Open Access
ARTICLE
Diagnosis of Lung Cancer Based on Plasma Metabolomics Combined with Serum Markers
1 Department of Oncology, The First Affiliated Hospital of Jinzhou Medical University, Jinzhou, China
2 Ultrasound Department, Jilin Cancer Hospital, Changchun, China
3 Dalian Institute of Chemical Physics, Chinese Academy of Sciences, Dalian, China
4 Mental Health Centre, University of Alberta, Edmonton, Canada
5 Kunming Medical University, Kunming, China
# Dong Zhang and Lingxia Tong contributed equally to this article
* Corresponding Author: Zhitu Zhu. Email:
Oncologie 2020, 22(2), 75-82. https://doi.org/10.32604/oncologie.2020.012376
Abstract
Predicting the onset and metastasis of early tumor is the primary means of improving lung cancer prognosis. The purpose of this study was to identify the ability of plasma metabolomics combined with blood markers to establish benign lung disease versus lung cancer regression models. Blood samples were collected from 174 lung cancer patients, 350 benign lung disease patients and 100 healthy volunteers and the metabolites were analyzed by mass spectrometry. The target metabolites consisted of 23 amino acids, 26 acylcarnitines and 45 conventional blood markers. A regression analysis model was established based on 12 metabolites and five blood markers selected by elastic network analysis. Two-thirds of the data were used in a training set for modeling and signature construction, and the remaining one-third were used in a validation set to test the model. This model was identified to have good specificity and sensitivity in distinguishing between lung cancer and lung disease. The performance of the model was evaluated using the area under the receiver operating curve, which was 0.915 in training set and 0.875 in validation set. In conclusion, this study demonstrates that regression model established by plasma metabolomics in combination with conventional serum markers has potential for the diagnosis of lung cancer.Keywords
Cite This Article
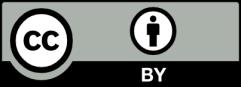