Open Access
ARTICLE
Implementation of Art Pictures Style Conversion with GAN
1 School of Computer Science, Southwest Petroleum University, Chengdu, 610000, China
2 AECC Sichuan Gas Turbine Establishment, Mianyang, 621700, China
* Corresponding Author: Desheng Zheng. Email:
Journal of Quantum Computing 2021, 3(4), 127-136. https://doi.org/10.32604/jqc.2021.017251
Received 20 July 2021; Accepted 21 September 2021; Issue published 10 January 2022
Abstract
Image conversion refers to converting an image from one style to another and ensuring that the content of the image remains unchanged. Using Generative Adversarial Networks (GAN) for image conversion can achieve good results. However, if there are enough samples, any image in the target domain can be mapped to the same set of inputs. On this basis, the Cycle Consistency Generative Adversarial Network (CycleGAN) was developed. This article verifies and discusses the advantages and disadvantages of the CycleGAN model in image style conversion. CycleGAN uses two generator networks and two discriminator networks. The purpose is to learn the mapping relationship and inverse mapping relationship between the source domain and the target domain. It can reduce the mapping and improve the quality of the generated image. Through the idea of loop, the loss of information in image style conversion is reduced. When evaluating the results of the experiment, the degree of retention of the input image content will be judged. Through the experimental results, CycleGAN can understand the artist’s overall artistic style and successfully convert real landscape paintings. The advantage is that most of the content of the original picture can be retained, and only the texture line of the picture is changed to a level similar to the artist’s style.Keywords
Cite This Article
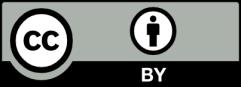
This work is licensed under a Creative Commons Attribution 4.0 International License , which permits unrestricted use, distribution, and reproduction in any medium, provided the original work is properly cited.