Open Access
ABSTRACT
Characterization of Coronary Atherosclerotic Plaque Composition Based on Convolutional Neural Network (CNN)
1 School of Biological Science and Medical Engineering, Southeast University, Nanjing, 210096, China.
2 Department of Cardiology, Nanjing Drum Tower Hospital, Nanjing, 210000, China.
* Corresponding Author: Zhiyong Li. Email: zylicam@gmail.com.
Molecular & Cellular Biomechanics 2019, 16(Suppl.1), 57-57. https://doi.org/10.32604/mcb.2019.05732
Abstract
The tissue composition and morphological structure of atherosclerotic plaques determine its stability or vulnerability. Intravascular optical coherence tomography (IVOCT) has rapidly become the method of choice for assessing the pathology of the coronary arterial wall in vivo due to its superior resolution. However, in clinical practice, the analysis of plaque composition of OCT images mainly relies on the interpretation of images by well-trained experts, which is a time-consuming, labor-intensive procedure and it is also subjective. The purpose of this study is to use the Convolutional neural network (CNN) method to automatically extract the best feature information from the OCT images to characterize the three basic components of atherosclerotic plaque (fibrous, lipid, and calcification). This study selected the OCT images of 20 patients from Nanjing Drum Tower Hospital from 2015.12 to 2016.12. The OCT-reading expert first excluded the images containing the brackets, and then divided all the remaining images, resulting in 1500 plaque OCT images. The expert labeled plaque composition in each image, cutting it into 11*11 image patches and obtained 87390 patches. 75000 of them were set as training examples and the others were set for testing. The classification accuracy of the test set served as the evaluation criterion. The experimental results show that the average classification accuracy of the fibrous, calcification, and lipid patches by the CNN classifier was over 75%, especially to characterize the fibrous patches, whose accuracy could reach more than 80%. The proposed method is effective and robust in the analysis of atherosclerotic plaque composition in coronary OCT images, providing a base for further segmentation study.Keywords
Cite This Article
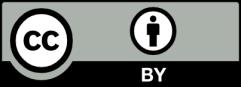