Open Access
ARTICLE
Lung Nodule Detection Based on YOLOv3 Deep Learning with Limited Datasets
1 School of Foreign Language, Guangxi University, Nanning, 530004, China
2 School of Computer, Electronics and Information, Guangxi University, Nanning, 530004, China
3 Guangxi Key Laboratory of Multimedia Communications and Network Technology, Nanning, 530004, China
4 School of Informatics, Xiamen University, Xiamen, 361005, China
5 College of Medicine, Guangxi University, Nanning, 530004, China
* Corresponding Author: Xuejun Zhang. Email:
Molecular & Cellular Biomechanics 2022, 19(1), 17-28. https://doi.org/10.32604/mcb.2022.018318
Received 16 July 2021; Accepted 01 October 2021; Issue published 12 January 2022
Abstract
The early symptom of lung tumor is always appeared as nodule on CT scans, among which 30% to 40% are malignant according to statistics studies. Therefore, early detection and classification of lung nodules are crucial to the treatment of lung cancer. With the increasing prevalence of lung cancer, large amount of CT images waiting for diagnosis are huge burdens to doctors who may missed or false detect abnormalities due to fatigue. Methods: In this study, we propose a novel lung nodule detection method based on YOLOv3 deep learning algorithm with only one preprocessing step is needed. In order to overcome the problem of less training data when starting a new study of Computer Aided Diagnosis (CAD), we firstly pick up a small number of diseased regions to simulate a limited datasets training procedure: 5 nodule patterns are selected and deformed into 110 nodules by random geometric transformation before fusing into 10 normal lung CT images using Poisson image editing. According to the experimental results, the Poisson fusion method achieves a detection rate of about 65.24% for testing 100 new images. Secondly, 419 slices from common database RIDER are used to train and test our YOLOv3 network. The time of lung nodule detection by YOLOv3 is shortened by 2–3 times compared with the mainstream algorithm, with the detection accuracy rate of 95.17%. Finally, the configuration of YOLOv3 is optimized by the learning data sets. The results show that YOLOv3 has the advantages of high speed and high accuracy in lung nodule detection, and it can access a large amount of CT image data within a short time to meet the huge demand of clinical practice. In addition, the use of Poisson image editing algorithms to generate data sets can reduce the need for raw training data and improve the training efficiency.Keywords
Cite This Article
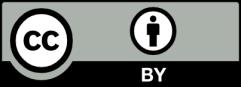