Open Access
ARTICLE
Malware Detection Based on Multidimensional Time Distribution Features
1 School of Cyberspace Security, Beijing University of Posts and Telecommunication, Beijing, China
2 Computer Network Emergency Response Technical Team/Coordination Center of China (CNCERT/CC), Beijing, China
3 Zhejiang Branch of National Computer Network Emergency Response Technical Team/Coordination Center of China, Hangzhou, China
4 Faculty of Engineering and Information Technology, University of Technology Sydney, Sydney, Australia
* Corresponding Author: Guosheng Xu. Email:
Journal of Quantum Computing 2021, 3(2), 55-63. https://doi.org/10.32604/jqc.2021.017365
Received 16 March 2021; Accepted 26 May 2021; Issue published 22 June 2021
Abstract
Language detection models based on system calls suffer from certain false negatives and detection blind spots. Hence, the normal behavior sequences of some malware applications for a short period can become malicious behavior within a certain time window. To detect such behaviors, we extract a multidimensional time distribution feature matrix on the basis of statistical analysis. This matrix mainly includes multidimensional time distribution features, multidimensional word pair correlation features, and multidimensional word frequency distribution features. A multidimensional time distribution model based on neural networks is built to detect the overall abnormal behavior within a given time window. Experimental evaluation is conducted using the ADFA-LD dataset. Accuracy, precision, and recall are used as the measurement indicators of the model. An accuracy rate of 95.26% and a recall rate of 96.11% are achieved.Keywords
Cite This Article
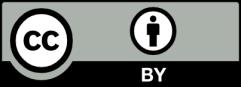
This work is licensed under a Creative Commons Attribution 4.0 International License , which permits unrestricted use, distribution, and reproduction in any medium, provided the original work is properly cited.