Open Access
ARTICLE
Hierarchical Geographically Weighted Regression Model
Journal of Quantum Computing 2019, 1(1), 9-20. https://doi.org/10.32604/jqc.2019.05954
Abstract
In spatial analysis, two problems of the scale effect and the spatial dependence have been plagued scholars, the first law of geography presented to solve the spatial dependence has played a good role in the guidelines, forming the Geographical Weighted Regression (GWR). Based on classic statistical techniques, GWR model has ascertain significance in solving spatial dependence and spatial non-uniform problems, but it has no impact on the integration of the scale effect. It does not consider the interaction between the various factors of the sampling scale observations and the numerous factors of possible scale effects, so there is a loss of information. Crossing a two-stage analysis of “return of regression” to establish the model of Hierarchical Geographically Weighted Regression (HGWR), the first layer of regression analysis reflects the spatial dependence of space samples and the second layer of the regression reflects the spatial relationships scaling. The combination of both solves the spatial scale effect analysis, spatial dependence and spatial heterogeneity of the combined effects.Keywords
Cite This Article
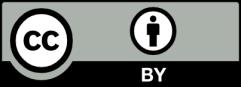
This work is licensed under a Creative Commons Attribution 4.0 International License , which permits unrestricted use, distribution, and reproduction in any medium, provided the original work is properly cited.