Open Access
ARTICLE
Research on Rainfall Estimation Based on Improved Kalman Filter Algorithm
1 Engineering Research Center of Digital Forensics, Ministry of Education, School of Computer & Software, Nanjing University of Information Science & Technology, China
2 Nanjing Xinda Institute of Meteorological Science and Technology Co., Ltd., Nanjing, 210044, Jiangsu, China
3 State Key Laboratory of Severe Weather, Chinese Academy of Meteorological Sciences, China
4 Department of Computer, Texas Tech University, Lubbock, TX 79409, USA
* Corresponding Author: Wei Fang. Email:
Journal of Quantum Computing 2022, 4(1), 23-37. https://doi.org/10.32604/jqc.2022.026975
Received 09 January 2022; Accepted 29 April 2022; Issue published 12 August 2022
Abstract
In order to solve the rainfall estimation error caused by various noise factors such as clutter, super refraction, and raindrops during the detection process of Doppler weather radar. This paper proposes to improve the rainfall estimation model of radar combined with rain gauge which calibrated by common Kalman filter. After data preprocessing, the radar data should be classified according to the precipitation intensity. And then, they are respectively substituted into the improved filter for calibration. The state noise variance and the measurement noise variance can be adaptively calculated and updated according to the input observation data during this process. Then the optimal parameter value of each type of precipitation intensity can be obtained. The state noise variance and the measurement noise variance could be assigned optimal values when filtering the remaining data. This rainfall estimation based on semi-adaptive Kalman filter calibration not only improves the accuracy of rainfall estimation, but also greatly reduces the amount of calculation. It avoids errors caused by repeated calculations, and improves the efficiency of the rainfall estimation at the same time.Keywords
Cite This Article
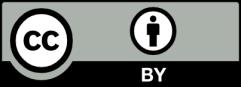
This work is licensed under a Creative Commons Attribution 4.0 International License , which permits unrestricted use, distribution, and reproduction in any medium, provided the original work is properly cited.