Open Access
ARTICLE
Fusion of Internal Similarity to Improve the Accuracy of Recommendation Algorithm
1 School of Computer and Software, Nanjing University of Information Science and Technology, Nanjing, 210044, China
2 Yukun (Beijing) Network Technology Co., Ltd., Beijing, 102200, China
3 Jiangsu Engineering Center of Network Monitoring, Nanjing University of Information Science and Technology, Nanjing,
210044, China
4 School of Computer Science and Technology, Colorado Technical University, Colorado Springs, 80907, USA
* Corresponding Author: Chengjun Zhang. Email:
Journal on Internet of Things 2021, 3(2), 65-76. https://doi.org/10.32604/jiot.2021.015401
Received 07 January 2021; Accepted 11 April 2021; Issue published 15 July 2021
Abstract
Collaborative filtering algorithms (CF) and mass diffusion (MD) algorithms have been successfully applied to recommender systems for years and can solve the problem of information overload. However, both algorithms suffer from data sparsity, and both tend to recommend popular products, which have poor diversity and are not suitable for real life. In this paper, we propose a user internal similarity-based recommendation algorithm (UISRC). UISRC first calculates the item-item similarity matrix and calculates the average similarity between items purchased by each user as the user’s internal similarity. The internal similarity of users is combined to modify the recommendation score to make score predictions and suggestions. Simulation experiments on RYM and Last.FM datasets, the results show that UISRC can obtain better recommendation accuracy and a variety of recommendations than traditional CF and MD algorithms.Keywords
Cite This Article
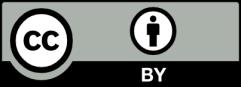