Open Access
ARTICLE
Optimizing Hybrid Fibre-Reinforced Polymer Bars Design: A Machine Learning Approach
1 School of Civil Engineering, Zhengzhou University, Zhengzhou, 450001, China
2 College of Civil Engineering, Tongji University, Shanghai, 200092, China
* Corresponding Author: Pu Zhang. Email:
Journal of Polymer Materials 2024, 41(1), 15-44. https://doi.org/10.32604/jpm.2024.053859
Received 11 May 2024; Accepted 06 June 2024; Issue published 21 June 2024
Abstract
Fiber-reinforced polymer (FRP) bars are gaining popularity as an alternative to steel reinforcement due to their advantages such as corrosion resistance and high strength-to-weight ratio. However, FRP has a lower modulus of elasticity compared to steel. Therefore, special attention is required in structural design to address deflection related issues and ensure ductile failure. This research explores the use of machine learning algorithms such as gene expression programming (GEP) to develop a simple and effective equation for predicting the elastic modulus of hybrid fiber-reinforced polymer (HFPR) bars. A comprehensive database of 125 experimental results of HFPR bars was used for training and validation. Statistical parameters such as R, MAE, RRSE, and RMSE are used to judge the accuracy of the developed model. Also, parametric analysis and SHAP analysis have been carried out to reveal the most influential factors in the predictive model. Finally, the proposed model was compared to the available equations for elastic modulus. The results demonstrate that the developed GEP model performance is better than that of the traditional formula. Statistical parameters and K-fold cross-validation ensured the accuracy and reliability of the predictive model. Finally, the study recommends the optimal GEP model for predicting the elastic modulus of HFRP bars and improving the structural design of HFRP.Keywords
Cite This Article
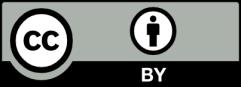
This work is licensed under a Creative Commons Attribution 4.0 International License , which permits unrestricted use, distribution, and reproduction in any medium, provided the original work is properly cited.