Open Access
ARTICLE
Tumor Cell Identification in Ki-67 Images on Deep Learning
Department of Biomedical Engineering, Nanjing University of Aeronautics & Astronautics, Nanjing, 211106, China.
The University of Melbourne, Parkville Campus, Melbourne, Victoria, 3010, Australia.
* Corresponding Author: Chunxiao Chen. E-mail: . Email:
Molecular & Cellular Biomechanics 2018, 15(3), 177-187. https://doi.org/10.3970/mcb.2018.04292
Abstract
The proportion of cells staining for the nuclear antigen Ki-67 is an important predictive indicator for assessment of tumor cell proliferation and growth in routine pathological investigation. Instead of traditional scoring methods based on the experience of a trained laboratory scientist, deep learning approach can be automatically used to analyze the expression of Ki-67 as well. Deep learning based on convolutional neural networks (CNN) for image classification and single shot multibox detector (SSD) for object detection are used to investigate the expression of Ki-67 for assessment of biopsies from patients with breast cancer in this study. The results focus on estimating the probability heatmap of tumor cells using CNN with accuracy of 98% and detecting the tumor cells using SSD with accuracy of 90%. This deep learning framework will provide an objective basis for the malignant degree of breast tumors and be beneficial to the pathologists for fast and efficiently Ki-67 scoring.Keywords
Cite This Article
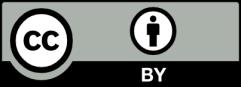
This work is licensed under a Creative Commons Attribution 4.0 International License , which permits unrestricted use, distribution, and reproduction in any medium, provided the original work is properly cited.