140–4156. DOI 10.1172/JCI87734. [Google Scholar] [CrossRef]
256. Fu, Y., Liu, S., Yin, S., Niu, W., Xiong, W. et al. (2017). The reverse warburg effect is likely to be an achilles’ heel of cancer that can be exploited for cancer therapy. Oncotarget, 8(34), 57813–57825. DOI 10.18632/oncotarget.18175. [Google Scholar] [CrossRef]
257. Danhier, P., Banski, P., Payen, V. L., Grasso, D., Ippolito, L. et al. (2017). Cancer metabolism in space and time: Beyond the warburg effect. Biochimica et Biophysica Acta (BBA) Bioenergetics, 1858(8), 556–572. DOI 10.1016/j.bbabio.2017.02.001. [Google Scholar] [CrossRef]
258. Benny, S., Mishra, R., Manojkumar, M. K., Aneesh, T. P. (2020). From warburg effect to reverse warburg effect; The new horizons of anti-cancer therapy. Medical Hypotheses, 144, 110216. DOI 10.1016/j.mehy.2020.110216. [Google Scholar] [CrossRef]
259. Pach, E., Kümper, M., Fromme, J. E., Zamek, J., Metzen, F. et al. (2021). Extracellular matrix remodeling by fibroblast-MMP14 regulates melanoma growth. International Journal of Molecular Sciences, 22(22), 12276. DOI 10.3390/ijms222212276. [Google Scholar] [CrossRef]
260. Karampoga, A., Tzaferi, K., Koutsakis, C., Kyriakopoulou, K., Karamanos, N. K. (2022). Exosomes and the extracellular matrix: A dynamic interplay in cancer progression. International Journal of Developmental Biology, 66, 97–102. DOI 10.1387/ijdb.210120nk. [Google Scholar] [CrossRef]
261. Weis, S. M., Cheresh, D. A. (2011). Tumor angiogenesis: Molecular pathways and therapeutic targets. Nature Medicine, 17(11), 1359–1370. DOI 10.1038/nm.2537. [Google Scholar] [CrossRef]
262. Cao, Z., Ding, B. S., Guo, P., Lee, S. B., Butler, J. M. et al. (2014). Angiocrine factors deployed by tumor vascular niche induce B cell lymphoma invasiveness and chemoresistance. Cancer Cell, 25(3), 350–365. DOI 10.1016/j.ccr.2014.02.005. [Google Scholar] [CrossRef]
263. Krishnamurthy, S., Dong, Z., Vodopyanov, D., Imai, A., Helman, J. I. et al. (2010). Endothelial cell-initiated signaling promotes the survival and self-renewal of cancer stem cells. Cancer Research, 70(23), 9969–9978. DOI 10.1158/0008-5472.CAN-10-1712. [Google Scholar] [CrossRef]
264. Huang, Z., Zhang, M., Chen, G., Wang, W., Zhang, P. et al. (2019). Bladder cancer cells interact with vascular endothelial cells triggering EGFR signals to promote tumor progression. International Journal of Oncology, 54(5), 1555–1566. DOI 10.3892/ijo. [Google Scholar] [CrossRef]
265. Keedy, V. L., Sandler, A. B. (2007). Inhibition of angiogenesis in the treatment of non-small cell lung cancer. Cancer Science, 98(12), 1825–1830. DOI 10.1111/j.1349-7006.2007.00620.x. [Google Scholar] [CrossRef]
266. Saif, M. W., Elfiky, A., Salem, R. R. (2007). Gastrointestinal perforation due to bevacizumab in colorectal cancer. Annals of Surgical Oncology, 14(6), 1860–1869. DOI 10.1245/s10434-006-9337-9. [Google Scholar] [CrossRef]
267. Vlachogiannis, G., Hedayat, S., Vatsiou, A., Jamin, Y., Fernández-Mateos, J. et al. (2018). Patient-derived organoids model treatment response of metastatic gastrointestinal cancers. Science, 359(6378), 920–926. DOI 10.1126/science.aao2774. [Google Scholar] [CrossRef]
Cite This Article
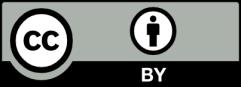
This work is licensed under a Creative Commons Attribution 4.0 International License , which permits unrestricted use, distribution, and reproduction in any medium, provided the original work is properly cited.