Open Access
ARTICLE
Racial Bias Can Confuse AI for Genomic Studies
1
Institute for Advanced Studies in Humanities and Social Science, Beihang University, Beijing, 102206, China
2
School of Law, Hubei University, Wuhan, 430062, China
3
School of Astronautics, Beihang University, Beijing, 102206, China
4
Department of Orthodontics, Peking University School of Stomatology, Beijing, 100034, China
* Corresponding Author: Xiaomo Liu. Email:
# These authors contributed equally
Oncologie 2022, 24(1), 113-130. https://doi.org/10.32604/oncologie.2022.020259
Received 13 November 2021; Accepted 21 February 2022; Issue published 31 March 2022
Abstract
Large-scale genomic studies are important ways to comprehensively decode the human genomics, and provide valuable insights to human disease causalities and phenotype developments. Genomic studies are in need of high throughput bioinformatics analyses to harness and integrate such big data. It is in this overarching context that artificial intelligence (AI) offers enormous potentials to advance genomic studies. However, racial bias is always an important issue in the data. It is usually due to the accumulation process of the dataset that inevitability involved diverse subjects with different races. How can race bias affect the outcomes of AI methods? In this work, we performed comprehensive analyses taking The Cancer Genome Atlas (TCGA) project as a case study. We construct a survival model as well as multiple artificial intelligence prediction models to analyze potential confusion caused by racial bias. From the genomic discovery, we demonstrated cancer associated genes identified from the major race hardly overlap with the discoveries from minor races from the same causal gene discovery model. We demonstrated that the biased racial distribution will greatly affect the cancer-associated genes, even taking the racial identity as a confounding factor in the model. The prediction models will be potentially risky and less accurate due to the existence of racial bias in projects. Cancer genes from the overall patient model with strong racial bias will be less informative to the minor races. Meanwhile, when the racial bias is less severe, the major conclusion from the overall analysis can be less useful even for the major group.Keywords
Cite This Article
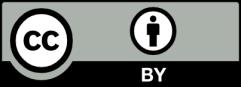