Open Access
ARTICLE
A Prediction Method of Trend-Type Capacity Index Based on Recurrent Neural Network
1 School of Information and Software Engineering, University of Electronic Science and Technology of China, Chengdu, China
2 SI-TECH Information Technology Company Limited, Beijing, China
3 Department of Chemistry, Physics and Atmospheric Sciences, Jackson State University, Jackson, USA
* Corresponding Authors: Wenxiao Wang. Email: ; Xiaoyu Li. Email:
Journal of Quantum Computing 2021, 3(1), 25-33. https://doi.org/10.32604/jqc.2021.016346
Received 31 December 2020; Accepted 13 May 2021; Issue published 20 May 2021
Abstract
Due to the increase in the types of business and equipment in telecommunications companies, the performance index data collected in the operation and maintenance process varies greatly. The diversity of index data makes it very difficult to perform high-precision capacity prediction. In order to improve the forecasting efficiency of related indexes, this paper designs a classification method of capacity index data, which divides the capacity index data into trend type, periodic type and irregular type. Then for the prediction of trend data, it proposes a capacity index prediction model based on Recurrent Neural Network (RNN), denoted as RNN-LSTM-LSTM. This model includes a basic RNN, two Long Short-Term Memory (LSTM) networks and two Fully Connected layers. The experimental results show that, compared with the traditional HoltWinters, Autoregressive Integrated Moving Average (ARIMA) and Back Propagation (BP) neural network prediction model, the mean square error (MSE) of the proposed RNN-LSTM-LSTM model are reduced by 11.82% and 20.34% on the order storage and data migration, which has greatly improved the efficiency of trend-type capacity index prediction.Keywords
Cite This Article
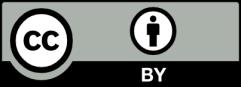
This work is licensed under a Creative Commons Attribution 4.0 International License , which permits unrestricted use, distribution, and reproduction in any medium, provided the original work is properly cited.