Open Access
ARTICLE
Improved Prediction and Understanding of Glass-Forming Ability Based on Random Forest Algorithm
1 School of Information and Software Engineering, University of Electronic Science and Technology of China, Chengdu, China
2 School of Physics, University of Electronic Science and Technology of China, Chengdu, 610054, China
3 Department of Chemistry, Physics, and Atmospheric Sciences, Jackson State University, Jackson, USA
* Corresponding Author: Xiaoyu Li. Email:
Journal of Quantum Computing 2021, 3(2), 79-87. https://doi.org/10.32604/jqc.2021.016651
Received 15 March 2021; Accepted 24 April 2021; Issue published 22 June 2021
Abstract
As an ideal material, bulk metallic glass (MG) has a wide range of applications because of its unique properties such as structural, functional and biomedical materials. However, it is difficult to predict the glass-forming ability (GFA) even given the criteria in theory and this problem greatly limits the application of bulk MG in industrial field. In this work, the proposed model uses the random forest classification method which is one of machine learning methods to solve the GFA prediction for binary metallic alloys. Compared with the previous SVM algorithm models of all features combinations, this new model is successfully constructed based on the random forest classification method with a new combination of features and it obtains better prediction results. Simultaneously, it further shows the degree of feature parameters influence on GFA. Finally, a normalized evaluation indicator of binary alloy for machine learning model performance is put forward for the first time. The result shows that the application of machine learning in MGs is valuable.Keywords
Cite This Article
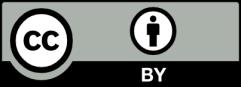
This work is licensed under a Creative Commons Attribution 4.0 International License , which permits unrestricted use, distribution, and reproduction in any medium, provided the original work is properly cited.